filmov
tv
Causal Effects via the Do-operator | Overview & Example
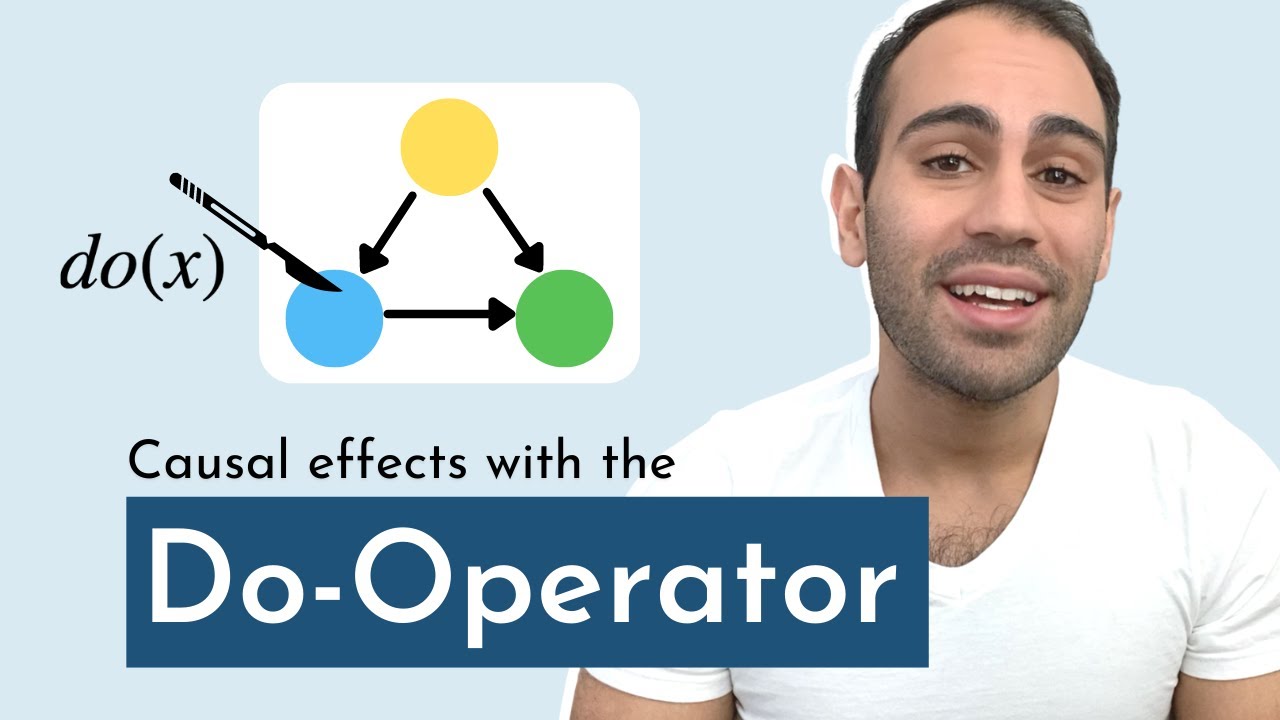
Показать описание
This is the 3rd video in a series on causal effects. Here I discuss a new way to formulate the average treatment effect (ATE) using the do-operator. This alternative formulation unlocks new paths toward estimating causal effects from observational data.
Resources:
--
Introduction - 0:00
Observational vs Interventional Data - 0:35
2 Formulations of ATE - 2:23
do-operator - 5:26
Identifiability - 7:05
Truncated Factorization Formula - 10:34
Coping with Unmeasured Confounders - 10:52
Interventional Distribution via Parents - 12:34
Key Points - 13:08
Resources:
--
Introduction - 0:00
Observational vs Interventional Data - 0:35
2 Formulations of ATE - 2:23
do-operator - 5:26
Identifiability - 7:05
Truncated Factorization Formula - 10:34
Coping with Unmeasured Confounders - 10:52
Interventional Distribution via Parents - 12:34
Key Points - 13:08
Causal Effects via the Do-operator | Overview & Example
Causal Effects via DAGs | How to Handle Unobserved Confounders
4.2 - Intervening, the do-operator, and Identifiability
Understanding the Do Operator in Causal AI
Causal Effects via Regression w/ Python Code
Causal Inference - Lecture 1.2 | Average (or aggregated) causal effects
Causal Effects | An introduction
Causal Effects via Propensity Scores | Introduction & Python Code
Richard Guo - Seminar - 'Possible Causal Effects: Enumeration and Estimation'
Causal Inference | Answering causal questions
Adversarial Machine Learning and Instrumental Variables for Flexible Causal Modeling
Causality: Interventions | Part A
2022-12-19 Causality - Do Calculus
Propensity scores - A Crash Course in Causality: Inferring Causal Effects from Observational Data
8.3 - Identification in Linear Setting
12.3 - Transportability of Causal Effects Across Populations
Rui Song: On causal decision making
Marloes Maathuis: Combining causal structure learning and covariate adjustment
5.4 - Pearl’s do-calculus
Patrick Blöbaum: Performing Root Cause Analysis with DoWhy, a Causal Machine-Learning Library
Rodrigo Pinto: What is causality? How to express it? And why it matters
Erik Hoel: A framework for effectively measuring the causal emergence(TBC)
Antti Hyttinen and Jussi Viinikka - On the Identifiability and Estimation of Causal Effects
Causal Discovery | Inferring causality from observational data
Комментарии