filmov
tv
Direct Preference Optimization: Forget RLHF (PPO)
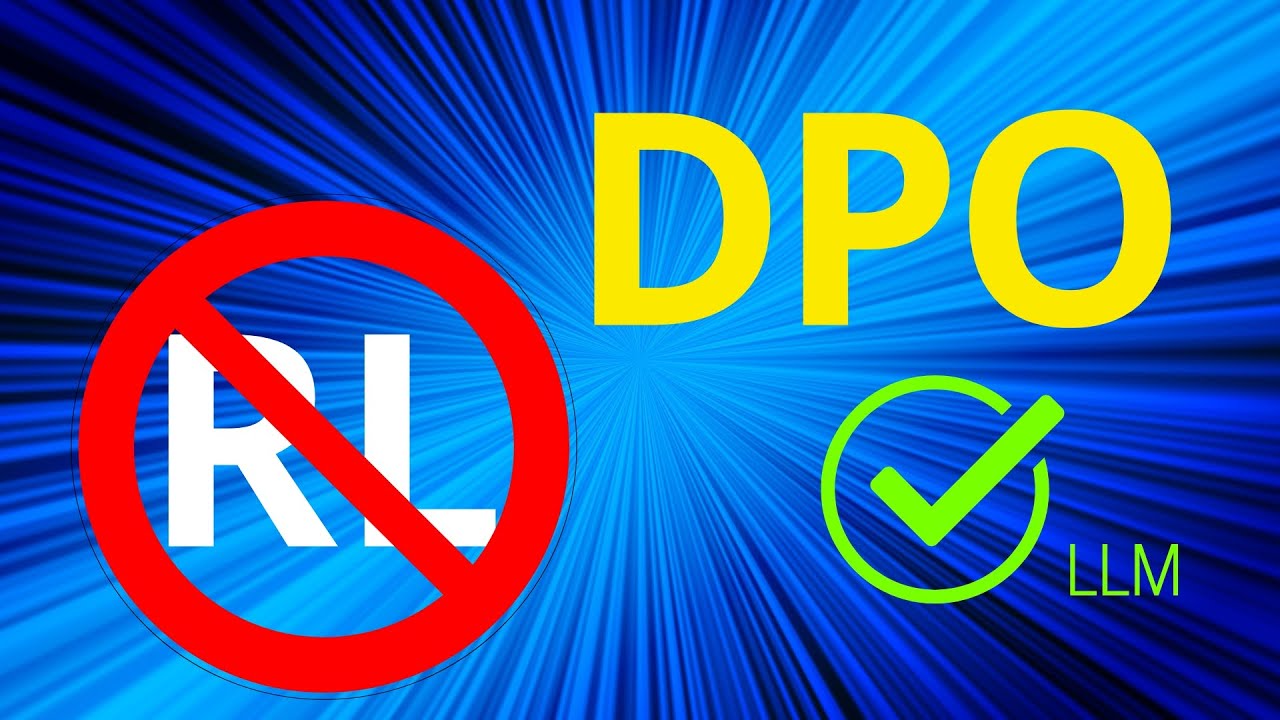
Показать описание
DPO replaces RLHF: In this technical and informative video, we explore a groundbreaking methodology called direct preference optimization (DPO) by Stanford Univ that has the potential to replace reinforcement learning in the training of GPT systems.
Join us as we dive into the intricacies of direct preference optimization, dissecting its technical details and highlighting its advantages over the conventional reinforcement learning approach.
Discover how this innovative technique opens new possibilities in AI training, offering more precise control and improved performance.
Direct Preference Optimization - DPO can fine-tune Language Models (LLMs) to align with human preferences as well as or better than existing methods. Notably, fine-tuning with DPO exceeds RLHF’s ability (Reinforcement Learning from Human Feedback) to control sentiment of generations and improves response quality in summarization and single-turn dialogue while being substantially simpler to implement and train.
All rights with authors of:
"Direct Preference Optimization: Your Language Model is Secretly a Reward Model"
Rafael Rafailov, Archit Sharma, Eric Mitchell, Stefano Ermon, Christopher D. Manning, Chelsea Finn (Stanford Univ)
Join us as we dive into the intricacies of direct preference optimization, dissecting its technical details and highlighting its advantages over the conventional reinforcement learning approach.
Discover how this innovative technique opens new possibilities in AI training, offering more precise control and improved performance.
Direct Preference Optimization - DPO can fine-tune Language Models (LLMs) to align with human preferences as well as or better than existing methods. Notably, fine-tuning with DPO exceeds RLHF’s ability (Reinforcement Learning from Human Feedback) to control sentiment of generations and improves response quality in summarization and single-turn dialogue while being substantially simpler to implement and train.
All rights with authors of:
"Direct Preference Optimization: Your Language Model is Secretly a Reward Model"
Rafael Rafailov, Archit Sharma, Eric Mitchell, Stefano Ermon, Christopher D. Manning, Chelsea Finn (Stanford Univ)
Direct Preference Optimization: Forget RLHF (PPO)
Direct Preference Optimization: Your Language Model is Secretly a Reward Model | DPO paper explained
Reinforcement Learning from Human Feedback (RLHF) & Direct Preference Optimization (DPO) Explain...
Direct Preference Optimization (DPO) explained: Bradley-Terry model, log probabilities, math
Direct Preference Optimization (DPO) - How to fine-tune LLMs directly without reinforcement learning
Direct Preference Optimization (DPO): Your Language Model is Secretly a Reward Model Explained
Direct Preference Optimization (DPO)
Towards Reliable Use of Large Language Models: Better Detection, Consistency, and Instruction-Tuning
DPO - Part1 - Direct Preference Optimization Paper Explanation | DPO an alternative to RLHF??
Direct Preference Optimization
PR-453: Direct Preference Optimization
Direct Preference Optimization (DPO)
How to Code RLHF on LLama2 w/ LoRA, 4-bit, TRL, DPO
How DPO Works and Why It's Better Than RLHF
DPO - Part2 - Direct Preference Optimization Implementation using TRL | DPO an alternative to RLHF??
The DPO debate: Do we need RL for RLHF?
4 Ways to Align LLMs: RLHF, DPO, KTO, and ORPO
CS 285: Eric Mitchell: Reinforcement Learning from Human Feedback: Algorithms & Applications
If you work in ML — You get it. RLHF is just so dang hard to remember the acronym! XD
Direct Preference Optimization Your Language Model is Secretly a Reward Model Stanford 2023
791: Reinforcement Learning from Human Feedback (RLHF) — with Dr. Nathan Lambert
Direct Preference Optimization: Your Language Model is Secretly a Reward Model (Ko/En Subtitles)
RLHF, PPO and DPO for Large language models
Proximal Policy Optimization Explained
Комментарии