filmov
tv
Direct Preference Optimization (DPO): Your Language Model is Secretly a Reward Model Explained
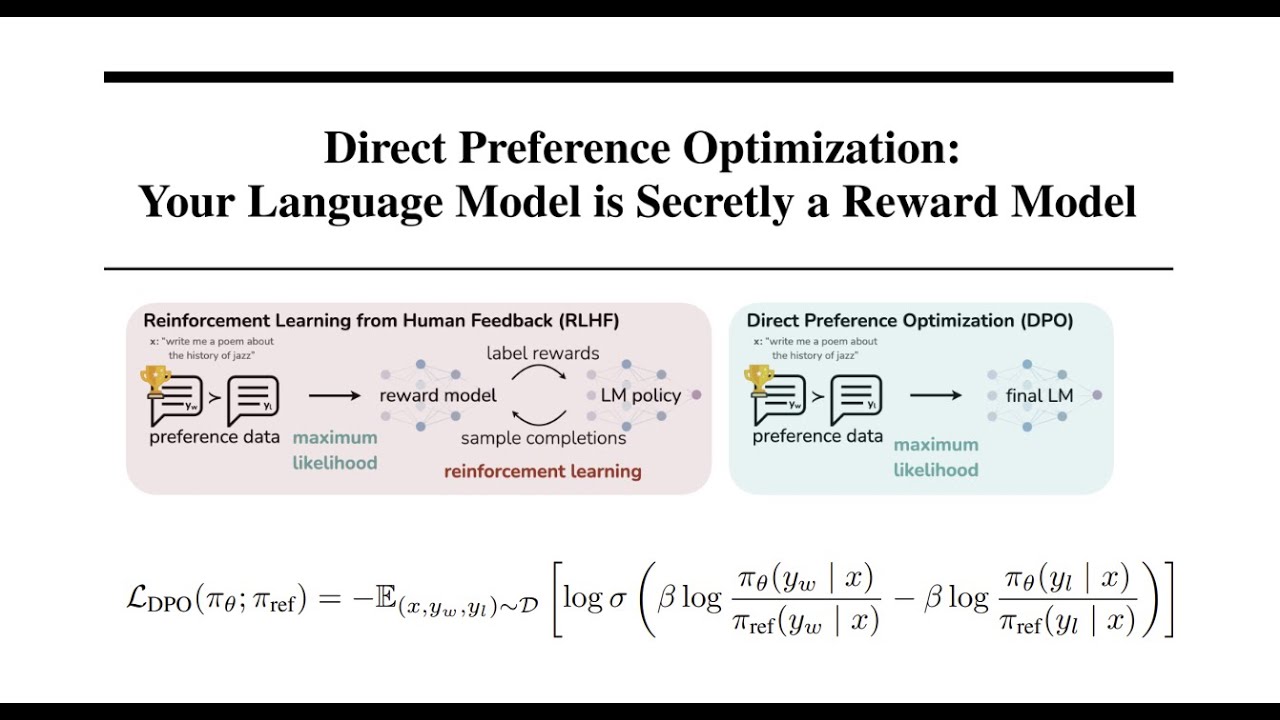
Показать описание
Direct Preference Optimization: Your Language Model is Secretly a Reward Model | DPO paper explained
Direct Preference Optimization (DPO) explained: Bradley-Terry model, log probabilities, math
Direct Preference Optimization (DPO) - How to fine-tune LLMs directly without reinforcement learning
Direct Preference Optimization (DPO): Your Language Model is Secretly a Reward Model Explained
Direct Preference Optimization: Forget RLHF (PPO)
Reinforcement Learning from Human Feedback (RLHF) & Direct Preference Optimization (DPO) Explain...
Aligning LLMs with Direct Preference Optimization
Direct Preference Optimization (DPO)
LLM Alignment: Techniques for Building Human-Aligned AI
Direct Preference Optimization (DPO) in AI
Direct Preference Optimization in One Minute
DPO Debate: Is RL needed for RLHF?
Direct Preference Optimization
Direct Preference Optimization (DPO)
Towards Reliable Use of Large Language Models: Better Detection, Consistency, and Instruction-Tuning
What is Direct Preference Optimization?
Direct Preference Optimization (DPO): How It Works and How It Topped an LLM Eval Leaderboard
DPO : Direct Preference Optimization
Direct Preference Optimization (DPO) of LLMs to Reduce Toxicity
DPO - Part1 - Direct Preference Optimization Paper Explanation | DPO an alternative to RLHF??
DPO - Part2 - Direct Preference Optimization Implementation using TRL | DPO an alternative to RLHF??
Direct Preference Optimization Your Language Model is Secretly a Reward Model
LLM training process with Direct Preference Optimization (DPO) and bypass Reward Model (Part3)
Direct Preference Optimization: Your Language Model is Secretly a Reward Model (Ko/En Subtitles)
Комментарии