filmov
tv
Quantifying Illumina Next-Gen Sequencing Libraries using digital PCR on the QuantStudio 3D
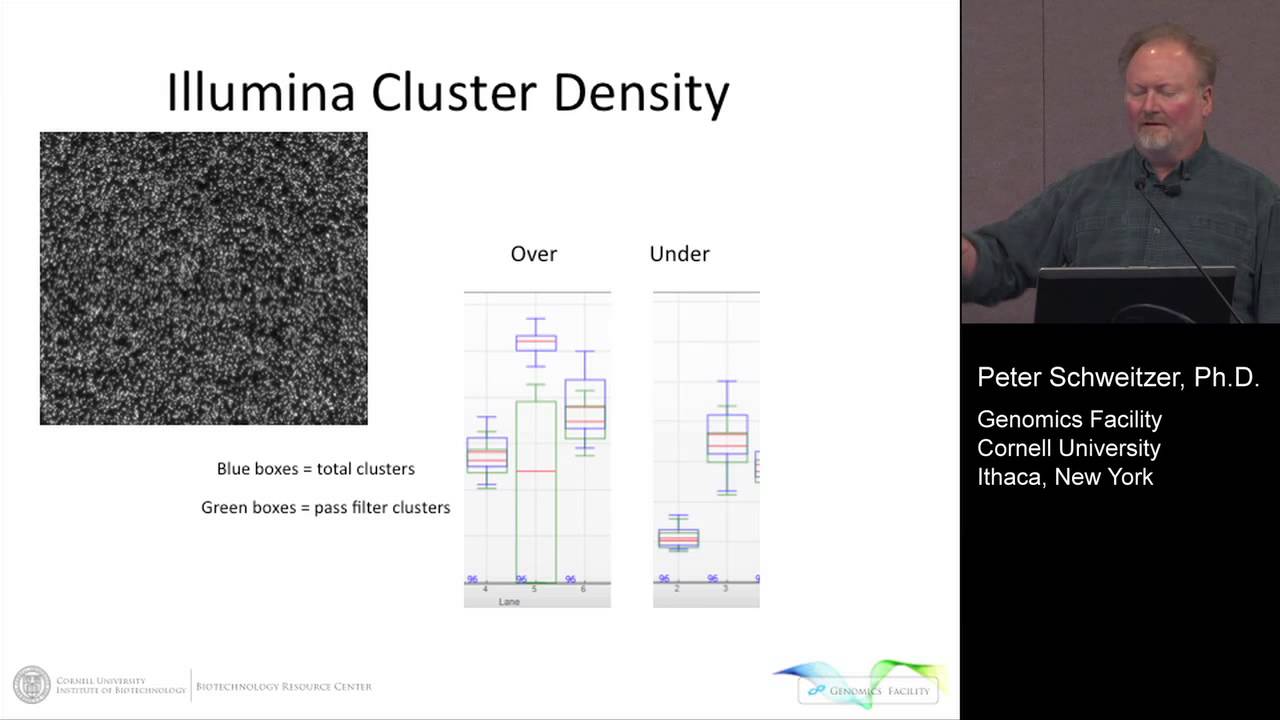
Показать описание
Digital PCR is a quantitative PCR method that works by diluting a sample into many individual PCR reactions and counting the number of partitions in which a reaction occurs. This sample partitioning enables the sensitive, specific detection of single template molecules and precise quantification. With its enhanced sensitivity and dynamic range, as well absolute quantification, digital PCR is increasingly being used to perform quality control experiments on next generation sequencing libraries. At ABRF 2014, Dr Peter Schweitzer from Cornell University Genomics Facility discusses how he has utilized digital PCR to increase sequencing accuracy, reduce run repeats, and validate sequencing results with absolute quantification.
Transcript:
It's a pleasure to come here and talk about our experiences with the QuantStudio 3D, digital PCR platform. This is a technology that's been available for a while and it's essentially a limiting dilution assay, which has been done for many, many years. But now these instruments are becoming more accessible to core facilities and individual laboratories because the cost for the instruments are dropping. The last two that have been released, the instrumentation that you had to do this on was relatively expensive, but these are quite affordable. The QuantStudio™ is quite affordable and a low risk investment for the lab.
That's one reason we brought it in initially. The chip itself sets up to 20,000 individual reactions from a single master mix. And this is the type of data that you produce. Absolute quantitation, gives a count of the number of molecules in your volume and actually then just calculates back to what your original volume was. You can also do relative quantitation or quantification and I'll argue that you should always try to do this.
