filmov
tv
MIT 6.S191 (2022): Deep Generative Modeling
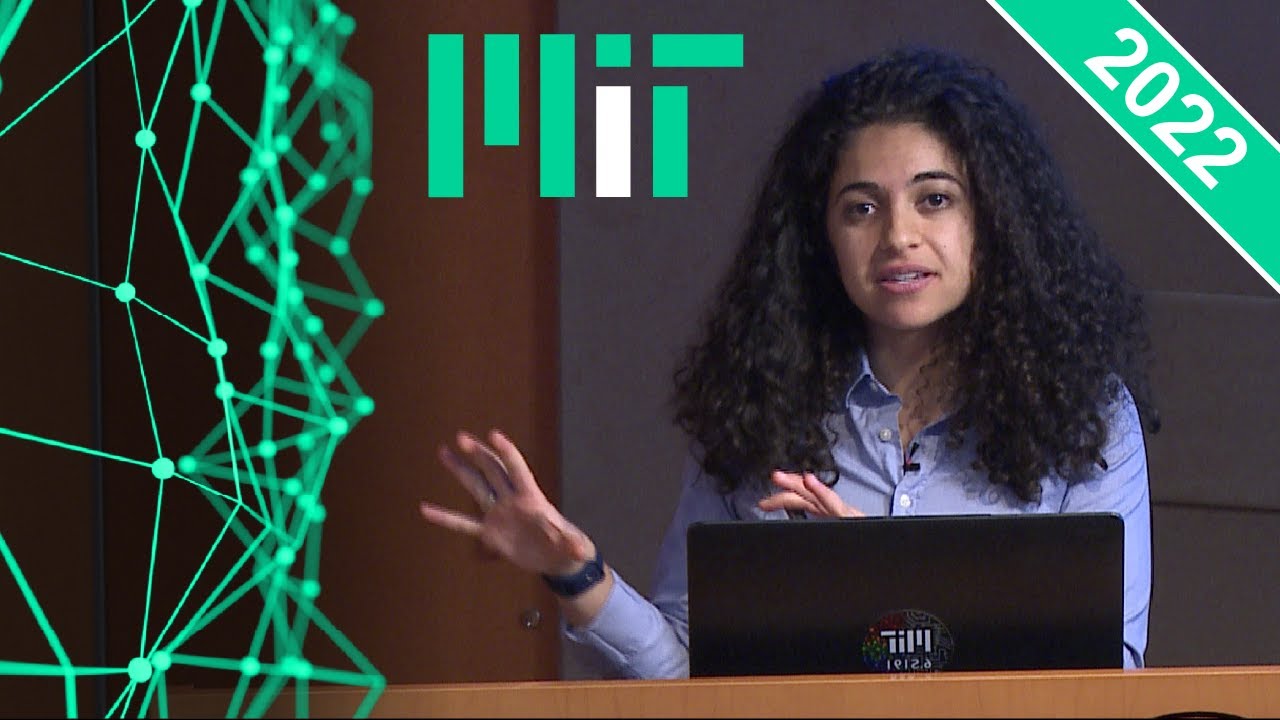
Показать описание
MIT Introduction to Deep Learning 6.S191: Lecture 4
Deep Generative Modeling
Lecturer: Ava Soleimany
January 2022
Lecture Outline - coming soon!
Subscribe to stay up to date with new deep learning lectures at MIT, or follow us @MITDeepLearning on Twitter and Instagram to stay fully-connected!!
Deep Generative Modeling
Lecturer: Ava Soleimany
January 2022
Lecture Outline - coming soon!
Subscribe to stay up to date with new deep learning lectures at MIT, or follow us @MITDeepLearning on Twitter and Instagram to stay fully-connected!!
MIT 6.S191 (2022): Deep Generative Modeling
MIT 6.S191 (2023): Deep Generative Modeling
MIT 6.S191 (2021): Deep Generative Modeling
MIT 6.S191: (Google) Generative AI for Media
MIT Introduction to Deep Learning (2023) | 6.S191
MIT 6.S191 (2022): Deep Learning New Frontiers
MIT Introduction to Deep Learning (2022) | 6.S191
MIT 6.S191 (2023): Deep Learning New Frontiers
MIT Deep Learning 6.S191 Teaser
MIT 6.S191: AI for Science
MIT 6.S191 (2023): Text-to-Image Generation
MIT 6.S191 (2022): Convolutional Neural Networks
MIT 6.S191 (2021): Deep Learning New Frontiers
MIT 6.S191: Evidential Deep Learning and Uncertainty
MIT 6.S191 (2023): Recurrent Neural Networks, Transformers, and Attention
MIT 6.S191 (2021): Introduction to Deep Learning
MIT 6.S191 (2023): The Future of Robot Learning
MIT 6.S191: Uncertainty in Deep Learning
MIT 6.S191 (2022): Recurrent Neural Networks and Transformers
Introduction to Deep Learning (MIT 6.S191)
MIT 6.S191 (2020): Deep Learning New Frontiers
MIT 6.S191 (2023): Convolutional Neural Networks
MIT 6.S191 (2023): Robust and Trustworthy Deep Learning
MIT 6.S191 (2022): Reinforcement Learning
Комментарии