filmov
tv
Principal Component Analysis (PCA) - THE MATH YOU SHOULD KNOW!
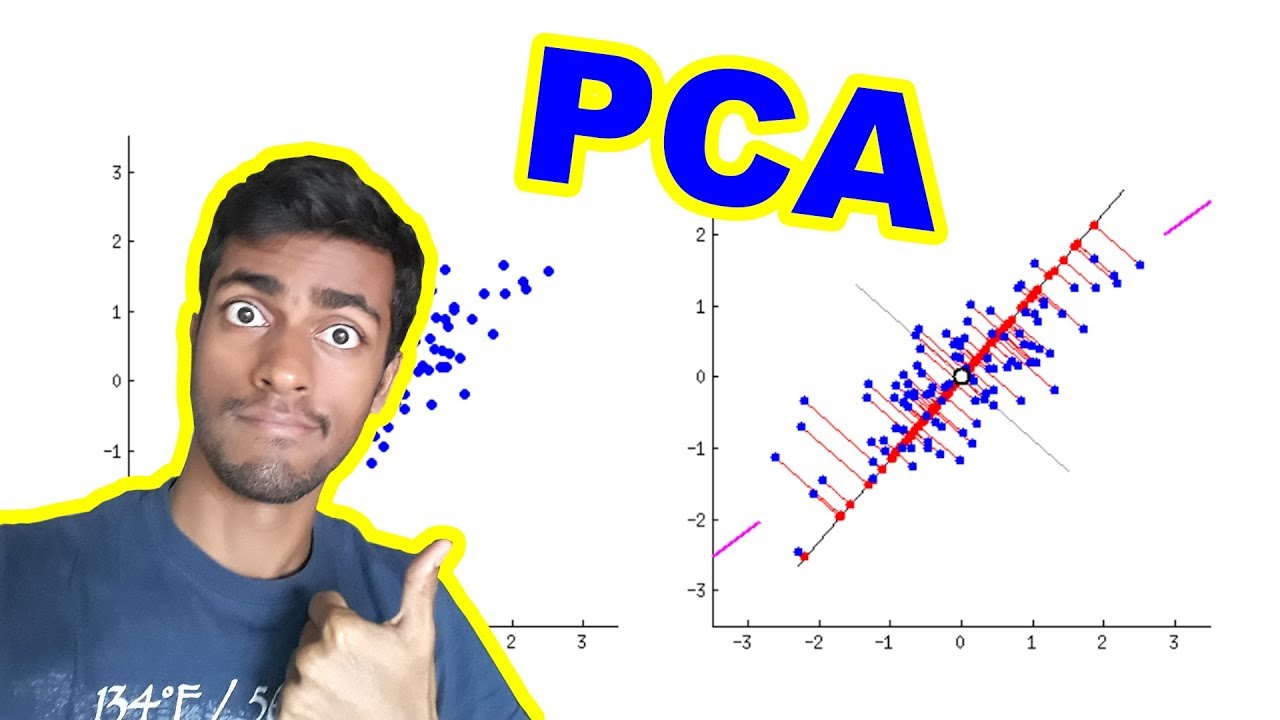
Показать описание
In this video, we are going to see exactly how we can perform dimensionality reduction with a famous Feature Extraction technique - Principal Component Analysis PCA. We’ll get into the math that powers it
REFERENCES
IMAGE REFERENCES
REFERENCES
IMAGE REFERENCES
StatQuest: Principal Component Analysis (PCA), Step-by-Step
Principal Component Analysis (PCA)
StatQuest: PCA main ideas in only 5 minutes!!!
Principal Component Analysis (PCA)
Principal Component Analysis (PCA) Explained: Simplify Complex Data for Machine Learning
Data Analysis 6: Principal Component Analysis (PCA) - Computerphile
Principal Component Analysis (PCA)
Principal Component Analysis (PCA) - easy and practical explanation
L6. Indices Exercise | FREE CMA FOUNDATION CLASSES | CA Pranav Chandak | #freecma #pca
Basics of PCA (Principal Component Analysis) : Data Science Concepts
PCA : the basics - explained super simple
Machine Learning Tutorial Python - 19: Principal Component Analysis (PCA) with Python Code
Principal Component Analysis Explained
Principal Component Analysis (PCA): With Practical Example in Minitab
Visualizing Principal Component Analysis (PCA)
19. Principal Component Analysis
PCA : the math - step-by-step with a simple example
Principal Component Analysis (PCA) - THE MATH YOU SHOULD KNOW!
17: Principal Components Analysis_ - Intro to Neural Computation
Principal Component Analysis (The Math) : Data Science Concepts
Principal component analysis step by step | PCA explained step by step | PCA in statistics
PCA In Machine Learning | Principal Component Analysis | Machine Learning Tutorial | Simplilearn
Principal Component Analysis (PCA) | Can't get simpler!
1 Principal Component Analysis | PCA | Dimensionality Reduction in Machine Learning by Mahesh Huddar
Комментарии