filmov
tv
Sebastian Borgeaud - Efficient Training of Large Language Models @ UCL DARK
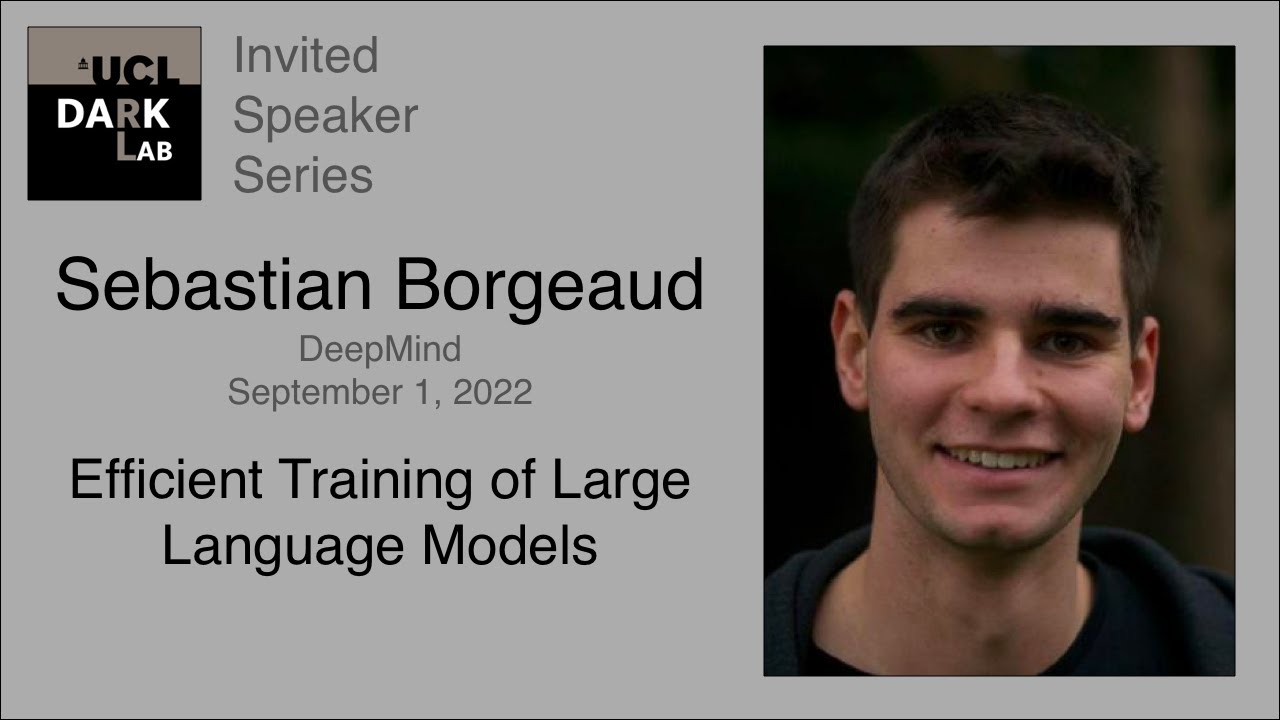
Показать описание
(there is a lag in sound until 2:15)
Invited talk by Sebastian Borgeaud on September 1, 2022 at UCL DARK.
Abstract: Large language models have become ubiquitous in many areas of deep learning research, however little is known today about how to train these models efficiently. In this talk, I’ll cover some recent advances in large language model pre-training, focusing on how these advancements decrease the compute requirement of training these large models. In particular, I’ll focus on our work revisiting the “neural scaling laws” which showed that previous large language models were too large for their compute budget, and our work on RETRO, where we enhance auto-regressive large language models with retrieval over a large database of text.
Bio: Sebastian Borgeaud is a Research Engineer at DeepMind where he co-leads the large scale language modeling team. Sebastian’s research focuses on large language models (Gopher, Chinchilla, Perceiver, Flamingo and RETRO) and more generally large scale deep learning. Before joining DeepMind in 2018, Sebastian completed his undergraduate and master's degrees at the University of Cambridge, with a focus on theoretical computer science and NLP.
Invited talk by Sebastian Borgeaud on September 1, 2022 at UCL DARK.
Abstract: Large language models have become ubiquitous in many areas of deep learning research, however little is known today about how to train these models efficiently. In this talk, I’ll cover some recent advances in large language model pre-training, focusing on how these advancements decrease the compute requirement of training these large models. In particular, I’ll focus on our work revisiting the “neural scaling laws” which showed that previous large language models were too large for their compute budget, and our work on RETRO, where we enhance auto-regressive large language models with retrieval over a large database of text.
Bio: Sebastian Borgeaud is a Research Engineer at DeepMind where he co-leads the large scale language modeling team. Sebastian’s research focuses on large language models (Gopher, Chinchilla, Perceiver, Flamingo and RETRO) and more generally large scale deep learning. Before joining DeepMind in 2018, Sebastian completed his undergraduate and master's degrees at the University of Cambridge, with a focus on theoretical computer science and NLP.