filmov
tv
Efficient Large-Scale Language Model Training on GPU Clusters
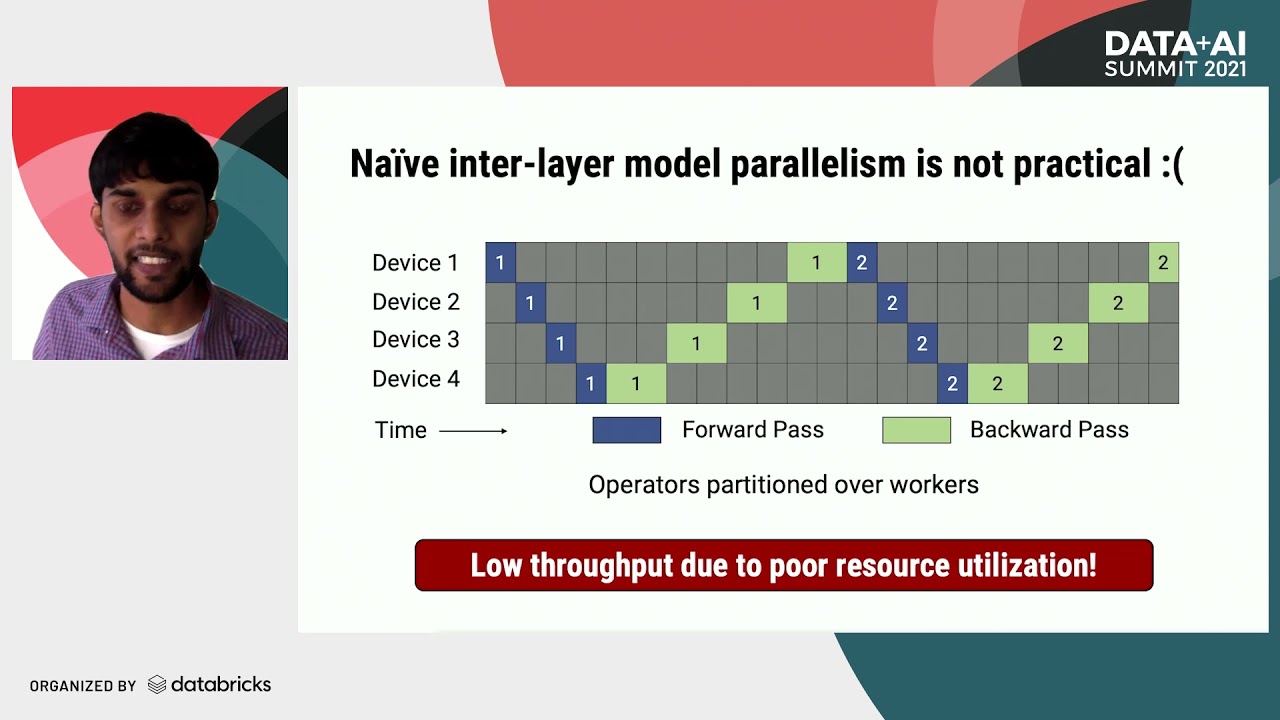
Показать описание
Large language models have led to state-of-the-art accuracies across a range of tasks. However, training these large models efficiently is challenging for two reasons: a) GPU memory capacity is limited, making it impossible to fit large models on a single GPU or even on a multi-GPU server; and b) the number of compute operations required to train these models can result in unrealistically long training times. New methods of model parallelism such as tensor and pipeline parallelism have been proposed to address these challenges; unfortunately, naive usage leads to fundamental scaling issues at thousands of GPUs due to various reasons, e.g., expensive cross-node communication or idle periods waiting on other devices.
Connect with us:
Connect with us:
Efficient Large-Scale Language Model Training on GPU Clusters
Efficient Large-Scale Language Model Training on GPU Clusters Using Megatron-LM | Jared Casper
Efficient Large Scale Language Modeling with Mixtures of Experts
Training LLMs at Scale - Deepak Narayanan | Stanford MLSys #83
RAS: Efficient Large-Scale Language Model Training on GPU Clusters Using Megatron-LM - G. Perrotta
Ultimate Guide To Scaling ML Models - Megatron-LM | ZeRO | DeepSpeed | Mixed Precision
Efficient Large Language Model training with LoRA and Hugging Face PEFT
Efficient Large-Scale AI Workshop | Session 2: Training and inference efficiency
AWS re:Invent 2024 - Scaling generative AI models for millions of users in Roblox (GAM310)
Sebastian Borgeaud - Efficient Training of Large Language Models @ UCL DARK
How are LLMs Trained? Distributed Training in AI (at NVIDIA)
Exploiting Parallelism in Large Scale DL Model Training: From Chips to Systems to Algorithms
Efficient Large-Scale AI Workshop | Session 1: Skills acquisition and new capabilities
How to Build an LLM from Scratch | An Overview
Efficient Large-Scale AI Workshop | Session 3: Aligning models with human intent
Efficient Fine-Tuning for Llama 3 Language Models
Megatron-LM: Mastering Multi-Billion Parameter Language Models
MegaScale: Scaling Large Language Model Training to More Than 10,000 GPUs | Haibin Lin
AI can't cross this line and we don't know why.
Scaling AI Model Training and Inferencing Efficiently with PyTorch
Miguel Martínez & Meriem Bendris - Building Large-scale Localized Language Models
LLM in a flash: Efficient Large Language Model Inference with Limited Memory
Unlocking Efficient Training for LLMs: The Power of Productivity per Watt - Elon Musk
Exploiting Parallelism in Large Scale Deep Learning Model Training: Chips to Systems to Algorithms
Комментарии