filmov
tv
Apache Spark Memory Management | Unified Memory Management
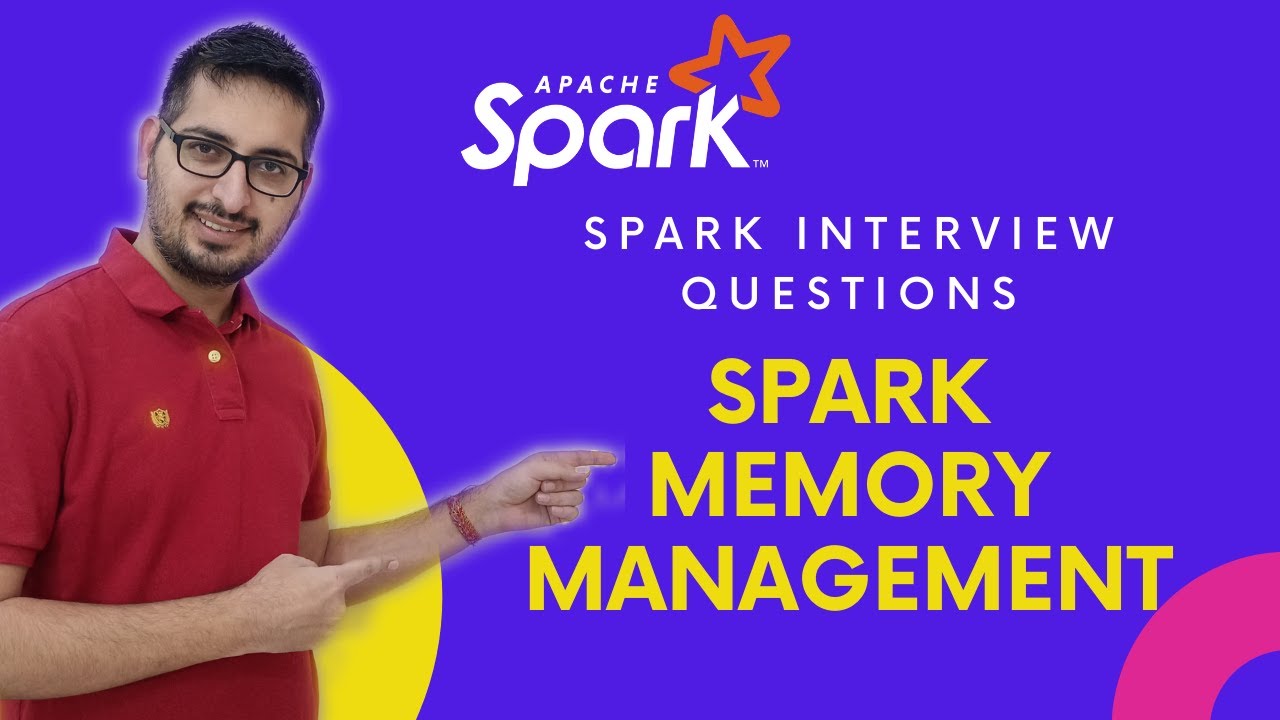
Показать описание
In this comprehensive video, we dive into the crucial topic of memory management in Apache Spark. Memory plays a vital role in the performance and resource utilization of Spark applications, and understanding the memory management mechanisms is key to optimizing your Spark jobs.
Join us as we explore the inner workings of Spark's memory management and uncover strategies to enhance the efficiency of your data processing workflows. This video covers a range of memory-related concepts, including:
Spark Memory Architecture:
Gain insights into the different memory regions in Spark, such as the Execution Memory, Storage Memory, and User Memory.
Understand how these memory regions are allocated and utilized during Spark job execution.
Learn about the significance of the Memory Manager and its role in managing memory resources.
Memory Allocation and Configuration:
Learn best practices for setting optimal memory configurations based on your application's requirements and available cluster resources.
Memory Usage and Monitoring:
Explore techniques for monitoring memory usage in Spark applications, including tools like Spark Web UI and monitoring APIs.
Understand how to interpret memory metrics and diagnose memory-related issues.
Learn strategies for optimizing memory usage, such as data serialization and caching.
Garbage Collection and Memory Tuning:
Delve into Spark's garbage collection (GC) mechanisms and their impact on memory management.
Discover techniques for tuning garbage collection settings to achieve better memory utilization and minimize GC overhead.
By the end of this video, you'll have a solid understanding of Apache Spark's memory management mechanisms and practical insights into optimizing memory usage for improved performance and resource efficiency.
Whether you're a data engineer, data scientist, or Spark enthusiast, this video will equip you with valuable knowledge to fine-tune memory management in your Spark applications.
Don't miss out on this opportunity to enhance your expertise in Apache Spark memory management. Hit play and unlock the secrets to optimizing performance and resource utilization in your Spark jobs!
Join us as we explore the inner workings of Spark's memory management and uncover strategies to enhance the efficiency of your data processing workflows. This video covers a range of memory-related concepts, including:
Spark Memory Architecture:
Gain insights into the different memory regions in Spark, such as the Execution Memory, Storage Memory, and User Memory.
Understand how these memory regions are allocated and utilized during Spark job execution.
Learn about the significance of the Memory Manager and its role in managing memory resources.
Memory Allocation and Configuration:
Learn best practices for setting optimal memory configurations based on your application's requirements and available cluster resources.
Memory Usage and Monitoring:
Explore techniques for monitoring memory usage in Spark applications, including tools like Spark Web UI and monitoring APIs.
Understand how to interpret memory metrics and diagnose memory-related issues.
Learn strategies for optimizing memory usage, such as data serialization and caching.
Garbage Collection and Memory Tuning:
Delve into Spark's garbage collection (GC) mechanisms and their impact on memory management.
Discover techniques for tuning garbage collection settings to achieve better memory utilization and minimize GC overhead.
By the end of this video, you'll have a solid understanding of Apache Spark's memory management mechanisms and practical insights into optimizing memory usage for improved performance and resource efficiency.
Whether you're a data engineer, data scientist, or Spark enthusiast, this video will equip you with valuable knowledge to fine-tune memory management in your Spark applications.
Don't miss out on this opportunity to enhance your expertise in Apache Spark memory management. Hit play and unlock the secrets to optimizing performance and resource utilization in your Spark jobs!
Комментарии