filmov
tv
Direct Preference Optimization (DPO): How It Works and How It Topped an LLM Eval Leaderboard
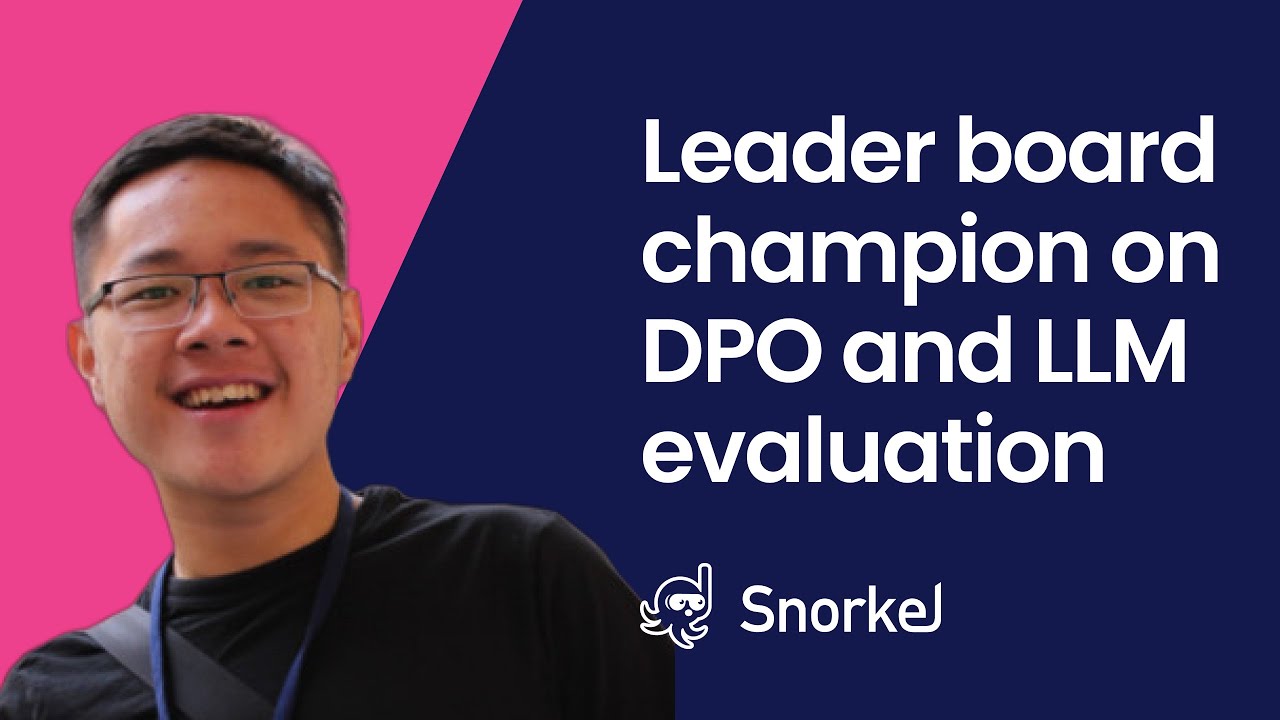
Показать описание
This interview dives into how Snorkel AI researcher Hoang Tran used direct preference optimization (DPO) to top the AlpacaEval leaderboard—and then changed how the leaderboard evaluated large language models (LLMs).
DPO is a cutting-edge approach for aligning LLMs with user preferences, and may replace reinforcement learning with human feedback (RLHF), which researchers have proven to be both more unstable than DPO and more computationally expensive.
Here's what you'll learn:
* DPO vs. RLHF: Understand the key differences between these two LLM alignment techniques.
* The Future of LLM Evaluation: Explore how Tran pushed for a change in the evaluation metric.
* How DPO can help enterprises build better LLMs.
Perfect for:
* Machine Learning Engineers
* NLP Researchers
* Anyone interested in the future of AI
#DPO #LLMevaluation #AlpacaEvalLeaderboard #SnorkelAI
DPO is a cutting-edge approach for aligning LLMs with user preferences, and may replace reinforcement learning with human feedback (RLHF), which researchers have proven to be both more unstable than DPO and more computationally expensive.
Here's what you'll learn:
* DPO vs. RLHF: Understand the key differences between these two LLM alignment techniques.
* The Future of LLM Evaluation: Explore how Tran pushed for a change in the evaluation metric.
* How DPO can help enterprises build better LLMs.
Perfect for:
* Machine Learning Engineers
* NLP Researchers
* Anyone interested in the future of AI
#DPO #LLMevaluation #AlpacaEvalLeaderboard #SnorkelAI