filmov
tv
Probability 8: What is wrong with NHST, p-values, and Significance Testing?
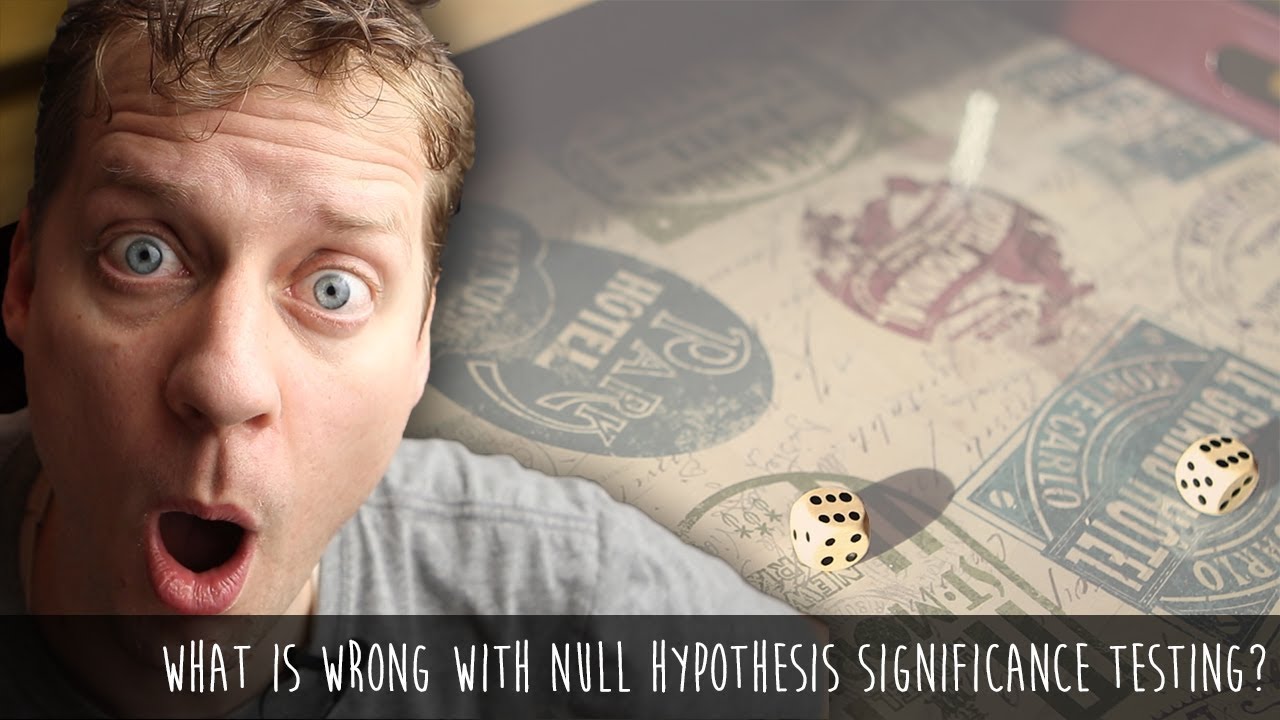
Показать описание
Relevant videos:
Relevant Papers:
Learning objectives:
#1. How ethics relates to sampling theory/significance testing
#2. How p-hacking inflates p-values
#3. Required conditions to correctly interpret a p-value
#4. Relationship between strict CDA and p-values
#5. Alternatives to NHST
Technical sidenote:
Relevant Papers:
Learning objectives:
#1. How ethics relates to sampling theory/significance testing
#2. How p-hacking inflates p-values
#3. Required conditions to correctly interpret a p-value
#4. Relationship between strict CDA and p-values
#5. Alternatives to NHST
Technical sidenote:
Probability 8: What is wrong with NHST, p-values, and Significance Testing?
How statistics can be misleading - Mark Liddell
Probability of a Dice Roll | Statistics & Math Practice | JusticeTheTutor #shorts #math #maths
Experimental vs Theoretical Probability
Probability of correctly guessing answer
Ch 8: Why is probability equal to amplitude squared? | Maths of Quantum Mechanics
How Casinos Exploit Our Limited Understanding of Statistics & Probability | Neil deGrasse Tyson
Humans are HORRIBLE at statistics and probability. Neil deGrasse Tyson with Joe Rogan
The medical test paradox, and redesigning Bayes' rule
Probability
The Monty Hall problem, casually explained - #probability #statistics #stemeducation
Probability of guessing 4 out of 5 questions right
The birthday problem: Why we all have it wrong? (classics in probability theory)
Roll A Pair Of Dice | Probability Math Problem
The Simple Question that Stumped Everyone Except Marilyn vos Savant
Calculating Power and the Probability of a Type II Error (A One-Tailed Example)
Probability of Being A Boy? #mathematics #probability
The Two Envelope Problem - a Mystifying Probability Paradox
Math 14 RA 4.2.5 What is the probability that someone does not have this belief?
Tree diagram probability examples,
📚 How to solve problems involving probability
PROBABILITY but it keeps getting HARDER!!! (how far can you get?)
What is entropy? - Jeff Phillips
Different Types of Events in Probability #probability #datascience #probabilitywithav #learnwithav
Комментарии