filmov
tv
DDPS | Challenges and opportunities for integrating physics-knowledge in machine learning strategies
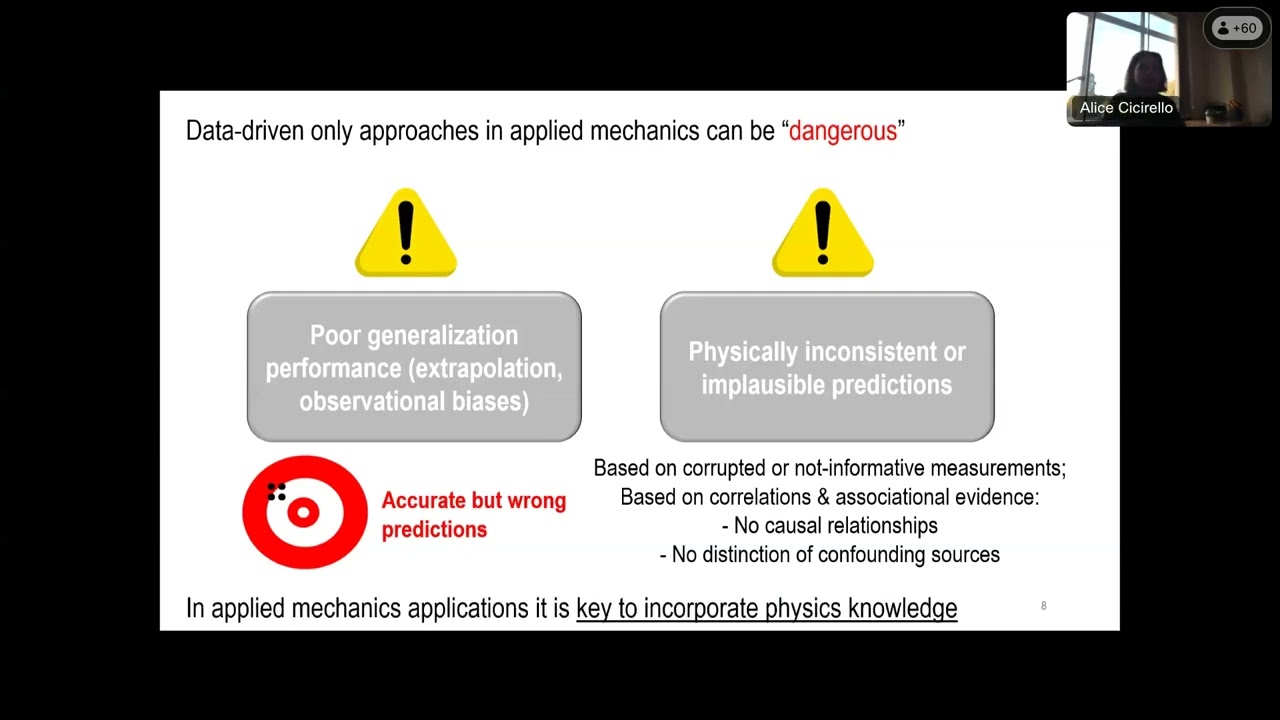
Показать описание
Challenges and opportunities for integrating physics-knowledge in machine learning strategies: friction identification case study
Abstract: Including physics-knowledge in Machine Learning (ML) based algorithms has several advantages, including the ability to generalize in the small-medium data regime and to enforce physics-based knowledge in the presence of large data ensuring physics-consistent predictions. This talk will focus on 4 different biases that can be enforced in a general ML strategy: observational, inductive, learning, and discrepancy. The need to include such biases to tackle an engineering challenge will be discussed and showcased in a case study: the identification of the friction forces in a dynamical system. Three physics-enhanced ML strategies are going to be discussed, two based on sparse system identification and another one based on Gaussian Processes. Numerical and experimental results are going to be presented, showing the applicability of the proposed strategies. Further, open challenges are going to be discussed.
Bio: Dr Alice Cicirello (1984) is the founder and the Head of the Data, Vibration and Uncertainty Group (2017), and currently holds the Alexander von Humboldt Foundation Research Fellowship for Experienced Researchers (2022). From 2020 to 2023, Alice was Associate Professor at TU Delft and Head of the Section of Mechanics and Physics of Structures in the Engineering Structures Department at TU Delft, where she also leads the Monitoring, Vibration and Uncertainty (MVU) laboratory. Recently she moved to University of Cambridge. Alice was a Departmental Lecturer in Dynamics and Vibration at the Oxford Engineering Science Department (Solid Mechanics & Materials Engineering Group) and a Career Development Fellow in Engineering Science at Balliol College (2017-2019). She founded (2017) and led (2017-2021) the Dynamics, Vibration and Uncertainty (DVU) Laboratory in the Oxford Engineering Science Department. Prior to these positions, she was a Senior Research Scientist at Schlumberger (2014-2017), a Research Associate (2012-2014) and a Marie Curie Early Stage Researcher (2009-2012) at the Cambridge University Engineering Department. Alice obtained her PhD from the University of Cambridge in 2013. Her research interests span from fundamental to applied research. Her technical expertise covers Uncertainty Quantification, Machine Learning applied to measurements, text, and physics-based models, advanced physics-based modelling of non-linear systems, Dynamic Experimental Testing and monitoring of components/systems/structures and materials. Alice's research aims at developing effective strategies for guiding, at the design-stage and in operating conditions, decisions making on important functional components, critical structures and complex systems under deep uncertainties and nonlinearity.
