filmov
tv
DDPS | Towards automatic architecture design for emerging machine learning tasks | Misha Khodak
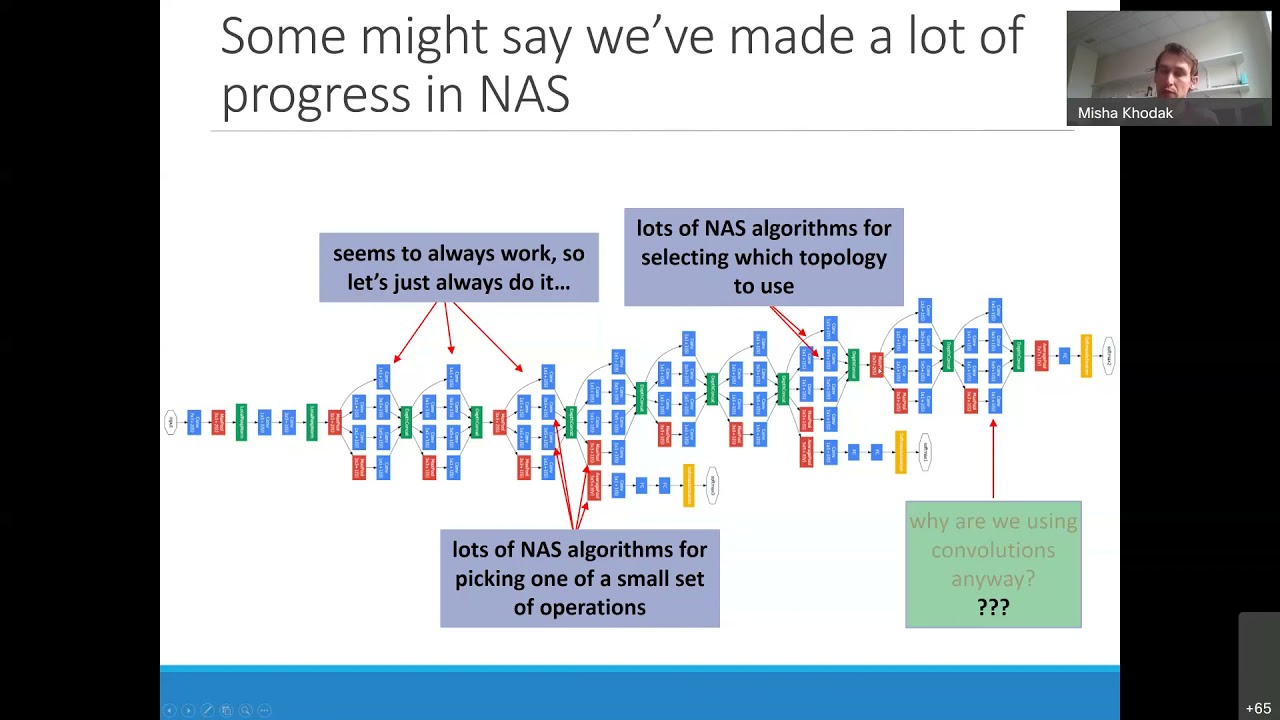
Показать описание
Hand-designed neural networks have played a major role in accelerating progress in traditional areas of machine learning such as computer vision, but designing neural networks for other domains remains a challenge. Successfully transferring existing architectures to applications such as sequence modeling, learning on graphs, or solving partial differential equations has required the manual design of task-specific neural operations to replace the standard convolutions used for classifying images. In this work, we study the problem of automating architecture transfer to enable users to find the right operations to apply neural networks to their specific domain. We introduce a family of neural operations called XD-Operations that mimic the useful degree-of-freedom constraints of convolutions while being much more expressive, provably including several well-known operations. We then demonstrate the effectiveness of XD-operations on the problem of learning to solve partial differential equations given initial conditions, outperforming both standard neural network baselines and the latest hand-designed operations on this task.
Bio: Misha is a PhD student in computer science at Carnegie Mellon University advised by Nina Balcan and Ameet Talwalkar. His research focuses on foundations and applications of machine learning, in particular the theoretical and practical understanding of meta-learning and automation. He is a recipient of the Facebook PhD Fellowship and has spent time as an intern at Microsoft Research - New England, the Lawrence Livermore National Lab, and the Princeton Plasma Physics Lab. Previously, he received an AB in Mathematics and an MSE in Computer Science from Princeton University.
LLNL-VIDEO-828889
#MachineLearning #AutomaticArchitecture #LLNL
Bio: Misha is a PhD student in computer science at Carnegie Mellon University advised by Nina Balcan and Ameet Talwalkar. His research focuses on foundations and applications of machine learning, in particular the theoretical and practical understanding of meta-learning and automation. He is a recipient of the Facebook PhD Fellowship and has spent time as an intern at Microsoft Research - New England, the Lawrence Livermore National Lab, and the Princeton Plasma Physics Lab. Previously, he received an AB in Mathematics and an MSE in Computer Science from Princeton University.
LLNL-VIDEO-828889
#MachineLearning #AutomaticArchitecture #LLNL