filmov
tv
Derivation of Recursive Least Squares Method from Scratch - Introduction to Kalman Filter
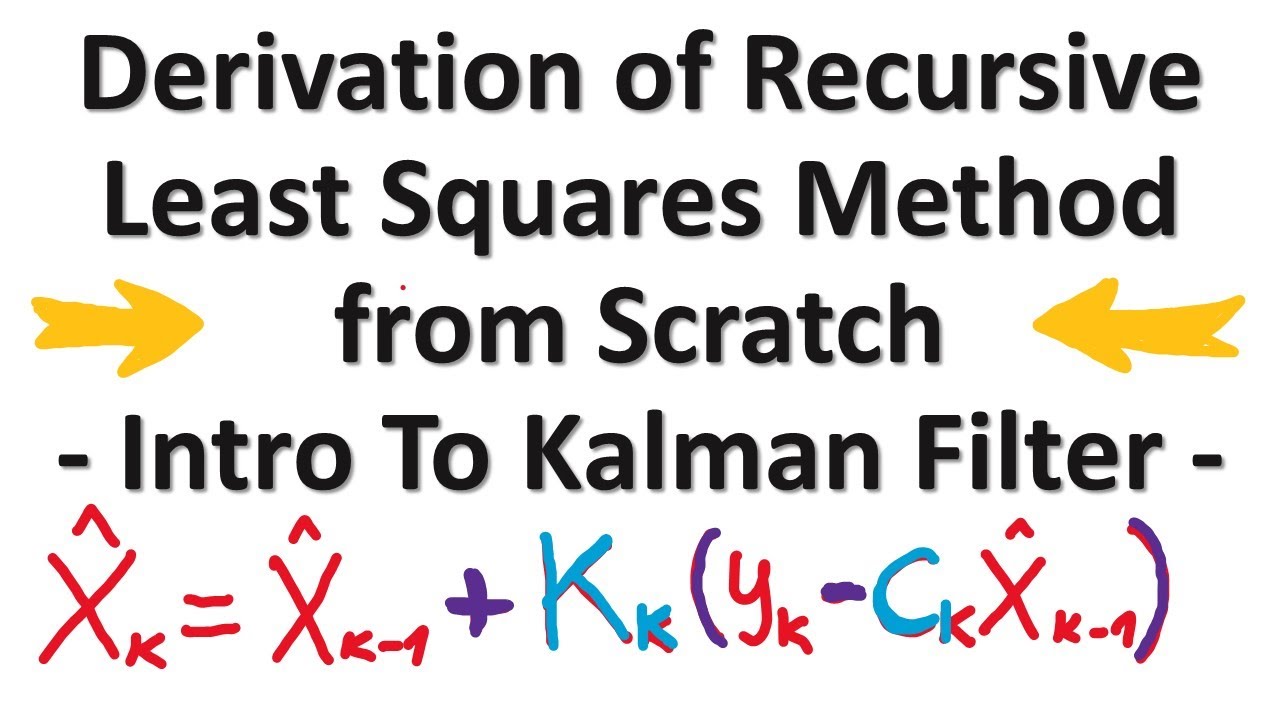
Показать описание
#kalmanfilter #estimation #controlengineering #controltheory #mechatronics #adaptivecontrol #adaptivefiltering #adaptivefilter #roboticsengineering #roboticslab #robotics #electricalengineering #controlengineering #pidcontrol #roboticseducation
It takes a significant amount of time and energy to create these free video tutorials. You can support my efforts in this way:
- You Can also press the Thanks YouTube Dollar button
The webpage accompanying this video is given here:
In this video tutorial and in the accompanying web tutorial, we explain how to derive a recursive least squares method from scratch. The recursive least squares method is a very important method since it serves as the basis of adaptive control, adaptive estimation, Kalman filter, and machine learning algorithms. In this video, we start with the measurement equation, and by formulating the cost function that sums the variances of the estimation error, and by solving this cost function we obtain the recursive least squares gain matrix. We also derive an expression for the propagation of the estimation error covariance matrix.
It takes a significant amount of time and energy to create these free video tutorials. You can support my efforts in this way:
- You Can also press the Thanks YouTube Dollar button
The webpage accompanying this video is given here:
In this video tutorial and in the accompanying web tutorial, we explain how to derive a recursive least squares method from scratch. The recursive least squares method is a very important method since it serves as the basis of adaptive control, adaptive estimation, Kalman filter, and machine learning algorithms. In this video, we start with the measurement equation, and by formulating the cost function that sums the variances of the estimation error, and by solving this cost function we obtain the recursive least squares gain matrix. We also derive an expression for the propagation of the estimation error covariance matrix.
Комментарии