filmov
tv
Causal Effects via DAGs | How to Handle Unobserved Confounders
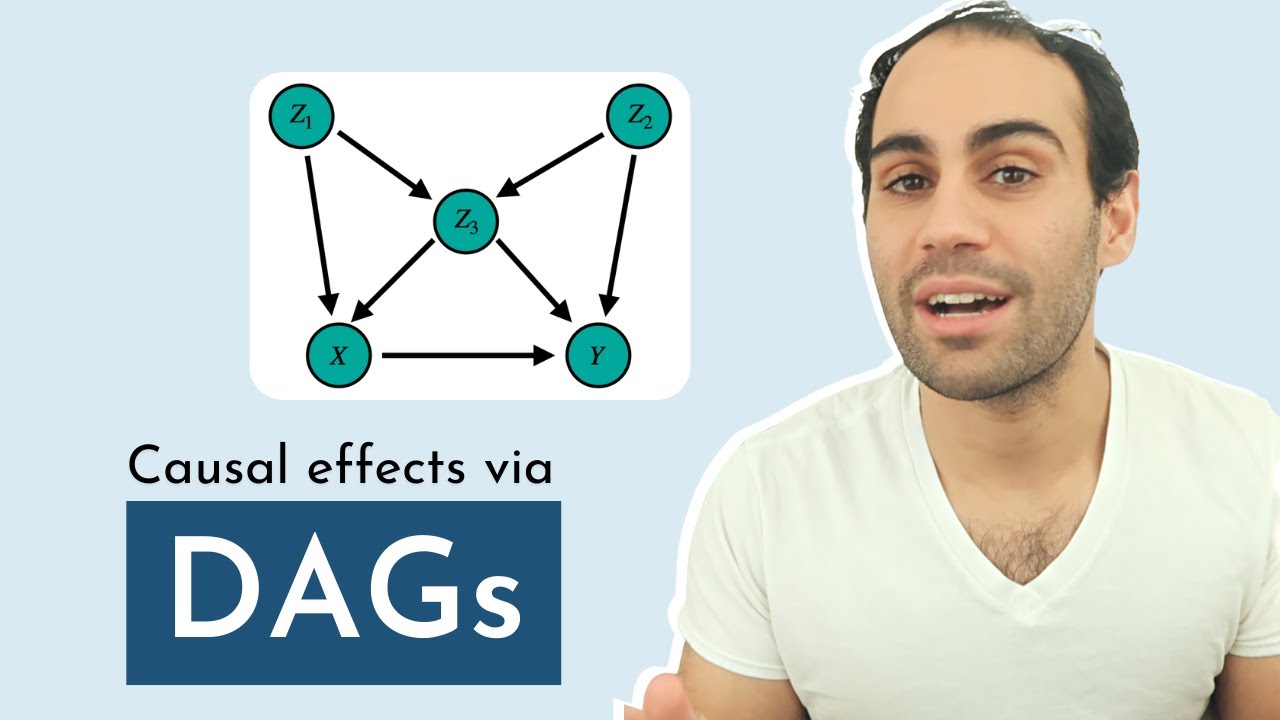
Показать описание
This is the 4th video in a series on causal effects. In the last video, we saw that we could evaluate any causal effect for a Markovian causal model. However, the question remained of how to handle models that are not Markovian. In this video, we start to answer this question via two quick-and-easy graphical criteria for evaluating causal effects.
Resources:
--
Introduction - 0:00
Identifiability - 0:28
Markovian Models - 2:12
Unobserved Confounders - 3:19
Back & Front Door Criteria - 4:18
Back Door Path - 4:44
Blocking - 5:22
Back Door Criterion - 7:27
Front Door Criterion - 9:14
Resources:
--
Introduction - 0:00
Identifiability - 0:28
Markovian Models - 2:12
Unobserved Confounders - 3:19
Back & Front Door Criteria - 4:18
Back Door Path - 4:44
Blocking - 5:22
Back Door Criterion - 7:27
Front Door Criterion - 9:14
Causal Effects via DAGs | How to Handle Unobserved Confounders
Building and Using DAGs for Causal Inference
Causal Effects via the Do-operator | Overview & Example
Directed Acyclic Graphs (DAGs)
PMAP 8521 • (4) Measurement and DAGs: (2) Causal models
PMAP 8521 • (4) Measurement and DAGs: (3) Paths, doors, and adjustment
Causal Effects via Regression w/ Python Code
Identifying causal effects in maximally oriented partially directed acyclic graphs
Emilija Perkovic: Causal effects in maximally oriented partially directed acyclic graphs (MPDAGs)
Part 4—Directed Acyclic Graphs (DAGs) for Causal Inference in Tobacco Research
What is DAG?
Causal Effects via Propensity Scores | Introduction & Python Code
Johannes Textor: Causal Inference using the R package DAGitty
Causal DAGs 101
4.6 - The Backdoor Adjustment
An Introduction to Potential Outcomes, DAGs and Single-World Intervention Graphs
CECTR Webinar Series Part 4—Directed Acyclic Graphs (DAGs) for Causal Inference in Tobacco Research...
Causal Effects | An introduction
Causal Inference - EXPLAINED!
How Markov assumption links DAG to data
Introduction to Causal Inference and Directed Acyclic Graphs
Selection Bias Graphically - Causal Inference
Estimating Causal Effects: Regression
Richard Guo - Seminar - 'Possible Causal Effects: Enumeration and Estimation'
Комментарии