filmov
tv
Exploiting Parallelism in Large Scale Deep Learning Model Training: Chips to Systems to Algorithms
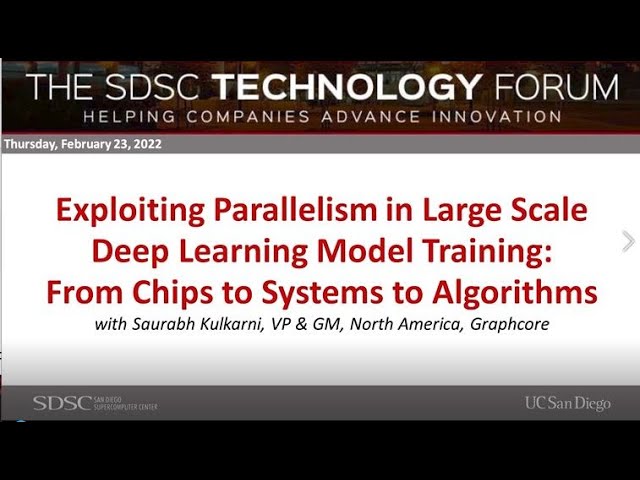
Показать описание
SDSC Industry Partners Program (IPP) Technology Forum
Presenter:
Saurabh Kulkarni is VP & GM for North America at Graphcore
Over the last 20 years, he has held various leadership positions at Intel, Microsoft, and Oracle prior to his current role at Graphcore. His roles have spanned a variety of domains, including computer architecture, server platform architecture, cloud infrastructure, and hardware accelerators for AI/ML.
Summary:
We live in a world where hyperscale systems for machine intelligence are increasingly being used to solve complex problems ranging from natural language processing to computer vision to molecular modeling, drug discovery and recommendation systems. A convergence of breakthrough research in machine learning models and algorithms, increased accessibility to hardware systems at cloud scale for research and thriving software ecosystems are paving the way for an exponential increase in model sizes. Effective parallel processing and model decomposition techniques and large clusters of accelerators will be required to train these models of the future economically.
Graphcore aims to address scale challenges associated with training large models. Get to know our Intelligent Processing Unit (IPU) – a purpose-built hardware accelerator with a unique MIMD architecture – designed to address the most demanding compute and memory bandwidth needs of modern ML models. Our network disaggregated architecture uniquely positions us to build highly scalable systems (IPU-PODs) with thousands of accelerators aimed at exploiting various dimensions of parallelism.
Presenter:
Saurabh Kulkarni is VP & GM for North America at Graphcore
Over the last 20 years, he has held various leadership positions at Intel, Microsoft, and Oracle prior to his current role at Graphcore. His roles have spanned a variety of domains, including computer architecture, server platform architecture, cloud infrastructure, and hardware accelerators for AI/ML.
Summary:
We live in a world where hyperscale systems for machine intelligence are increasingly being used to solve complex problems ranging from natural language processing to computer vision to molecular modeling, drug discovery and recommendation systems. A convergence of breakthrough research in machine learning models and algorithms, increased accessibility to hardware systems at cloud scale for research and thriving software ecosystems are paving the way for an exponential increase in model sizes. Effective parallel processing and model decomposition techniques and large clusters of accelerators will be required to train these models of the future economically.
Graphcore aims to address scale challenges associated with training large models. Get to know our Intelligent Processing Unit (IPU) – a purpose-built hardware accelerator with a unique MIMD architecture – designed to address the most demanding compute and memory bandwidth needs of modern ML models. Our network disaggregated architecture uniquely positions us to build highly scalable systems (IPU-PODs) with thousands of accelerators aimed at exploiting various dimensions of parallelism.