filmov
tv
StatQuest: Principal Component Analysis (PCA), Step-by-Step
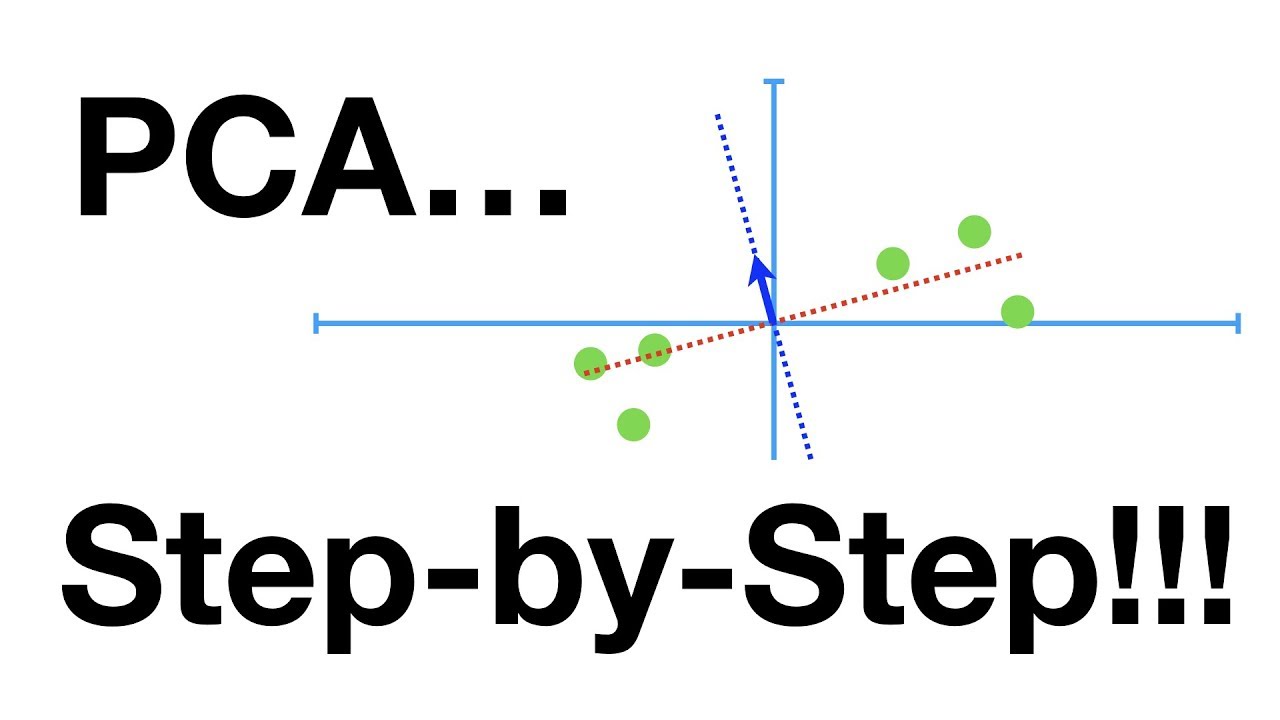
Показать описание
Principal Component Analysis, is one of the most useful data analysis and machine learning methods out there. It can be used to identify patterns in highly complex datasets and it can tell you what variables in your data are the most important. Lastly, it can tell you how accurate your new understanding of the data actually is.
In this video, I go one step at a time through PCA, and the method used to solve it, Singular Value Decomposition. I take it nice and slowly so that the simplicity of the method is revealed and clearly explained.
For a complete index of all the StatQuest videos, check out:
If you'd like to support StatQuest, please consider...
Buying The StatQuest Illustrated Guide to Machine Learning!!!
...or...
...a cool StatQuest t-shirt or sweatshirt:
...buying one or two of my songs (or go large and get a whole album!)
...or just donating to StatQuest!
Lastly, if you want to keep up with me as I research and create new StatQuests, follow me on twitter:
0:00 Awesome song and introduction
0:30 Conceptual motivation for PCA
3:23 PCA worked out for 2-Dimensional data
5:03 Finding PC1
12:08 Singular vector/value, Eigenvector/value and loading scores defined
12:56 Finding PC2
14:14 Drawing the PCA graph
15:03 Calculating percent variation for each PC and scree plot
16:30 PCA worked out for 3-Dimensional data
#statquest #PCA #ML
In this video, I go one step at a time through PCA, and the method used to solve it, Singular Value Decomposition. I take it nice and slowly so that the simplicity of the method is revealed and clearly explained.
For a complete index of all the StatQuest videos, check out:
If you'd like to support StatQuest, please consider...
Buying The StatQuest Illustrated Guide to Machine Learning!!!
...or...
...a cool StatQuest t-shirt or sweatshirt:
...buying one or two of my songs (or go large and get a whole album!)
...or just donating to StatQuest!
Lastly, if you want to keep up with me as I research and create new StatQuests, follow me on twitter:
0:00 Awesome song and introduction
0:30 Conceptual motivation for PCA
3:23 PCA worked out for 2-Dimensional data
5:03 Finding PC1
12:08 Singular vector/value, Eigenvector/value and loading scores defined
12:56 Finding PC2
14:14 Drawing the PCA graph
15:03 Calculating percent variation for each PC and scree plot
16:30 PCA worked out for 3-Dimensional data
#statquest #PCA #ML
Комментарии