filmov
tv
MIT 6.S191: AI Bias and Fairness
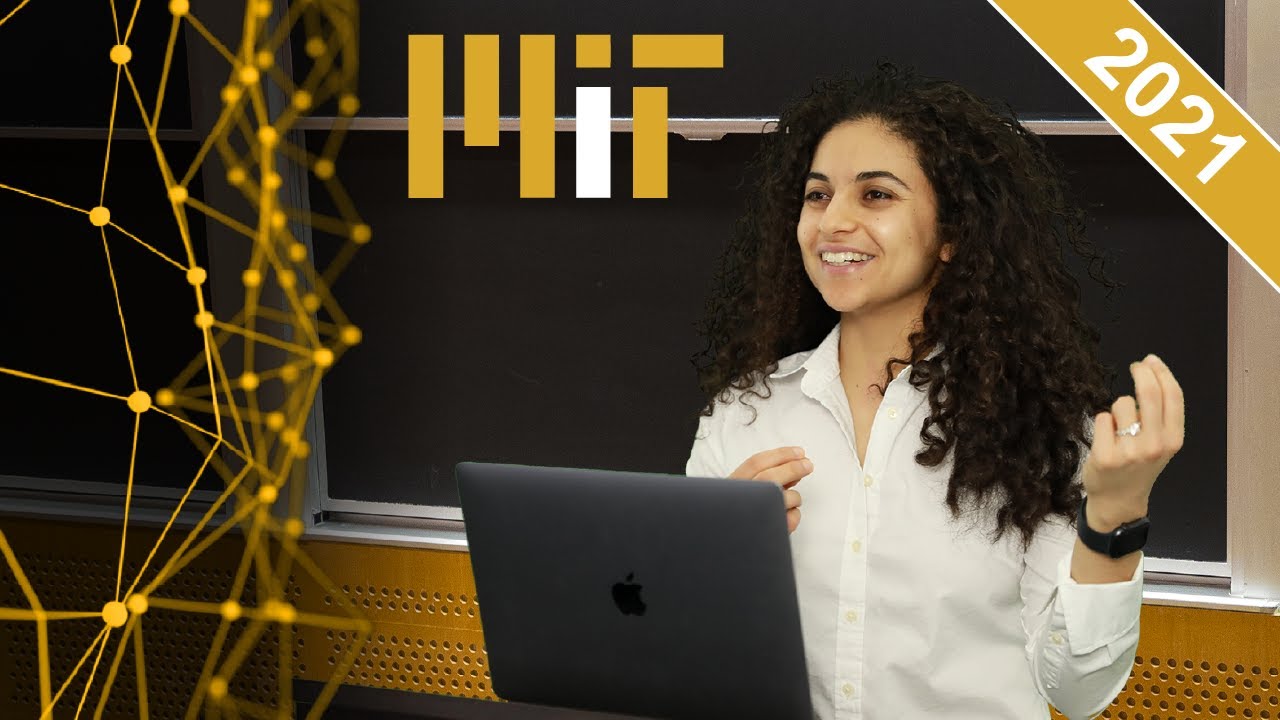
Показать описание
MIT Introduction to Deep Learning 6.S191: Lecture 8
Algorithmic Bias and Fairness
Lecturer: Ava Soleimany
January 2021
Lecture Outline
0:00 - Introduction and motivation
1:40 - What does "bias" mean?
4:22 - Bias in machine learning
8:32 - Bias at all stages in the AI life cycle
9:25 - Outline of the lecture
10:00 - Taxonomy (types) of common biases
11:29 - Interpretation driven biases
16:04 - Data driven biases - class imbalance
24:02 - Bias within the features
27:09 - Mitigate biases in the model/dataset
33:20 - Automated debiasing from learned latent structure
37:11 - Adaptive latent space debiasing
39:39 - Evaluation towards decreased racial and gender bias
41:00 - Summary and future considerations for AI fairness
Subscribe to stay up to date with new deep learning lectures at MIT, or follow us @MITDeepLearning on Twitter and Instagram to stay fully-connected!!
Algorithmic Bias and Fairness
Lecturer: Ava Soleimany
January 2021
Lecture Outline
0:00 - Introduction and motivation
1:40 - What does "bias" mean?
4:22 - Bias in machine learning
8:32 - Bias at all stages in the AI life cycle
9:25 - Outline of the lecture
10:00 - Taxonomy (types) of common biases
11:29 - Interpretation driven biases
16:04 - Data driven biases - class imbalance
24:02 - Bias within the features
27:09 - Mitigate biases in the model/dataset
33:20 - Automated debiasing from learned latent structure
37:11 - Adaptive latent space debiasing
39:39 - Evaluation towards decreased racial and gender bias
41:00 - Summary and future considerations for AI fairness
Subscribe to stay up to date with new deep learning lectures at MIT, or follow us @MITDeepLearning on Twitter and Instagram to stay fully-connected!!
MIT 6.S191: AI Bias and Fairness
MIT 6.S191 (2023): Robust and Trustworthy Deep Learning
MIT Introduction to Deep Learning | 6.S191
MIT 6.S191: Recurrent Neural Networks, Transformers, and Attention
MIT 6.S191: AI in Healthcare
MIT 6.S191 (2023): Recurrent Neural Networks, Transformers, and Attention
MIT Introduction to Deep Learning (2023) | 6.S191
MIT 6.S191 (2023): Deep Generative Modeling
MIT 6.S191 (2020): Introduction to Deep Learning
MIT 6.S191: Language Models and New Frontiers
MIT 6.S191 (2023): Deep Learning New Frontiers
MIT 6.S191: AI for Science
MIT 6.S191 (2021): Deep Learning New Frontiers
MIT Introduction to Deep Learning (2022) | 6.S191
MIT 6.S191: Taming Dataset Bias via Domain Adaptation
MIT 6.S191: Building AI Models in the Wild
MIT 6.S191 (2018): Introduction to Deep Learning
MIT 6.S191: Automatic Speech Recognition
MIT 6.S191: Convolutional Neural Networks
MIT 6.S191 (2021): Introduction to Deep Learning
MIT 6.S191: (Google) Generative AI for Media
MIT 6.S191 (2023): The Modern Era of Statistics
MIT 6.S191 (2023): Convolutional Neural Networks
Ethics of AI Bias (full video)
Комментарии