filmov
tv
MIT 6.S191 (2023): The Modern Era of Statistics
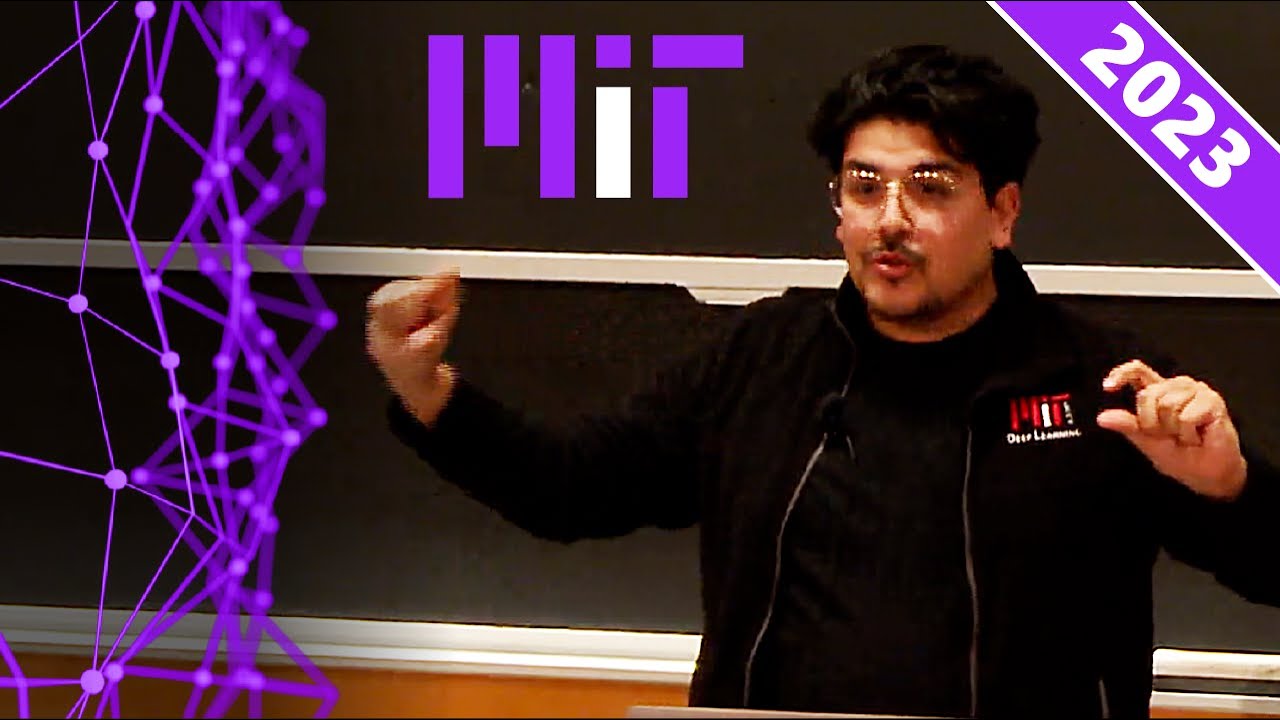
Показать описание
MIT Introduction to Deep Learning 6.S191: Lecture 9
The Modern Era of Statistics
Lecturer: Ramin Hasani
2023 Edition
Lecture Outline - coming soon!
Subscribe to stay up to date with new deep learning lectures at MIT, or follow us @MITDeepLearning on Twitter and Instagram to stay fully-connected!!
The Modern Era of Statistics
Lecturer: Ramin Hasani
2023 Edition
Lecture Outline - coming soon!
Subscribe to stay up to date with new deep learning lectures at MIT, or follow us @MITDeepLearning on Twitter and Instagram to stay fully-connected!!
MIT 6.S191 (2023): The Modern Era of Statistics
MIT 6.S191 (2023): Recurrent Neural Networks, Transformers, and Attention
MIT 6.S191 (2023): Deep Generative Modeling
MIT Introduction to Deep Learning (2023) | 6.S191
MIT 6.S191 (2023): Reinforcement Learning
MIT 6.S191 (2023): Deep Learning New Frontiers
MIT Introduction to Deep Learning | 6.S191
MIT 6.S191 (2023): Convolutional Neural Networks
MIT 6.S191 (2023): The Future of Robot Learning
MIT 6.S191 (2023): Robust and Trustworthy Deep Learning
MIT 6.S191 (2023): Text-to-Image Generation
MIT Introduction to Deep Learning (2022) | 6.S191
MIT 6.S191 (2021): Deep Learning New Frontiers
MIT 6.S191 (2021): Introduction to Deep Learning
MIT 6.S191 (2022): Recurrent Neural Networks and Transformers
MIT 6.S191 (2022): Deep Generative Modeling
MIT 6.S191: AI for Science
MIT 6.S191: Evidential Deep Learning and Uncertainty
MIT 6.S191: AI Bias and Fairness
MIT 6.S191: Automatic Speech Recognition
MIT 6.S191 (2021): Reinforcement Learning
MIT 6.S191 (2022): Deep Learning New Frontiers
MIT 6.S191 (2021): Recurrent Neural Networks
MIT 6.S191 (2022): Reinforcement Learning
Комментарии