filmov
tv
MIT 6.S094: Recurrent Neural Networks for Steering Through Time
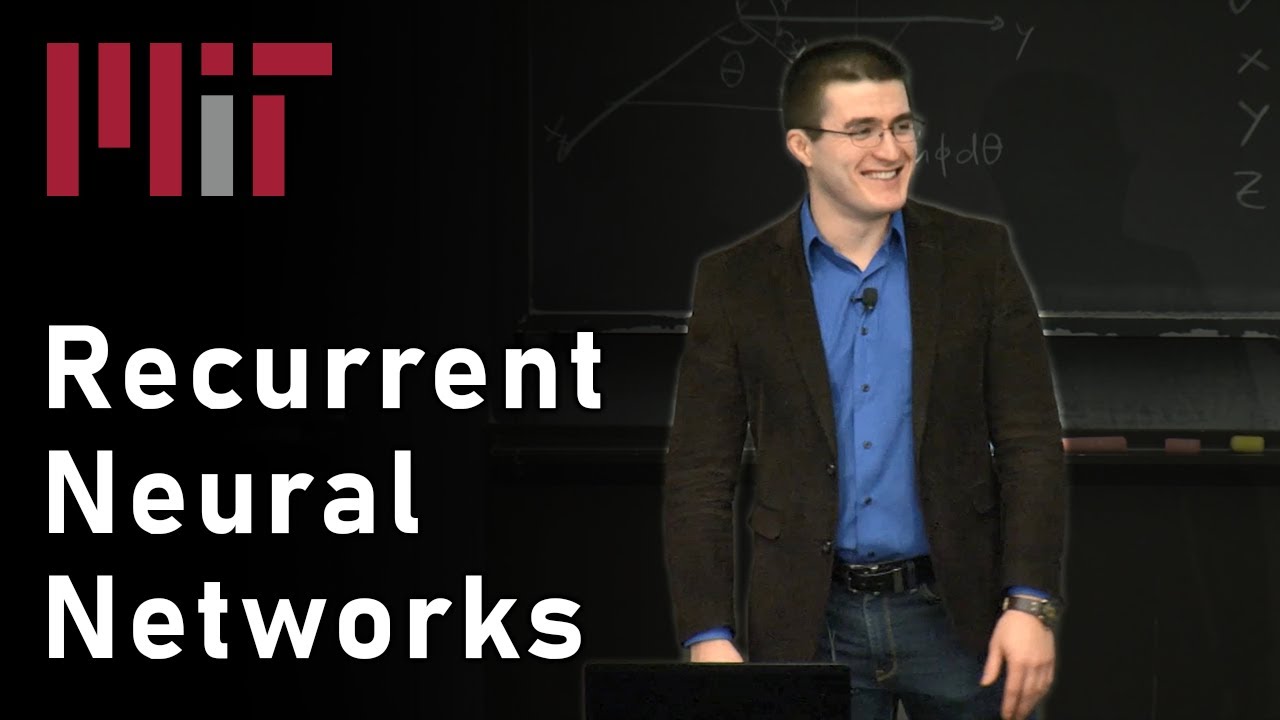
Показать описание
This is lecture 4 of course 6.S094: Deep Learning for Self-Driving Cars taught in Winter 2017.
INFO:
Links to individual lecture videos for the course:
Lecture 1: Introduction to Deep Learning and Self-Driving Cars
Lecture 2: Deep Reinforcement Learning for Motion Planning
Lecture 3: Convolutional Neural Networks for End-to-End Learning of the Driving Task
Lecture 4: Recurrent Neural Networks for Steering through Time
Lecture 5: Deep Learning for Human-Centered Semi-Autonomous Vehicles
CONNECT:
- If you enjoyed this video, please subscribe to this channel.
INFO:
Links to individual lecture videos for the course:
Lecture 1: Introduction to Deep Learning and Self-Driving Cars
Lecture 2: Deep Reinforcement Learning for Motion Planning
Lecture 3: Convolutional Neural Networks for End-to-End Learning of the Driving Task
Lecture 4: Recurrent Neural Networks for Steering through Time
Lecture 5: Deep Learning for Human-Centered Semi-Autonomous Vehicles
CONNECT:
- If you enjoyed this video, please subscribe to this channel.
MIT 6.S094: Recurrent Neural Networks for Steering Through Time
MIT 6.S191 (2020): Recurrent Neural Networks
MIT 6.S094: Convolutional Neural Networks for End-to-End Learning of the Driving Task
Recurrent Neural Networks (RNNs), Clearly Explained!!!
MIT 6.S094: Introduction to Deep Learning and Self-Driving Cars
MIT 6.S191 (2019): Recurrent Neural Networks
MIT 6.S094: Deep Reinforcement Learning for Motion Planning
MIT 6.S094: Computer Vision
MIT 6.S191 (2021): Recurrent Neural Networks
L19/1 Recurrent Neural Networks
Deep Learning Lecture 12: Introducing Recurrent Neural Networks (RNN's)
Illustrated Guide to Recurrent Neural Networks: Understanding the Intuition
Lecture 10 | Recurrent Neural Networks
What is LSTM (Long Short Term Memory)?
Ali Ghodsi, Lec [5.1]: Deep Learning, Recurrent neural network
Recurrent Neural Networks
MIT 6.S191 (2022): Recurrent Neural Networks and Transformers
Deep Learning Basics: Introduction and Overview
Recurrent Neural Networks - Ep. 9 (Deep Learning SIMPLIFIED)
MIT 6.S094: Deep Reinforcement Learning
MIT 6.S094: Deep Learning for Human-Centered Semi-Autonomous Vehicles
Real-time recurrent neural network visualization
Ali Ghodsi, Lec [5.2]: Deep Learning, Recurrent neural network
Introduction to Recurrent Neural Networks (RNNs)
Комментарии