filmov
tv
MIT 6.S191: Recurrent Neural Networks, Transformers, and Attention
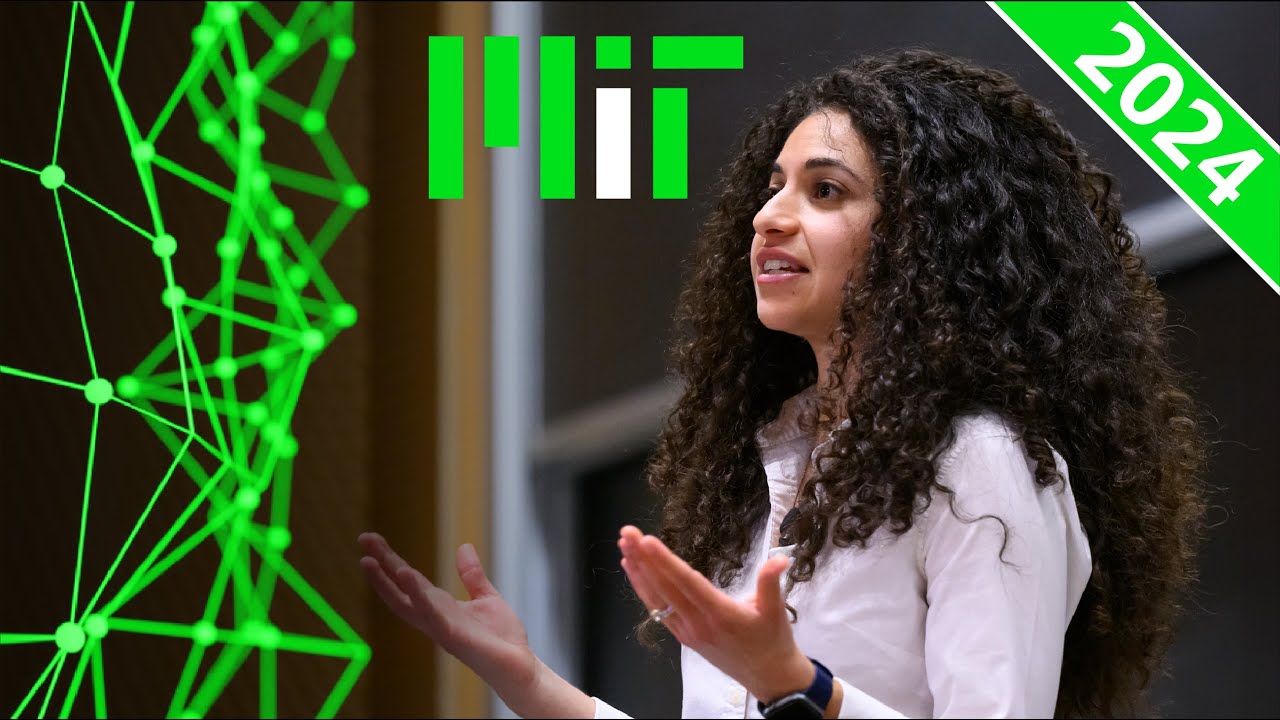
Показать описание
MIT Introduction to Deep Learning 6.S191: Lecture 2
Recurrent Neural Networks
Lecturer: Ava Amini
** New 2024 Edition **
Lecture Outline
0:00 - Introduction
3:42 - Sequence modeling
5:30 - Neurons with recurrence
12:20 - Recurrent neural networks
14:08 - RNN intuition
17:14 - Unfolding RNNs
19:54 - RNNs from scratch
22:41 - Design criteria for sequential modeling
24:24 - Word prediction example
31:50 - Backpropagation through time
33:40 - Gradient issues
37:15 - Long short term memory (LSTM)
40:00 - RNN applications
44:00- Attention fundamentals
46:46 - Intuition of attention
49:13 - Attention and search relationship
51:22 - Learning attention with neural networks
57:45 - Scaling attention and applications
1:00:08 - Summary
Subscribe to stay up to date with new deep learning lectures at MIT, or follow us @MITDeepLearning on Twitter and Instagram to stay fully-connected!!
Recurrent Neural Networks
Lecturer: Ava Amini
** New 2024 Edition **
Lecture Outline
0:00 - Introduction
3:42 - Sequence modeling
5:30 - Neurons with recurrence
12:20 - Recurrent neural networks
14:08 - RNN intuition
17:14 - Unfolding RNNs
19:54 - RNNs from scratch
22:41 - Design criteria for sequential modeling
24:24 - Word prediction example
31:50 - Backpropagation through time
33:40 - Gradient issues
37:15 - Long short term memory (LSTM)
40:00 - RNN applications
44:00- Attention fundamentals
46:46 - Intuition of attention
49:13 - Attention and search relationship
51:22 - Learning attention with neural networks
57:45 - Scaling attention and applications
1:00:08 - Summary
Subscribe to stay up to date with new deep learning lectures at MIT, or follow us @MITDeepLearning on Twitter and Instagram to stay fully-connected!!
MIT 6.S191: Recurrent Neural Networks, Transformers, and Attention
MIT 6.S191 (2023): Recurrent Neural Networks, Transformers, and Attention
MIT 6.S191 (2020): Recurrent Neural Networks
MIT 6.S191 (2021): Recurrent Neural Networks
MIT Introduction to Deep Learning | 6.S191
MIT 6.S191 (2019): Recurrent Neural Networks
MIT 6.S191: Convolutional Neural Networks
MIT 6.S191 (2022): Recurrent Neural Networks and Transformers
MIT Introduction to Deep Learning (2022) | 6.S191
Barack Obama: Intro to Deep Learning | MIT 6.S191
MIT Introduction to Deep Learning (2023) | 6.S191
MIT 6.S191 (2020): Introduction to Deep Learning
MIT 6.S094: Recurrent Neural Networks for Steering Through Time
Introduction To Recurrent Neural Network (MIT 6.S191 Lab1)
MIT 6.S191 (2018): Sequence Modeling with Neural Networks
MIT 6.S191 (2023): Convolutional Neural Networks
MIT Deep Learning 6.S191 Teaser
MIT: Machine Learning 6.036, Lecture 11: Recurrent neural networks (Fall 2020)
Deep Learning Basics: Introduction and Overview
MIT 6.S191 (2018): Deep Learning - A Personal Perspective
MIT 6.S191: (Google) Generative AI for Media
MIT 6.S191 (2021): Introduction to Deep Learning
MIT 6.S191 (2023): Deep Generative Modeling
MIT 6.S191 (2023): The Modern Era of Statistics
Комментарии