filmov
tv
(EViews10) - How to Estimate ARCH Models #arch #timeseries #volatility #modeling #econometrics
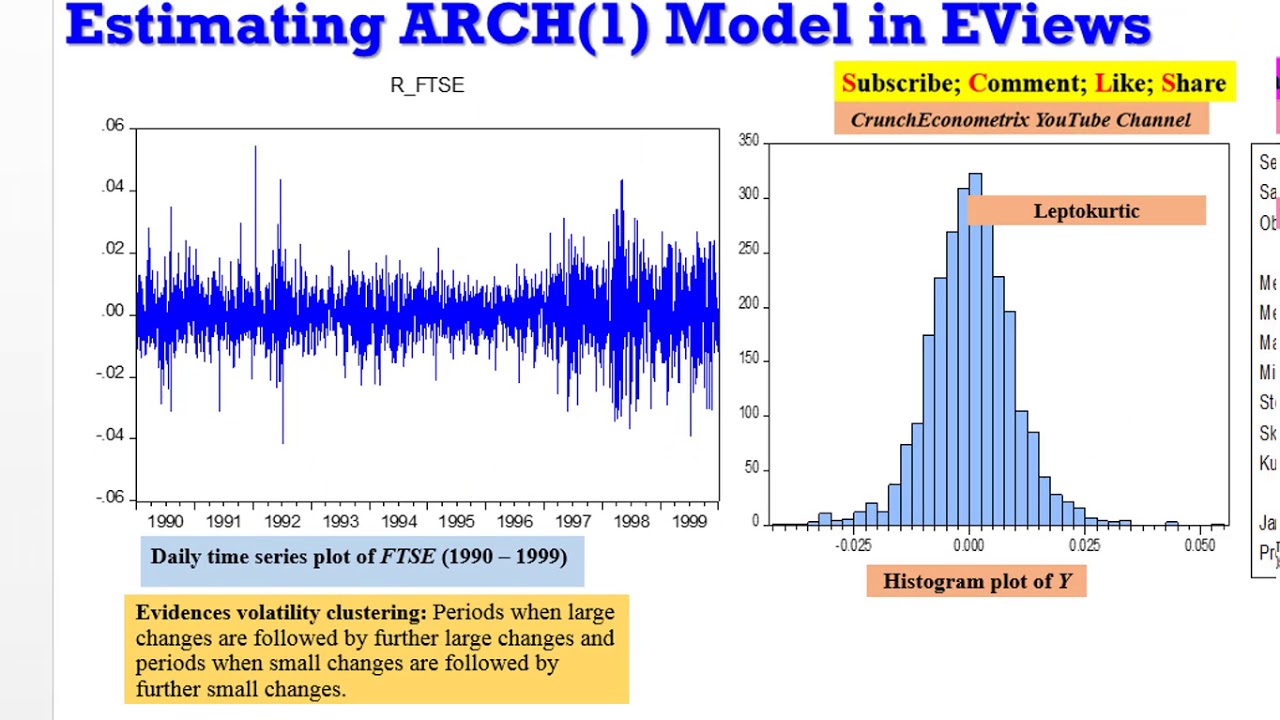
Показать описание
This video simplifies the understanding of the autoregressive conditional heteroscedasticity (ARCH) using an approach that beginners can grasp. The video series will contain four other tutorials: (1) How to Simulate ARCH model; (2) How to Test for the presence of ARCH Effects; (3) How to Estimate ARCH Models and (4) How to Forecast ARCH Volatility. So, what is ARCH? Autoregressive indicates that heteroscedasticity observed over different time periods may be autocorrelated; conditional informs that variance is based on past errors; heteroscedasticity implies the series displays unequal variance. Popularised by Nobel Prize Winner, Robert F. Engel (1982)
Why use ARCH: Models the attitude of investors not only towards expected returns but also towards risk (uncertainty); Relates to economic forecasting and measuring volatility; Techniques ARCH, ARCH-M, GARCH, GARCH-M, TGARCH and EGARCH; Concerned with modeling the volatility of the variance; Conditional and time-varying variance; Deals with stationary (time-invariant mean) and nonstationary (time-varying mean) variables; Nonstationary varying mean; Heteroscedastic varying variance; Concerns financial and macroeconomic time series; Duration daily, weekly, monthly, quarterly (high frequency data); Financial/economic series stock prices, oil prices, bond prices, inflation rates, exchange rates, interest rates, GDP, unemployment rates etc.
What is conditional variance? The assumption of homoscedasticity (constant variance) is very limiting, hence preferable to examine patterns that allow the variance to depend (conditional) on its history. Volatility: When the values of financial variables change rapidly from time to time in an apparently unpredictable manner. Volatility Clustering: Periods when large changes are followed by further large changes and periods when small changes are followed by further small changes. Shows wild and calm periods.
The ARCH Estimator: The presence of ARCH does not affect consistency of OLS. Still has desirable properties under ARCH. OLS yields consistent but inefficient estimates. Estimates of the covariance matrix will be biased. Leading to invalid t-statistics. Remember, these are valid for any form of heteroskedasticity, and ARCH is just one particular form of heteroskedasticity. An efficient estimator is required maximum likelihood algorithm.
