filmov
tv
How to Performance-Tune Apache Spark Applications in Large Clusters
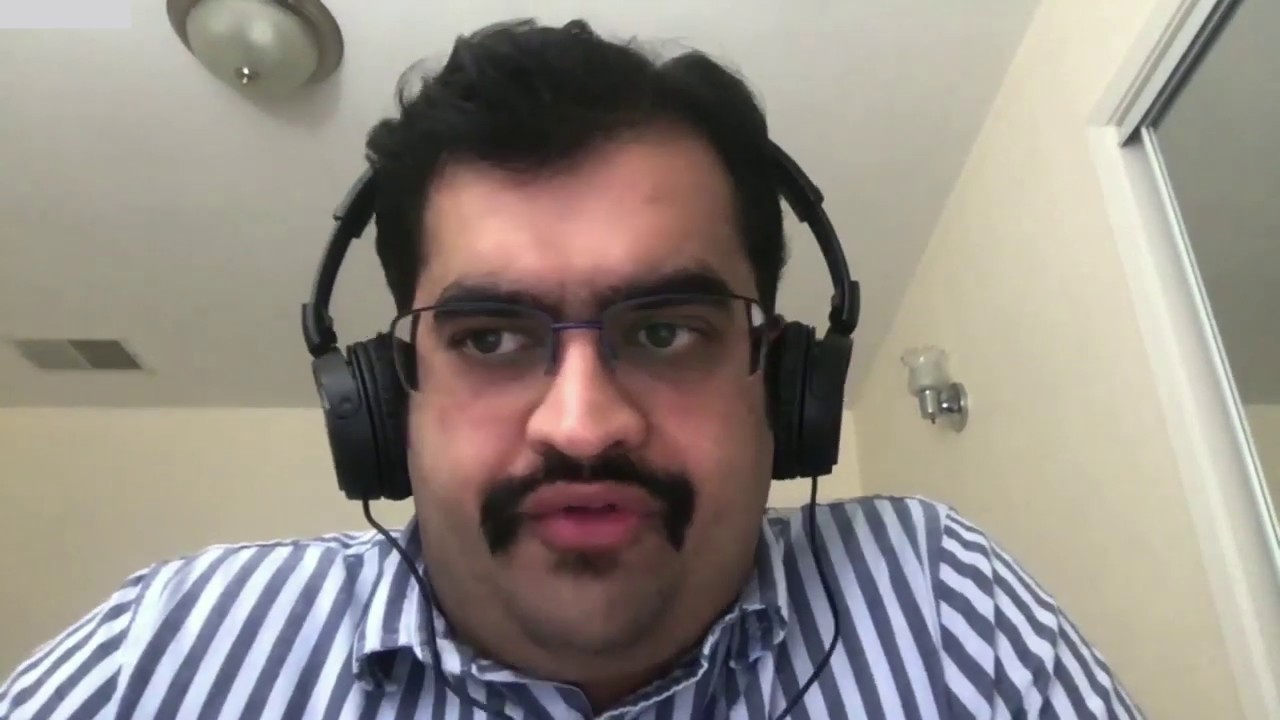
Показать описание
Omkar Joshi offers an overview on how performance challenges were addressed at Uber while rolling out its newly built flagship ingestion system, Marmaray (open-sourced) for data ingestion from various sources like Kafka, MySQL, Cassandra, and Hadoop. This system is rolled out in production and has been running for over a year now, with more ingestion systems onboarded on top of it. Omkar and team heavily used jvm-profiler during their analysis to give them valuable insights. This new system is built using the Spark framework for data ingestion. It’s designed to ingest billions of Kafka messages per topic from thousands of topics every 30 minutes. The amount of data handled by the pipeline is of the order hundreds of TBs. At this scale, every byte and millisecond saved counts. Omkar detail how to tackle such problems and insights into the optimizations already done in production.
Some key highlights are:
- how to understand your bottlenecks in Spark applications, to cache or not to cache your Spark DAG to avoid rereading your input data
- how to effectively use accumulators to avoid unnecessary Spark actions
- how to inspect your heap and non heap memory usage across hundreds of executors
- how you can change the layout of your data to save long-term storage cost
- how to effectively use serializers and compression to save network and disk traffic
- how to reduce amortized cost of your application by multiplexing your jobs.
They used different techniques for reducing memory footprint, runtime, and on-disk usage for the running applications. In terms of savings, they were able to significantly (~10% – 40%) reduce memory footprint, runtime, and disk usage.
About:
Databricks provides a unified data analytics platform, powered by Apache Spark™, that accelerates innovation by unifying data science, engineering and business.
Connect with us:
Some key highlights are:
- how to understand your bottlenecks in Spark applications, to cache or not to cache your Spark DAG to avoid rereading your input data
- how to effectively use accumulators to avoid unnecessary Spark actions
- how to inspect your heap and non heap memory usage across hundreds of executors
- how you can change the layout of your data to save long-term storage cost
- how to effectively use serializers and compression to save network and disk traffic
- how to reduce amortized cost of your application by multiplexing your jobs.
They used different techniques for reducing memory footprint, runtime, and on-disk usage for the running applications. In terms of savings, they were able to significantly (~10% – 40%) reduce memory footprint, runtime, and disk usage.
About:
Databricks provides a unified data analytics platform, powered by Apache Spark™, that accelerates innovation by unifying data science, engineering and business.
Connect with us:
Комментарии