Alice has held visiting positions at several research institutions, including MIT, Sandia National Laboratories, the Alan Turing Institute and the University of Auckland. Alice was Honorary Lecturer at the University of Liverpool and an IAS Open Programme Fellow at Institute of Advanced Studies (Loughborough University).
Alice has organized and chaired technical sessions at international conferences, technical workshops and online seminar series. Alice was part of the organizing committee of the 10th International Conference on Modern Practice in Stress and Vibration Analysis (MPSVA 2022) and EURODYN 23, and member of the scientific committee of several conferences (including the Noise and Vibration Engineering Conference (ISMA) and of Uncertainty in Structural Dynamics (USD) conferences, ISMA2022-USD2022, UNCECOMP 21 and 23, and PASC 23). Alice organised and chaired the Physics-Enhancing Machine Learning in Applied Solid Mechanics Workshop (2022).
IM release number: LLNL-VIDEO-855657
Abstract: Including physics-knowledge in Machine Learning (ML) based algorithms has several advantages, including the ability to generalize in the small-medium data regime and to enforce physics-based knowledge in the presence of large data ensuring physics-consistent predictions. This talk will focus on 4 different biases that can be enforced in a general ML strategy: observational, inductive, learning, and discrepancy. The need to include such biases to tackle an engineering challenge will be discussed and showcased in a case study: the identification of the friction forces in a dynamical system. Three physics-enhanced ML strategies are going to be discussed, two based on sparse system identification and another one based on Gaussian Processes. Numerical and experimental results are going to be presented, showing the applicability of the proposed strategies. Further, open challenges are going to be discussed.
Bio: Dr Alice Cicirello (1984) is the founder and the Head of the Data, Vibration and Uncertainty Group (2017), and currently holds the Alexander von Humboldt Foundation Research Fellowship for Experienced Researchers (2022). From 2020 to 2023, Alice was Associate Professor at TU Delft and Head of the Section of Mechanics and Physics of Structures in the Engineering Structures Department at TU Delft, where she also leads the Monitoring, Vibration and Uncertainty (MVU) laboratory. Recently she moved to University of Cambridge. Alice was a Departmental Lecturer in Dynamics and Vibration at the Oxford Engineering Science Department (Solid Mechanics & Materials Engineering Group) and a Career Development Fellow in Engineering Science at Balliol College (2017-2019). She founded (2017) and led (2017-2021) the Dynamics, Vibration and Uncertainty (DVU) Laboratory in the Oxford Engineering Science Department. Prior to these positions, she was a Senior Research Scientist at Schlumberger (2014-2017), a Research Associate (2012-2014) and a Marie Curie Early Stage Researcher (2009-2012) at the Cambridge University Engineering Department. Alice obtained her PhD from the University of Cambridge in 2013. Her research interests span from fundamental to applied research. Her technical expertise covers Uncertainty Quantification, Machine Learning applied to measurements, text, and physics-based models, advanced physics-based modelling of non-linear systems, Dynamic Experimental Testing and monitoring of components/systems/structures and materials. Alice's research aims at developing effective strategies for guiding, at the design-stage and in operating conditions, decisions making on important functional components, critical structures and complex systems under deep uncertainties and nonlinearity.
Alice has held visiting positions at several research institutions, including MIT, Sandia National Laboratories, the Alan Turing Institute and the University of Auckland. Alice was Honorary Lecturer at the University of Liverpool and an IAS Open Programme Fellow at Institute of Advanced Studies (Loughborough University).
Alice has organized and chaired technical sessions at international conferences, technical workshops and online seminar series. Alice was part of the organizing committee of the 10th International Conference on Modern Practice in Stress and Vibration Analysis (MPSVA 2022) and EURODYN 23, and member of the scientific committee of several conferences (including the Noise and Vibration Engineering Conference (ISMA) and of Uncertainty in Structural Dynamics (USD) conferences, ISMA2022-USD2022, UNCECOMP 21 and 23, and PASC 23). Alice organised and chaired the Physics-Enhancing Machine Learning in Applied Solid Mechanics Workshop (2022).
IM release number: LLNL-VIDEO-855657