filmov
tv
Deep Lidar CNN to Understand the Dynamics of Moving Vehicles
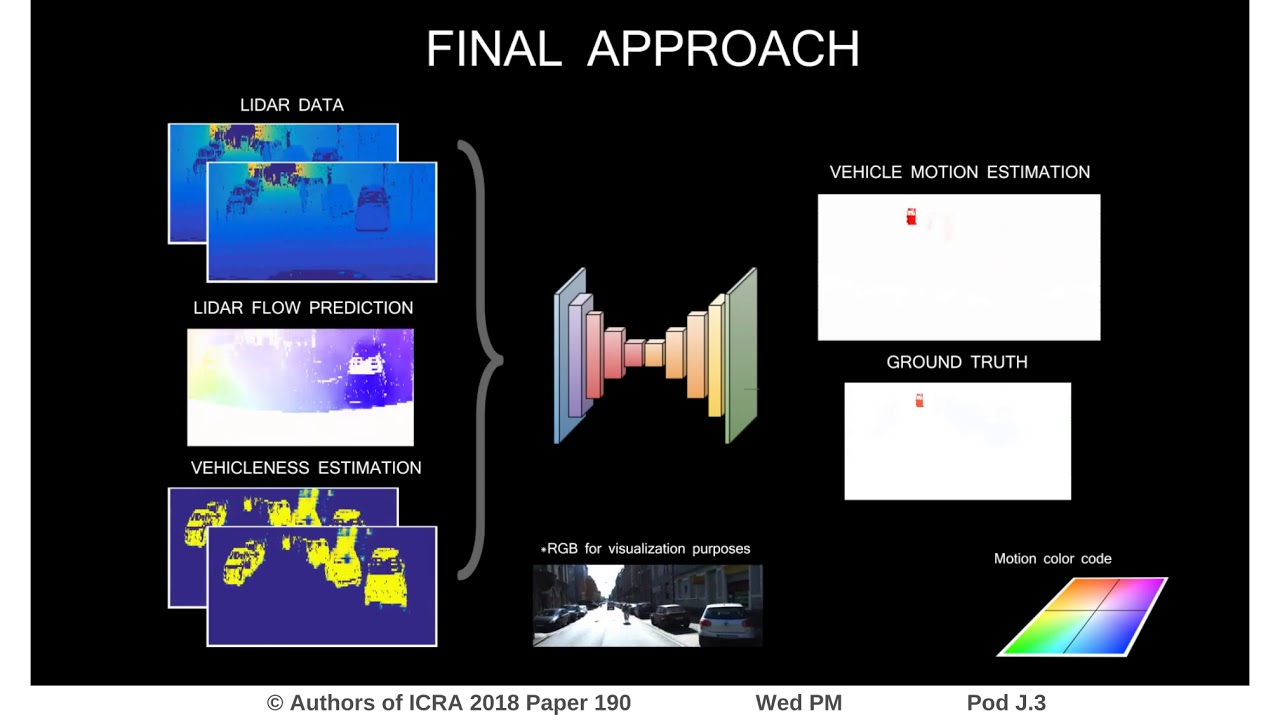
Показать описание
ICRA 2018 Spotlight Video
Interactive Session Wed PM Pod J.3
Authors: Vaquero, Victor; Sanfeliu, Alberto; Moreno-Noguer, Francesc
Title: Deep Lidar CNN to Understand the Dynamics of Moving Vehicles
Abstract:
Perception technologies in Autonomous Driving are experiencing their golden age due to the advances in Deep Learning. Yet, most of these systems rely on the semantically rich information of RGB images. Deep Learning solutions applied to the data of other sensors typically mounted on autonomous cars (e.g. lidars or radars) are not explored much. In this paper we propose a novel solution to understand the dynamics of moving vehicles of the scene from only lidar information. The main challenge of this problem stems from the fact that we need to disambiguate the proprio-motion of the observer" vehicle from that of the external observed" vehicles. For this purpose, we devise a CNN architecture which at testing time is fed with pairs of consecutive lidar scans. However, in order to properly learn the parameters of this network, during training we introduce a series of so-called pretext tasks which also leverage on image data. These tasks include semantic information about vehicleness and a novel lidar-flow feature which combines standard image-based optical flow with lidar scans. We obtain very promising results and show that including distilled image information only during training, allows improving the inference results of the network at test time, even when image data is no longer used.
Interactive Session Wed PM Pod J.3
Authors: Vaquero, Victor; Sanfeliu, Alberto; Moreno-Noguer, Francesc
Title: Deep Lidar CNN to Understand the Dynamics of Moving Vehicles
Abstract:
Perception technologies in Autonomous Driving are experiencing their golden age due to the advances in Deep Learning. Yet, most of these systems rely on the semantically rich information of RGB images. Deep Learning solutions applied to the data of other sensors typically mounted on autonomous cars (e.g. lidars or radars) are not explored much. In this paper we propose a novel solution to understand the dynamics of moving vehicles of the scene from only lidar information. The main challenge of this problem stems from the fact that we need to disambiguate the proprio-motion of the observer" vehicle from that of the external observed" vehicles. For this purpose, we devise a CNN architecture which at testing time is fed with pairs of consecutive lidar scans. However, in order to properly learn the parameters of this network, during training we introduce a series of so-called pretext tasks which also leverage on image data. These tasks include semantic information about vehicleness and a novel lidar-flow feature which combines standard image-based optical flow with lidar scans. We obtain very promising results and show that including distilled image information only during training, allows improving the inference results of the network at test time, even when image data is no longer used.