filmov
tv
Python Tutorial: Small multiples
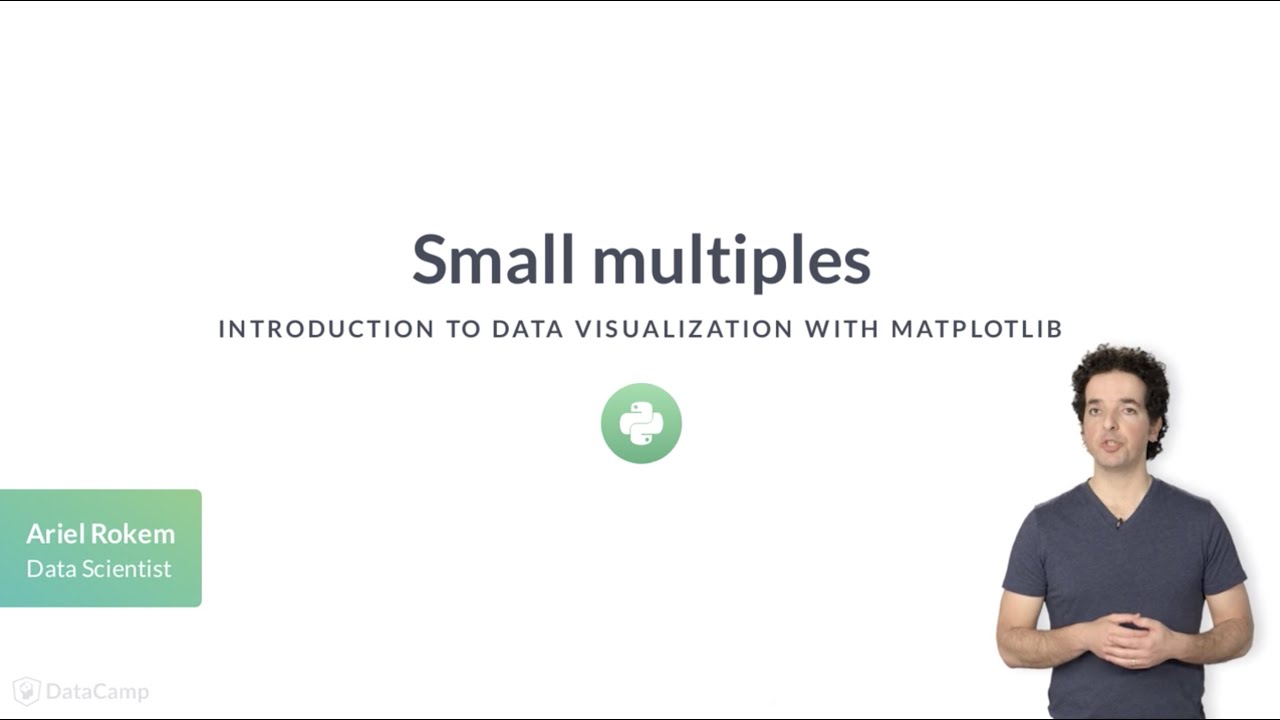
Показать описание
---
In some cases, adding more data to a plot can make the plot too busy, obscuring patterns rather than revealing them.
For example, let's explore the data we have about weather in Seattle. Here we plot average precipitation in Seattle during the course of the year.
But let's say that we are also interested in the range of values.
We add the 25th percentile and the 75th percentile of the precipitation in dashed lines above and below the average.
What would happen if we compared this to Austin?
This code adds the data from Austin to the plot. When we display the plot,
it's a bit of a mess. There's too much data in this plot.
One way to overcome this kind of mess is to use what are called small multiples. These are multiple small plots that show similar data across different conditions. For example, precipitation data across different cities.
In Matplotlib, small multiples are called sub-plots. That is also the reason that the function that creates these is called subplots. Previously, we called this function with no inputs. This creates one subplot. Now, we'll give it some inputs.
Small multiples are typically arranged on the page as a grid with rows and columns. Here, we are creating a Figure object with three rows of subplots, and two columns.
This is what this would look like before we add any data to it.
In this case, the variable ax is no longer only one Axes object.
Instead, it is an array of Axes objects with a shape of 3 by 2.
To add data, we would now have to index into this object and call the plot method on an element of the array.
There is a special case for situations where you have only one row or only one column of plots.
In this case, the resulting array will be one-dimensional and you will only have to provide one index to access the elements of this array.
For example, consider what we might do with the rainfall data that we were plotting before.
We create a figure and an array of Axes objects with two rows and one column.
We address the first element in this array, which is the top sub-plot, and add the data for Seattle to this plot.
Then, we address the second element in the array, which is the bottom plot, and add the data from Austin to it.
We can add a y-axis label to each one of these.
Because they are one on top of the other, we only add an x-axis label to the bottom plot, by addressing only the second element in the array of Axes objects.
When we show this,
we see that the data are now cleanly presented in a way that facilitates the direct comparison between the two cities.
One thing we still need to take care of is the range of the y-axis in the two plots, which is not exactly the same. This is because the highest and lowest values in the two datasets are not identical.
To make sure that all the subplots have the same range of y-axis values, we initialize the figure and its subplots with the key-word argument sharey set to True. This means that both subplots will have the same range of y-axis values, based on the data from both datasets. Now the comparison across datasets is more straightforward.
Next, go ahead and practice creating visualizations with small multiples.
#Python #PythonTutorial #DataCamp #Data #Visualization #Matplotlib