Some Lessons Learnt: The time-varying variance is modeled by the procedure called autoregressive conditional heteroscedasticity (ARCH); ARCH simply conveys that the series in question has a time-varying variance (heteroscedasticity) that depends on (conditional on) lagged effects (autocorrelation); ARCH model is intuitively appealing because it explains volatility as a function of the errors. These errors are called “shocks” or “news” by financial analysts. They represent the unexpected!; The larger the shocks, the greater the volatility in the series; Since variance is often used to measure volatility, and volatility is a key element in asset pricing theories, ARCH models have become important in empirical finance; Most financial time series like stock prices, exchange rates, oil prices etc. exhibit random walks in their level form, that is, nonstationary (time-varying means); But stationary at 1st difference which often exhibit wide swings or volatility; Wide swings suggest that the variance of the financial time series changes over time (time-varying volatility); Volatility clustering big changes in u_t are fed into further big changes in h_t via the lagged effects u_(t-1); ARCH modeling has become increasingly popular; useful for modeling volatility; especially changes in volatility over time (that is, time-varying volatility).
References and Readings: Asteriou and Hall (2016) Applied Econometrics, 3ed; Hill, Griffiths and Lim (2008) Principles of Econometrics, 3ed; Roman Kozan (2010) Financial Econometrics with EViews; Gujarati and Damodar (2009) Basic Econometrics, International Edition; R. Engle, “Autoregressive Conditional Heteroscedasticity with Estimates of the Variance of United Kingdom Inflation,” Econometrica, vol. 50. No. 1, 1982, pp. 987–1007; A. Bera and M. Higgins, “ARCH Models: Properties, Estimation and Testing,” Journal of Economic Surveys, vol. 7, 1993, pp. 305–366.
Follow up with soft-notes and updates from CrunchEconometrix:
Why use ARCH: Models the attitude of investors not only towards expected returns but also towards risk (uncertainty); Relates to economic forecasting and measuring volatility; Techniques ARCH, ARCH-M, GARCH, GARCH-M, TGARCH and EGARCH; Concerned with modeling the volatility of the variance; Conditional and time-varying variance; Deals with stationary (time-invariant mean) and nonstationary (time-varying mean) variables; Nonstationary varying mean; Heteroscedastic varying variance; Concerns financial and macroeconomic time series; Duration daily, weekly, monthly, quarterly (high frequency data); Financial/economic series stock prices, oil prices, bond prices, inflation rates, exchange rates, interest rates, GDP, unemployment rates etc.
What is conditional variance? The assumption of homoscedasticity (constant variance) is very limiting, hence preferable to examine patterns that allow the variance to depend (conditional) on its history. Volatility: When the values of financial variables change rapidly from time to time in an apparently unpredictable manner. Volatility Clustering: Periods when large changes are followed by further large changes and periods when small changes are followed by further small changes. Shows wild and calm periods.
The ARCH Estimator: The presence of ARCH does not affect consistency of OLS. Still has desirable properties under ARCH. OLS yields consistent but inefficient estimates. Estimates of the covariance matrix will be biased. Leading to invalid t-statistics. Remember, these are valid for any form of heteroskedasticity, and ARCH is just one particular form of heteroskedasticity. An efficient estimator is required maximum likelihood algorithm.
Some Lessons Learnt: The time-varying variance is modeled by the procedure called autoregressive conditional heteroscedasticity (ARCH); ARCH simply conveys that the series in question has a time-varying variance (heteroscedasticity) that depends on (conditional on) lagged effects (autocorrelation); ARCH model is intuitively appealing because it explains volatility as a function of the errors. These errors are called “shocks” or “news” by financial analysts. They represent the unexpected!; The larger the shocks, the greater the volatility in the series; Since variance is often used to measure volatility, and volatility is a key element in asset pricing theories, ARCH models have become important in empirical finance; Most financial time series like stock prices, exchange rates, oil prices etc. exhibit random walks in their level form, that is, nonstationary (time-varying means); But stationary at 1st difference which often exhibit wide swings or volatility; Wide swings suggest that the variance of the financial time series changes over time (time-varying volatility); Volatility clustering big changes in u_t are fed into further big changes in h_t via the lagged effects u_(t-1); ARCH modeling has become increasingly popular; useful for modeling volatility; especially changes in volatility over time (that is, time-varying volatility).
References and Readings: Asteriou and Hall (2016) Applied Econometrics, 3ed; Hill, Griffiths and Lim (2008) Principles of Econometrics, 3ed; Roman Kozan (2010) Financial Econometrics with EViews; Gujarati and Damodar (2009) Basic Econometrics, International Edition; R. Engle, “Autoregressive Conditional Heteroscedasticity with Estimates of the Variance of United Kingdom Inflation,” Econometrica, vol. 50. No. 1, 1982, pp. 987–1007; A. Bera and M. Higgins, “ARCH Models: Properties, Estimation and Testing,” Journal of Economic Surveys, vol. 7, 1993, pp. 305–366.
Follow up with soft-notes and updates from CrunchEconometrix:
Комментарии