filmov
tv
DDPS | Machine Learning and Multi-scale Modeling
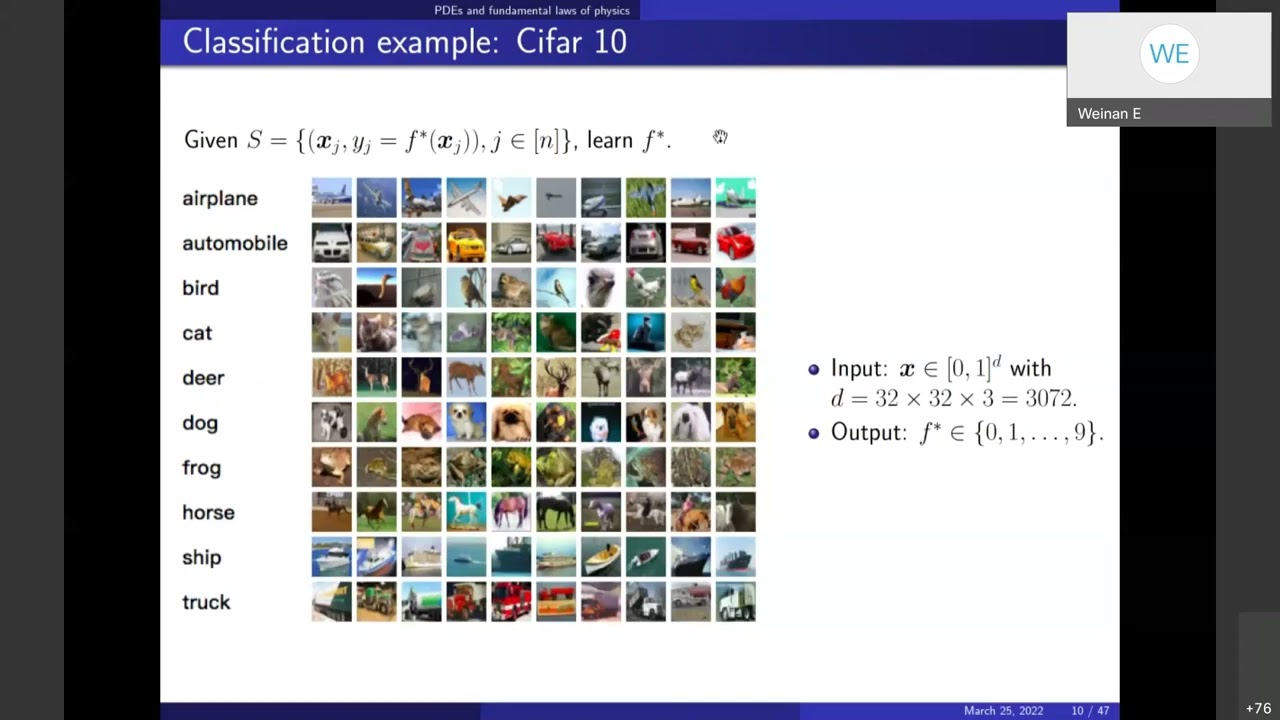
Показать описание
Description: Multi-scale modeling is an ambitious program that aims at unifying the different physical models at different scales for the practical purpose of developing accurate models and simulation protocols for properties of interest. Although the concept of multi-scale modeling is very powerful and very appealing, practical success on really challenging problems has been limited. One key difficulty has been our limited ability to represent complex models and complex functions.
In recent years, machine learning has emerged as a promising tool for overcoming the difficulty of representing complex functions and complex models. In this talk, we will review some of the successes in applying machine learning to multi-scale modeling. These include molecular dynamics, coarse-grained molecular dynamics, non-Newtonian fluid dynamics and model reduction for PDEs.
Bio: Weinan E is a professor in the School of Mathematical Sciences at Peking University and a professor in the Department of Mathematics and Program in Applied and Computational Mathematics at Princeton University. His main research interest is numerical algorithms, machine learning and multi-scale modeling, with applications to chemistry, material sciences and fluid mechanics. In recent years, he has also developed an interest in control theory and related fields.
Weinan E was awarded the ICIAM Collatz Prize in 2003, the SIAM Kleinman Prize in 2009 and the SIAM von Karman Prize in 2014, the SIAM-ETH Peter Henrici Prize in 2019, and the ACM Gordon-Bell Prize in 2020. He is a member of the Chinese Academy of Sciences, a fellow of SIAM, AMS and IOP. Weinan E has been an invited speaker at ICM, ICIAM as well as the AMS National Meeting. He has also been an invited speaker at APS, ACS, AIChe annual meetings and the American Conference of Theoretical Chemistry.
#MachineLearning #MultiScaleModeling #LLNL
LLNL-VIDEO-833537
In recent years, machine learning has emerged as a promising tool for overcoming the difficulty of representing complex functions and complex models. In this talk, we will review some of the successes in applying machine learning to multi-scale modeling. These include molecular dynamics, coarse-grained molecular dynamics, non-Newtonian fluid dynamics and model reduction for PDEs.
Bio: Weinan E is a professor in the School of Mathematical Sciences at Peking University and a professor in the Department of Mathematics and Program in Applied and Computational Mathematics at Princeton University. His main research interest is numerical algorithms, machine learning and multi-scale modeling, with applications to chemistry, material sciences and fluid mechanics. In recent years, he has also developed an interest in control theory and related fields.
Weinan E was awarded the ICIAM Collatz Prize in 2003, the SIAM Kleinman Prize in 2009 and the SIAM von Karman Prize in 2014, the SIAM-ETH Peter Henrici Prize in 2019, and the ACM Gordon-Bell Prize in 2020. He is a member of the Chinese Academy of Sciences, a fellow of SIAM, AMS and IOP. Weinan E has been an invited speaker at ICM, ICIAM as well as the AMS National Meeting. He has also been an invited speaker at APS, ACS, AIChe annual meetings and the American Conference of Theoretical Chemistry.
#MachineLearning #MultiScaleModeling #LLNL
LLNL-VIDEO-833537