filmov
tv
Principal Component Analysis (PCA)
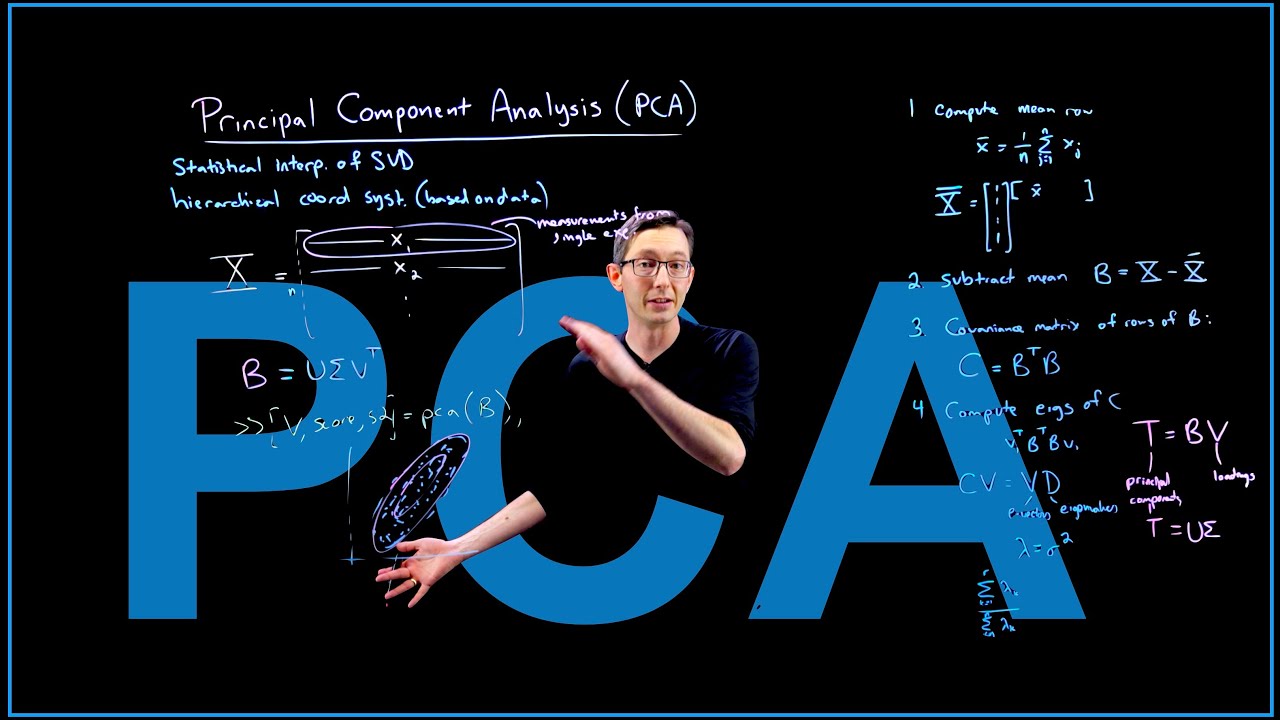
Показать описание
Principal component analysis (PCA) is a workhorse algorithm in statistics, where dominant correlation patterns are extracted from high-dimensional data.
These lectures follow Chapter 1 from: "Data-Driven Science and Engineering: Machine Learning, Dynamical Systems, and Control" by Brunton and Kutz
This video was produced at the University of Washington
These lectures follow Chapter 1 from: "Data-Driven Science and Engineering: Machine Learning, Dynamical Systems, and Control" by Brunton and Kutz
This video was produced at the University of Washington
StatQuest: Principal Component Analysis (PCA), Step-by-Step
Principal Component Analysis (PCA)
StatQuest: PCA main ideas in only 5 minutes!!!
Principal Component Analysis (PCA)
Principal Component Analysis (PCA) Explained: Simplify Complex Data for Machine Learning
Data Analysis 6: Principal Component Analysis (PCA) - Computerphile
Principal Component Analysis (PCA)
Principal Component Analysis (PCA) - easy and practical explanation
A Tale Of Two Reservoirs: How Machine Learning Can Help Define “Sweet Spots” In Reservoirs
Basics of PCA (Principal Component Analysis) : Data Science Concepts
PCA : the basics - explained super simple
Machine Learning Tutorial Python - 19: Principal Component Analysis (PCA) with Python Code
Principal Component Analysis Explained
Principal Component Analysis (PCA): With Practical Example in Minitab
19. Principal Component Analysis
PCA : the math - step-by-step with a simple example
Visualizing Principal Component Analysis (PCA)
Principal Component Analysis (PCA) - THE MATH YOU SHOULD KNOW!
17: Principal Components Analysis_ - Intro to Neural Computation
Principal Component Analysis (The Math) : Data Science Concepts
Principal component analysis step by step | PCA explained step by step | PCA in statistics
PCA In Machine Learning | Principal Component Analysis | Machine Learning Tutorial | Simplilearn
Principal Component Analysis (PCA) | Can't get simpler!
1 Principal Component Analysis | PCA | Dimensionality Reduction in Machine Learning by Mahesh Huddar
Комментарии