filmov
tv
Colin Raffel: Exploring the Limits of Transfer Learning with a Unified Text-to-Text Transformer
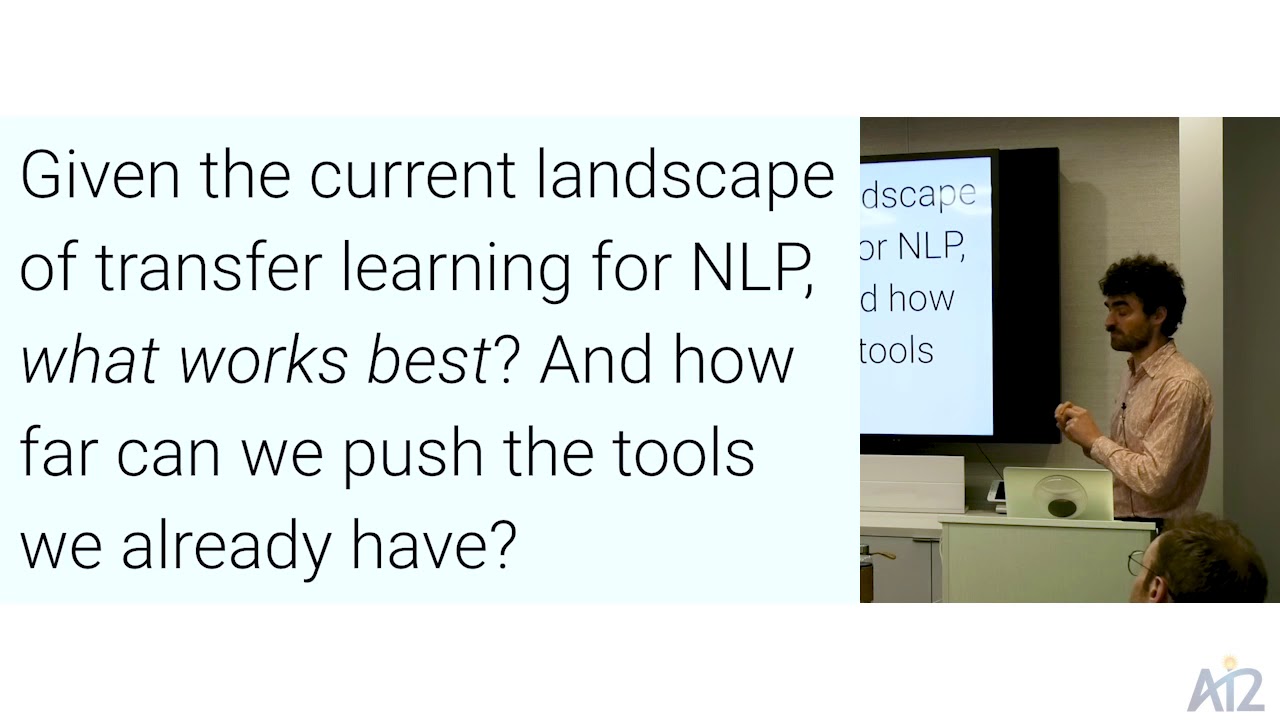
Показать описание
Transfer learning, where a model is first pre-trained on a data-rich task before being fine-tuned on a downstream task, has emerged as a powerful technique in natural language processing (NLP). The effectiveness of transfer learning has given rise to a diversity of approaches, methodology, and practice. In this talk, I will discuss our recent paper where we explored the landscape of transfer learning techniques for NLP by introducing a unified framework that converts every language problem into a text-to-text format. Our systematic study compared pre-training objectives, architectures, unlabeled datasets, transfer approaches, and other factors on dozens of language understanding tasks. By combining the insights from our exploration with scale and our new "Colossal Clean Crawled Corpus", we achieved state-of-the-art results on many benchmarks covering summarization, question answering, text classification, and more. I will wrap up by discussing some of our ongoing and future work on transfer learning for NLP.
Colin Raffel: Exploring the Limits of Transfer Learning with a Unified Text-to-Text Transformer
The Limits of NLP
Colin Raffel - Doing Strange Things with Attention - AI With The Best October 14-15, 2017
Colin Raffel | Applied Mathematics (APPM) Department Colloquium
Exploring the Limits of Transfer Learning with a Unified Text-to-Text Transformer
T5: Exploring Limits of Transfer Learning with Text-to-Text Transformer (Research Paper Walkthrough)
Exploring the Limits of Transfer Learning with a Unified Text-to-Text Transformer
C4AI Sparks: Colin Raffel's Sweet Lesson
[Audio notes] T5 - Exploring the Limits of Transfer Learning with a Unified Text-to-Text Transformer
Colin Raffel -- Explicit and Implicit Entropy Minimization in Proxy-Label-Based Semi-Supervised L...
Cohere For AI Presents: Colin Raffel
Exploring the Limits of Transfer Learning with a Unified Text-to-Text Transformer (reading papers)
Colin Raffel: A call to build models like we build open-source software
PR-216: Exploring the Limits of Transfer Learning with a Unified Text-to-Text Transformer
Talks # 3: Lorenzo Ampil - Introduction to T5 for Sentiment Span Extraction
WELM
Build an Ecosystem, Not a Monolith
T5 | Lecture 55 (Part 2) | Applied Deep Learning (Supplementary)
Realistic Evaluation of Deep Semi-Supervised Learning Algorithms (3 minute overview)
LLM: Exploring the Limits of Transfer Learning with a unified Text-to-Text Transformer (T5)
Applying the Transformer to Character-level Transduction [EACL 2021]
Thunk T5 Text To Speach
The Limits of NLU & the Rise of NLG in the Future of NLP
Efficient Large-Scale AI Workshop | Session 1: Skills acquisition and new capabilities
Комментарии