filmov
tv
Direct Preference Optimization (DPO): A low cost alternative to train LLM models
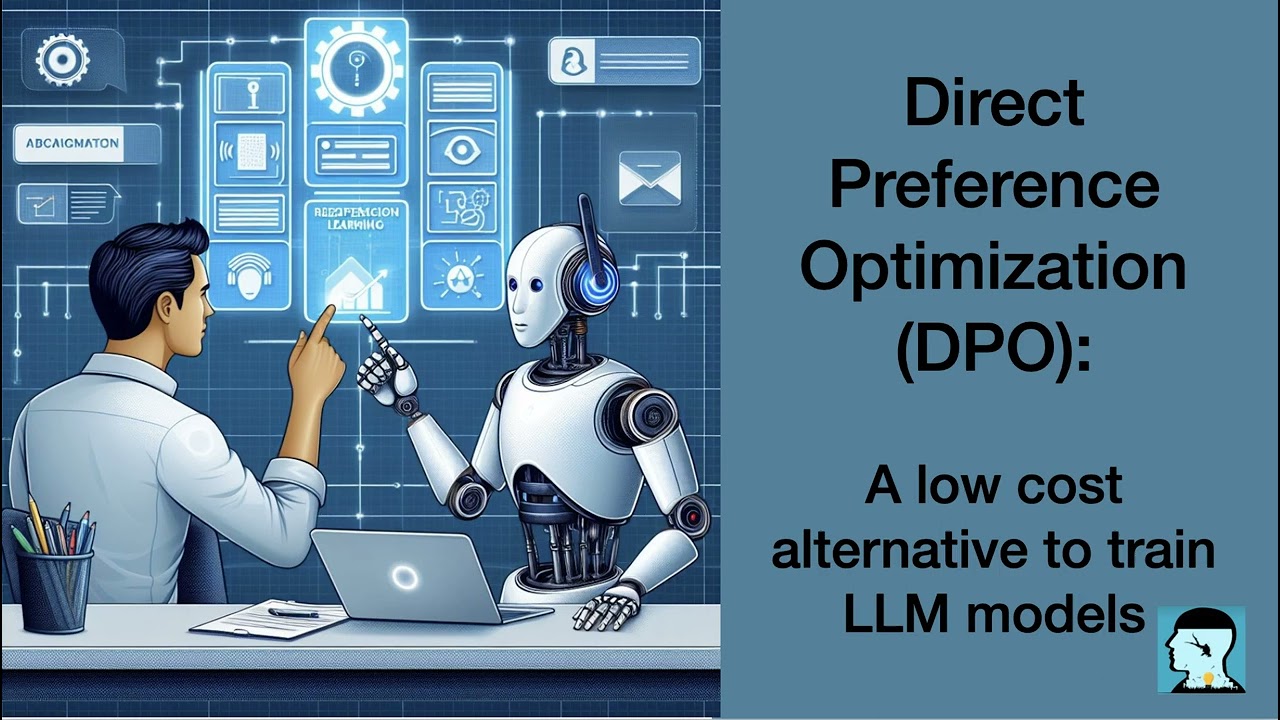
Показать описание
Building the best Large Language Models (LLMs) like ChatGPT is expensive and inaccessible for most researchers. Reinforcement Learning from Human Feedback (RLHF), a method used to optimize models, is costly and requires extensive resources. However, Direct Preference Optimization (DPO) is a mathematical breakthrough that aligns the trained model with human preferences without the need for a reinforcement learning loop. DPO uses algebra to define the reward and train the LLM directly, eliminating the need for a separate reward model. This allows for a more efficient and cost-effective way to optimize language models.