filmov
tv
(EViews10): How to Perform GARCH Diagnostics #garch #diagnostics #garchdiagnostics #archdiagnostics
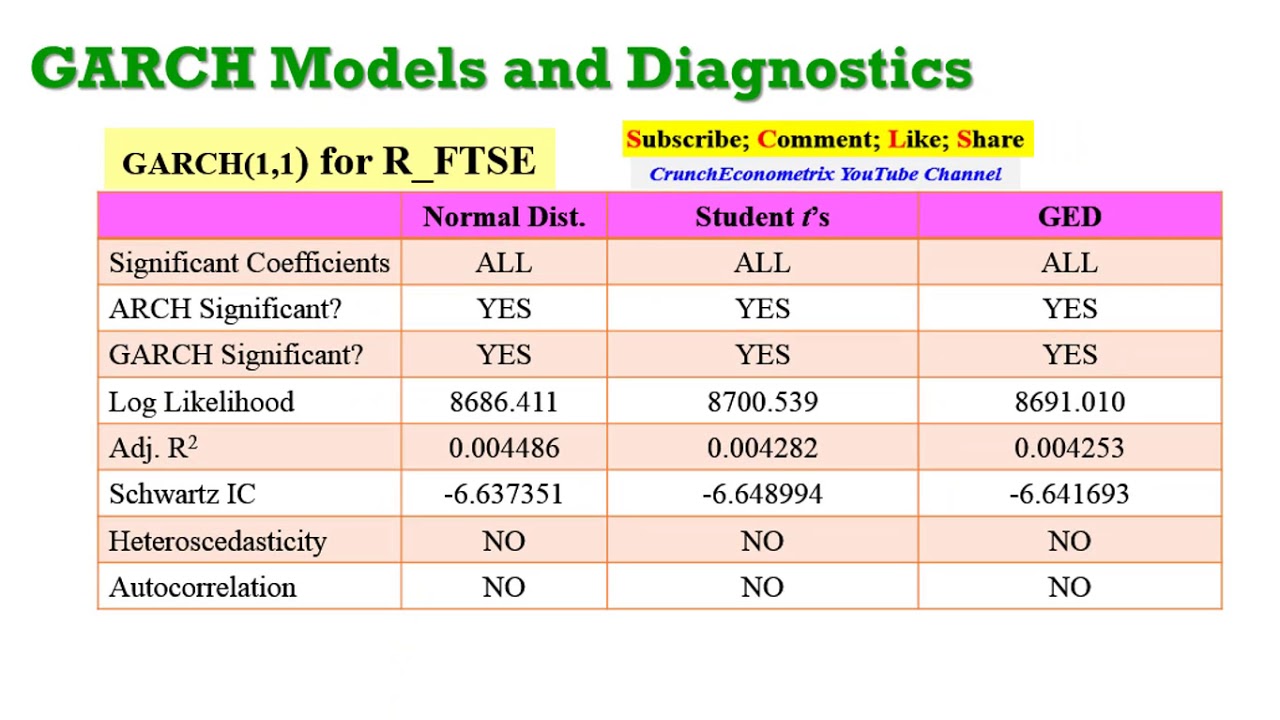
Показать описание
This video explains how to perform GARCH diagnostics using an approach that beginners can grasp. The GARCH Modeling series has 9 collections on the following topics: (1) ARCH versus GARCH (Background), (2) Basics of GARCH Modeling, (3) how to estimate a simple GARCH model, (4) ARCH versus GARCH (Estimations), (5) how to estimate GARCH-in-Mean models, (6) how to estimate Threshold GARCH (GJR GARCH) models, (7) how to estimate Exponential GARCH models, (8) GARCH models and diagnostics and (9) how to forecast GARCH volatility. So, what is GARCH? Generalised autoregressive indicates that heteroscedasticity observed over different time periods may be autocorrelated; conditional informs that variance is based on past errors; heteroscedasticity implies the series displays unequal variance. Popularised by Tim Bollerslev in 1986.
Why use GARCH: Models the attitude of investors not only towards expected returns but also towards risk (uncertainty); Relates to economic forecasting and measuring volatility; Techniques GARCH, GARCH-M, TGARCH, EGARCH, PGARCH, CGARCH, IGARCH and several other extensions; Concerned with modeling the volatility of the variance; Conditional and time-varying variance; Deals with stationary (time-invariant mean) and nonstationary (time-varying mean) variables; Nonstationary varying mean; Heteroscedastic varying variance; Concerns financial and macroeconomic time series; Duration daily, weekly, monthly, quarterly (high frequency data); Financial/economic series stock prices, oil prices, bond prices, inflation rates, exchange rates, interest rates, GDP, unemployment rates etc. What is conditional variance? The assumption of homoscedasticity (constant variance) is very limiting, hence preferable to examine patterns that allow the variance to depend (conditional) on its history. Volatility Clustering: Periods when large changes are followed by further large changes and periods when small changes are followed by further small changes. Shows wild and calm periods.
