filmov
tv
Causal Effects | An introduction
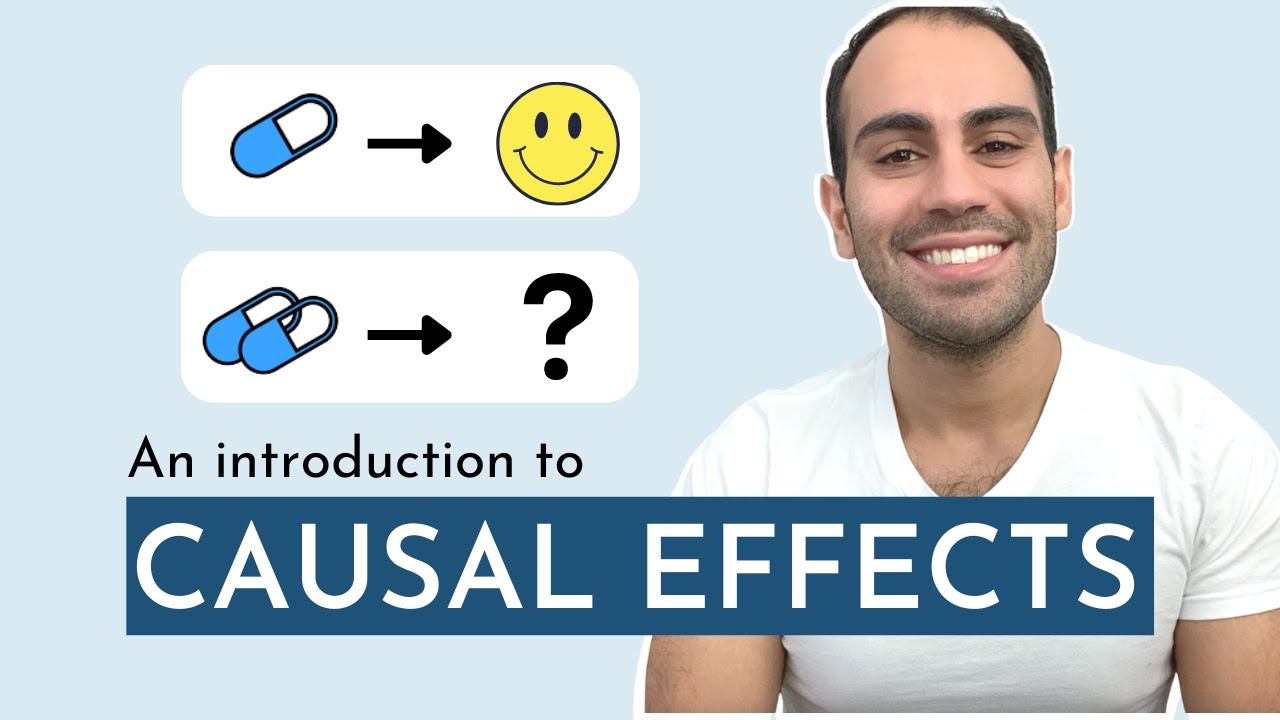
Показать описание
This is the first video in a series on causal effects. Here I introduce the Potential Outcomes Framework and use it to formulate 3 different types of causal effects. In future videos, I discuss how to compute causal effects from observational data.
Resources:
- An Introduction to Propensity Score Methods for Reducing the Effects of Confounding in Observational Studies by Peter C. Austin
- Counterfactuals and Causal Inference: Methods and Principles for Social Research by Stephen L. Morgan & Christopher Winship
- An Introduction to Causal Inference by Judea Pearl
--
Socials
The Data Entrepreneurs
Support ❤️
Introduction - 0:00
Causal Effects - 0:24
3 Types of Variables - 1:01
Potential Outcomes Framework - 1:50
3 Types of Causal Effects - 2:28
1) Individual Treatment Effect (ITE) - 2:38
2) Average Treatment Effect (ATE) - 4:01
2.1) ATE in RCTs - 5:24
3) Average Treatment Effect of Treated/Controls (ATT/ATC) - 6:56
Practical Questions - 9:07
Resources:
- An Introduction to Propensity Score Methods for Reducing the Effects of Confounding in Observational Studies by Peter C. Austin
- Counterfactuals and Causal Inference: Methods and Principles for Social Research by Stephen L. Morgan & Christopher Winship
- An Introduction to Causal Inference by Judea Pearl
--
Socials
The Data Entrepreneurs
Support ❤️
Introduction - 0:00
Causal Effects - 0:24
3 Types of Variables - 1:01
Potential Outcomes Framework - 1:50
3 Types of Causal Effects - 2:28
1) Individual Treatment Effect (ITE) - 2:38
2) Average Treatment Effect (ATE) - 4:01
2.1) ATE in RCTs - 5:24
3) Average Treatment Effect of Treated/Controls (ATT/ATC) - 6:56
Practical Questions - 9:07
Causal Effects | An introduction
Defining Causal Effects
Causal Inference - EXPLAINED!
Causal Effects via Propensity Scores | Introduction & Python Code
What Is A Causal Effect? |【Five Minute Econometrics】Topic 2
Causal Effects via DAGs | How to Handle Unobserved Confounders
An introduction to Causal Inference with Python – making accurate estimates of cause and effect from...
three strategies to estimate causal effects
The Truth About Time: Physics, Philosophy, and Future Tech
Causality - an introduction
Causal Effects via the Do-operator | Overview & Example
Causation in econometrics - selection bias and average causal effect
The Rubin Causal model - an introduction
Correlation vs Causation (Statistics)
Causal Inference Introduction
Estimating Causal Effects
Average Treatment Effects: Causal Inference Bootcamp
Unit Level Effects: Causal Inference Bootcamp
Causal Inference in Python: Theory to Practice
24. Introduction to Econometrics: Estimation of Dynamic Causal Effects (Part A)
Introduction to the Causal Inference Bootcamp
Causal Effects via Regression w/ Python Code
The average causal effect - an example
Causality and counterfactuals
Комментарии