It's a fabulous way to do it because then you don't really have to worry about the DNA concentration going in as long as you're within the range of the instrument. The type of images that the software produces can be manually edited, especially the dot plots. You can drag that left and right if you want to change the threshold for the software to do the different calculations. If you don't like the threshold that the software automatically puts in, although I will say, we typically don't fiddle with this except I'll show one case at the end where we did, the numbers from the instrument, the software are almost always spot on.
The software does the Poisson calculation to correct for the numbers of doubles, triples, because we're looking at individual wells in this 20,000 reaction chip and some of them are doubles and triples.
We've been doing some copy number variation studies up in Ithaca and this is actually a small part of a 17 panel DNA set looking at a single copy gene or ended up being a single copy gene but all of them, we're looking at this was the relative quantitation, so we're expressing Taqman assay for FAM versus the Taqman® assay for VIC, which is FAM is on the Y axis, VIC is on the X axis and we just look at the ratios. We don't really care about the number of spots on that chip, it's really the ratios of those two different reporters and out of 17, this is only six of them I'm showing but out of 17 they looked almost identical, the variation among the 17 was about six percent, all these ratios. So it's really terrific for that.
What I want to get to our experiences with quantifying next generation sequencing libraries. This is the problem that we're trying to address. Those of you who have Illumina instruments, you know this problem. It's the clustering issue. So the upper left is an image of what happens on the surface of the flow cell. We generate millions of clusters derived from an original DNA fragment. But we don't want those clusters to be too dense, which is shown in the middle, this is over-clustered. So the bars here are basically cluster densities. The two outside ones are good. The blue bars are total clusters, the green bar has filter clusters and the middle channel here is over-clustered, so the clusters are too high. This is just thousands per millimeter squared. The cluster density is too high. They're packed too closely together and the quality of data just drops off and the pass filter rate is low. So you want to avoid this. The other thing you want to avoid is being under-clustered. So this is an example of an under-clustered lane on a flow cell. This is what we're shooting for, somewhere in this area.
Transcript:
It's a pleasure to come here and talk about our experiences with the QuantStudio 3D, digital PCR platform. This is a technology that's been available for a while and it's essentially a limiting dilution assay, which has been done for many, many years. But now these instruments are becoming more accessible to core facilities and individual laboratories because the cost for the instruments are dropping. The last two that have been released, the instrumentation that you had to do this on was relatively expensive, but these are quite affordable. The QuantStudio™ is quite affordable and a low risk investment for the lab.
That's one reason we brought it in initially. The chip itself sets up to 20,000 individual reactions from a single master mix. And this is the type of data that you produce. Absolute quantitation, gives a count of the number of molecules in your volume and actually then just calculates back to what your original volume was. You can also do relative quantitation or quantification and I'll argue that you should always try to do this.
It's a fabulous way to do it because then you don't really have to worry about the DNA concentration going in as long as you're within the range of the instrument. The type of images that the software produces can be manually edited, especially the dot plots. You can drag that left and right if you want to change the threshold for the software to do the different calculations. If you don't like the threshold that the software automatically puts in, although I will say, we typically don't fiddle with this except I'll show one case at the end where we did, the numbers from the instrument, the software are almost always spot on.
The software does the Poisson calculation to correct for the numbers of doubles, triples, because we're looking at individual wells in this 20,000 reaction chip and some of them are doubles and triples.
We've been doing some copy number variation studies up in Ithaca and this is actually a small part of a 17 panel DNA set looking at a single copy gene or ended up being a single copy gene but all of them, we're looking at this was the relative quantitation, so we're expressing Taqman assay for FAM versus the Taqman® assay for VIC, which is FAM is on the Y axis, VIC is on the X axis and we just look at the ratios. We don't really care about the number of spots on that chip, it's really the ratios of those two different reporters and out of 17, this is only six of them I'm showing but out of 17 they looked almost identical, the variation among the 17 was about six percent, all these ratios. So it's really terrific for that.
What I want to get to our experiences with quantifying next generation sequencing libraries. This is the problem that we're trying to address. Those of you who have Illumina instruments, you know this problem. It's the clustering issue. So the upper left is an image of what happens on the surface of the flow cell. We generate millions of clusters derived from an original DNA fragment. But we don't want those clusters to be too dense, which is shown in the middle, this is over-clustered. So the bars here are basically cluster densities. The two outside ones are good. The blue bars are total clusters, the green bar has filter clusters and the middle channel here is over-clustered, so the clusters are too high. This is just thousands per millimeter squared. The cluster density is too high. They're packed too closely together and the quality of data just drops off and the pass filter rate is low. So you want to avoid this. The other thing you want to avoid is being under-clustered. So this is an example of an under-clustered lane on a flow cell. This is what we're shooting for, somewhere in this area.