Some Lessons Learnt: The time-varying variance is modeled by the procedure called autoregressive conditional heteroscedasticity (ARCH); GARCH simply conveys that the series in question has a time-varying variance (heteroscedasticity) that depends on (conditional on) lagged effects (autocorrelation); GARCH model is intuitively appealing because it explains volatility as a function of the errors. These errors are called “shocks” or “news” by financial analysts. They represent the unexpected!; The larger the shocks, the greater the volatility in the series; Since variance is often used to measure volatility, and volatility is a key element in asset pricing theories, GARCH models have become important in empirical finance; Most financial time series like stock prices, exchange rates, oil prices etc. exhibit random walks in their level form, that is, nonstationary (time-varying means)
Need the data used in the video? Click on these links:
References and Readings: Asteriou and Hall (2016) Applied Econometrics, 3ed; Hill, Griffiths and Lim (2008) Principles of Econometrics, 3ed; Roman Kozhan (2010) Financial Econometrics with EViews; Gujarati and Porter (2009) Basic Econometrics, International Edition; R. Engle, “Autoregressive Conditional Heteroscedasticity with Estimates of the Variance of United Kingdom Inflation,” Econometrica, vol. 50. No. 1, 1982, pp. 987–1007; A. Bera and M. Higgins, “ARCH Models: Properties, Estimation and Testing,” Journal of Economic Surveys, vol. 7, 1993, pp. 305–366; Bollerslev (1986); Amadeus Wennström (2014) Volatility Forecasting Performance: Evaluation of GARCH type volatility models on Nordic equity indices; Bollerslev, T (1986)“Generalised Autoregressive Conditional Heteroscedasticity with Estimates of the Variance of United Kingdom Inflation,” Journal of Econometrica, vol. 31, pp. 307–327; Tsay, R.S. (2002) Analysis of Financial Time Series, John Wiley & Sons, Inc., New York.
Follow up with soft-notes and updates from CrunchEconometrix:
Why use GARCH: Models the attitude of investors not only towards expected returns but also towards risk (uncertainty); Relates to economic forecasting and measuring volatility; Techniques GARCH, GARCH-M, TGARCH, EGARCH, PGARCH, CGARCH, IGARCH and several other extensions; Concerned with modeling the volatility of the variance; Conditional and time-varying variance; Deals with stationary (time-invariant mean) and nonstationary (time-varying mean) variables; Nonstationary varying mean; Heteroscedastic varying variance; Concerns financial and macroeconomic time series; Duration daily, weekly, monthly, quarterly (high frequency data); Financial/economic series stock prices, oil prices, bond prices, inflation rates, exchange rates, interest rates, GDP, unemployment rates etc. What is conditional variance? The assumption of homoscedasticity (constant variance) is very limiting, hence preferable to examine patterns that allow the variance to depend (conditional) on its history. Volatility Clustering: Periods when large changes are followed by further large changes and periods when small changes are followed by further small changes. Shows wild and calm periods.
Some Lessons Learnt: The time-varying variance is modeled by the procedure called autoregressive conditional heteroscedasticity (ARCH); GARCH simply conveys that the series in question has a time-varying variance (heteroscedasticity) that depends on (conditional on) lagged effects (autocorrelation); GARCH model is intuitively appealing because it explains volatility as a function of the errors. These errors are called “shocks” or “news” by financial analysts. They represent the unexpected!; The larger the shocks, the greater the volatility in the series; Since variance is often used to measure volatility, and volatility is a key element in asset pricing theories, GARCH models have become important in empirical finance; Most financial time series like stock prices, exchange rates, oil prices etc. exhibit random walks in their level form, that is, nonstationary (time-varying means)
Need the data used in the video? Click on these links:
References and Readings: Asteriou and Hall (2016) Applied Econometrics, 3ed; Hill, Griffiths and Lim (2008) Principles of Econometrics, 3ed; Roman Kozhan (2010) Financial Econometrics with EViews; Gujarati and Porter (2009) Basic Econometrics, International Edition; R. Engle, “Autoregressive Conditional Heteroscedasticity with Estimates of the Variance of United Kingdom Inflation,” Econometrica, vol. 50. No. 1, 1982, pp. 987–1007; A. Bera and M. Higgins, “ARCH Models: Properties, Estimation and Testing,” Journal of Economic Surveys, vol. 7, 1993, pp. 305–366; Bollerslev (1986); Amadeus Wennström (2014) Volatility Forecasting Performance: Evaluation of GARCH type volatility models on Nordic equity indices; Bollerslev, T (1986)“Generalised Autoregressive Conditional Heteroscedasticity with Estimates of the Variance of United Kingdom Inflation,” Journal of Econometrica, vol. 31, pp. 307–327; Tsay, R.S. (2002) Analysis of Financial Time Series, John Wiley & Sons, Inc., New York.
Follow up with soft-notes and updates from CrunchEconometrix:
Комментарии