filmov
tv
OpenAI CLIP: ConnectingText and Images (Paper Explained)
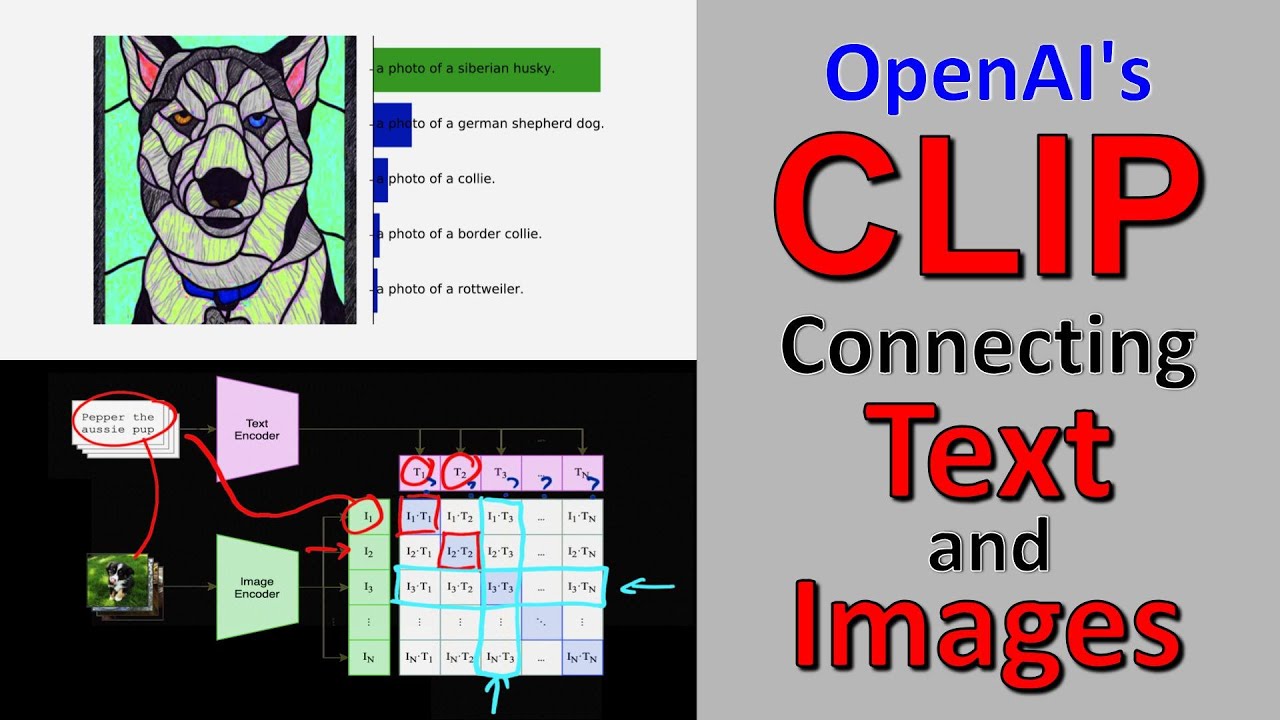
Показать описание
#ai #openai #technology
Paper Title: Learning Transferable Visual Models From Natural Language Supervision
CLIP trains on 400 million images scraped from the web, along with text descriptions to learn a model that can connect the two modalities. The core idea is a contrastive objective combined with a large batch size. The resulting model can be turned into arbitrary zero-shot classifiers for new image & text tasks.
OUTLINE:
0:00 - Introduction
3:15 - Overview
4:40 - Connecting Images & Text
9:00 - Building Zero-Shot Classifiers
14:40 - CLIP Contrastive Training Objective
22:25 - Encoder Choices
25:00 - Zero-Shot CLIP vs Linear ResNet-50
31:50 - Zero-Shot vs Few-Shot
35:35 - Scaling Properties
36:35 - Comparison on different tasks
37:40 - Robustness to Data Shift
44:20 - Broader Impact Section
47:00 - Conclusion & Comments
Abstract:
State-of-the-art computer vision systems are trained to predict a fixed set of predetermined object categories. This restricted form of supervision limits their generality and usability since additional labeled data is needed to specify any other visual concept. Learning directly from raw text about images is a promising alternative which leverages a much broader source of supervision. We demonstrate that the simple pre-training task of predicting which caption goes with which image is an efficient and scalable way to learn SOTA image representations from scratch on a dataset of 400 million (image, text) pairs collected from the internet. After pre-training, natural language is used to reference learned visual concepts (or describe new ones) enabling zero-shot transfer of the model to downstream tasks. We study the performance of this approach by benchmarking on over 30 different existing computer vision datasets, spanning tasks such as OCR, action recognition in videos, geo-localization, and many types of fine-grained object classification. The model transfers non-trivially to most tasks and is often competitive with a fully supervised baseline without the need for any dataset specific training. For instance, we match the accuracy of the original ResNet-50 on ImageNet zero-shot without needing to use any of the 1.28 million training examples it was trained on.
