filmov
tv
Spark Executor Core & Memory Explained
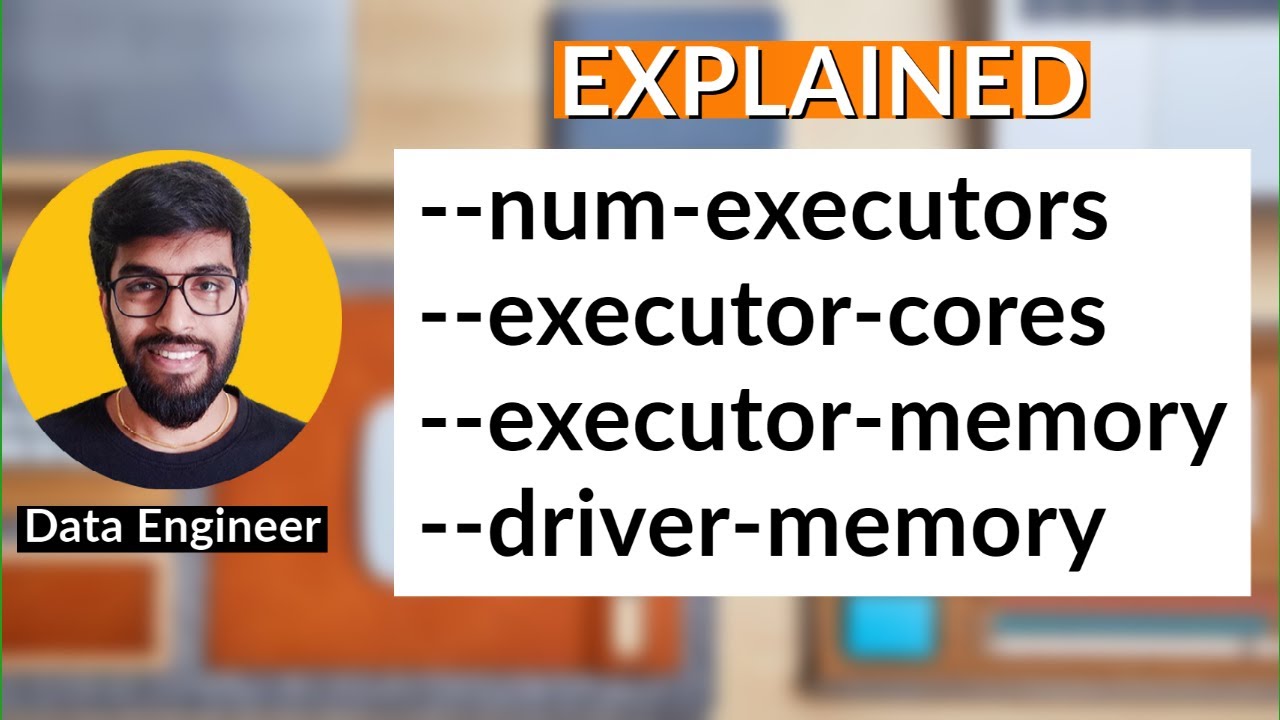
Показать описание
Spark Executor Core & Memory Explained
#apachespark #bigdata #apachespark
Video Playlist
-----------------------
YouTube channel link
Website
Technology in Tamil & English
#apachespark #bigdata #apachespark
Video Playlist
-----------------------
YouTube channel link
Website
Technology in Tamil & English
Spark Executor Core & Memory Explained
Spark Executor Memory Calculation | Number of Executors | Executor Cores | Spark Interview Q&A
Spark Interview Question | How many CPU Cores | How many executors | How much executor memory
Spark [Executor & Driver] Memory Calculation
Apache Spark Executor Tuning | Executor Cores & Memory
Apache Spark Architecture with Spark Context Driver and Executor
How Spark Executes A Program || What is Spark Driver and Executor || Spark Interview Questions
Processing 25GB of data in Spark | How many Executors and how much Memory per Executor is required.
Spark [Driver and Executor] Memory Management Deep Dive
Spark Memory Management | How to calculate the cluster Memory in Spark
Spark memory allocation and reading large files| Spark Interview Questions
executor out of memory spark | spark memory management | Lec-16
1.6 Executor vs Executor core | Spark Interview Questions
Spark Out of Memory Issue | Spark Memory Tuning | Spark Memory Management | Part 1
Learning to Determine the Number of Executors and Executor Memory | Apache Spark Job #interview
Apache Spark Memory Management | Unified Memory Management
Spark Executor Memory Calculation | Hindi | Lecture 5 | Number of Executors | Cores | Interview
Fine Tuning and Enhancing Performance of Apache Spark Jobs
Spark Basics | Partitions
Deciding the right size for executor | Spark in Production | Course on Apache Spark Core | Lesson 23
Spark Executor Tuning | Decide Number Of Executors and Memory | Spark Tutorial Interview Questions
what is VCPU? CPU - Core - Threads #compute #vcpu #bigdata #sparkcluster #databrickscluster #cluster
Spark Executor & Driver Memory Calculation | Dynamic Allocation | Interview Question
Spark Performance Tuning | EXECUTOR Tuning | Interview Question
Комментарии