Authors: Alec Radford, Jong Wook Kim, Chris Hallacy, Aditya Ramesh, Gabriel Goh, Sandhini Agarwal, Girish Sastry, Amanda Askell, Pamela Mishkin, Jack Clark, Gretchen Krueger, Ilya Sutskever
Links:
If you want to support me, the best thing to do is to share out the content :)
If you want to support me financially (completely optional and voluntary, but a lot of people have asked for this):
Bitcoin (BTC): bc1q49lsw3q325tr58ygf8sudx2dqfguclvngvy2cq
Ethereum (ETH): 0x7ad3513E3B8f66799f507Aa7874b1B0eBC7F85e2
Litecoin (LTC): LQW2TRyKYetVC8WjFkhpPhtpbDM4Vw7r9m
Monero (XMR): 4ACL8AGrEo5hAir8A9CeVrW8pEauWvnp1WnSDZxW7tziCDLhZAGsgzhRQABDnFy8yuM9fWJDviJPHKRjV4FWt19CJZN9D4n
Paper Title: Learning Transferable Visual Models From Natural Language Supervision
CLIP trains on 400 million images scraped from the web, along with text descriptions to learn a model that can connect the two modalities. The core idea is a contrastive objective combined with a large batch size. The resulting model can be turned into arbitrary zero-shot classifiers for new image & text tasks.
OUTLINE:
0:00 - Introduction
3:15 - Overview
4:40 - Connecting Images & Text
9:00 - Building Zero-Shot Classifiers
14:40 - CLIP Contrastive Training Objective
22:25 - Encoder Choices
25:00 - Zero-Shot CLIP vs Linear ResNet-50
31:50 - Zero-Shot vs Few-Shot
35:35 - Scaling Properties
36:35 - Comparison on different tasks
37:40 - Robustness to Data Shift
44:20 - Broader Impact Section
47:00 - Conclusion & Comments
Abstract:
State-of-the-art computer vision systems are trained to predict a fixed set of predetermined object categories. This restricted form of supervision limits their generality and usability since additional labeled data is needed to specify any other visual concept. Learning directly from raw text about images is a promising alternative which leverages a much broader source of supervision. We demonstrate that the simple pre-training task of predicting which caption goes with which image is an efficient and scalable way to learn SOTA image representations from scratch on a dataset of 400 million (image, text) pairs collected from the internet. After pre-training, natural language is used to reference learned visual concepts (or describe new ones) enabling zero-shot transfer of the model to downstream tasks. We study the performance of this approach by benchmarking on over 30 different existing computer vision datasets, spanning tasks such as OCR, action recognition in videos, geo-localization, and many types of fine-grained object classification. The model transfers non-trivially to most tasks and is often competitive with a fully supervised baseline without the need for any dataset specific training. For instance, we match the accuracy of the original ResNet-50 on ImageNet zero-shot without needing to use any of the 1.28 million training examples it was trained on.
Authors: Alec Radford, Jong Wook Kim, Chris Hallacy, Aditya Ramesh, Gabriel Goh, Sandhini Agarwal, Girish Sastry, Amanda Askell, Pamela Mishkin, Jack Clark, Gretchen Krueger, Ilya Sutskever
Links:
If you want to support me, the best thing to do is to share out the content :)
If you want to support me financially (completely optional and voluntary, but a lot of people have asked for this):
Bitcoin (BTC): bc1q49lsw3q325tr58ygf8sudx2dqfguclvngvy2cq
Ethereum (ETH): 0x7ad3513E3B8f66799f507Aa7874b1B0eBC7F85e2
Litecoin (LTC): LQW2TRyKYetVC8WjFkhpPhtpbDM4Vw7r9m
Monero (XMR): 4ACL8AGrEo5hAir8A9CeVrW8pEauWvnp1WnSDZxW7tziCDLhZAGsgzhRQABDnFy8yuM9fWJDviJPHKRjV4FWt19CJZN9D4n
Комментарии