filmov
tv
DDPS|Generalizing Scientific Machine Learning and Differentiable Simulation Beyond Continuous models
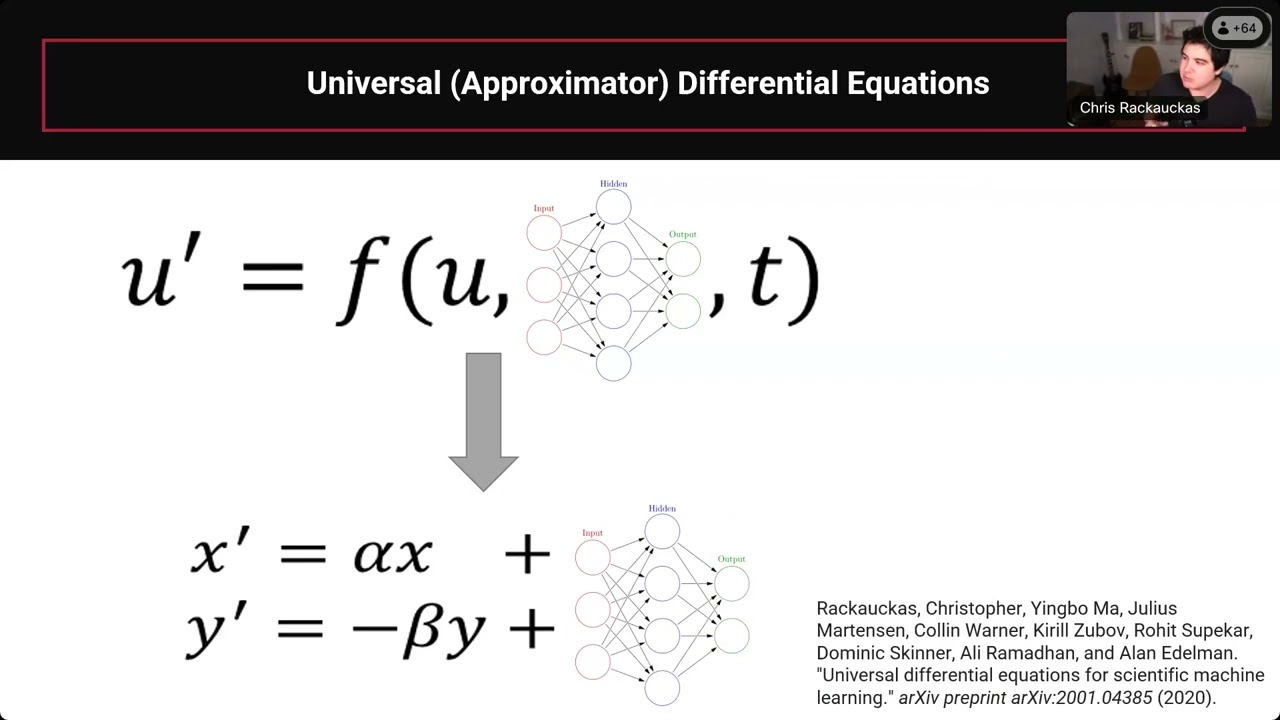
Показать описание
Abstract: The combination of scientific models into deep learning structures, commonly referred to as scientific machine learning (SciML), has made great strides in the last few years in incorporating models such as ODEs and PDEs into deep learning through differentiable simulation. However, the vast space of scientific simulation also includes models like jump diffusions, agent-based models, and more. Is SciML constrained to the simple continuous cases or is there a way to generalize to more advanced model forms? This talk will dive into the mathematical aspects of generalizing differentiable simulation to discuss cases like chaotic simulations, differentiating stochastic simulations like particle filters and agent-based models, and solving inverse problems of Bayesian inverse problems (i.e. differentiation of Markov Chain Monte Carlo methods). We will then discuss the evolving numerical stability issues, implementation issues, and other interesting mathematical tidbits that are coming to light as these differentiable programming capabilities are being adopted.
IM release number: LLNL-VIDEO-856508
📲 Instagram: / livermore_lab
🐤 Twitter: / livermore_lab
🔔 Subscribe: / livermorelab
IM release number: LLNL-VIDEO-856508
📲 Instagram: / livermore_lab
🐤 Twitter: / livermore_lab
🔔 Subscribe: / livermorelab
DDPS|Generalizing Scientific Machine Learning and Differentiable Simulation Beyond Continuous models
DDPS | Scientific Machine Learning through the Lens of Physics-Informed Neural Networks
DDPS | A flexible and generalizable XAI framework for scientific deep learning
DDPS | “Multi-fidelity linear regression for scientific machine learning from scarce data”
DDPS | The problem with deep learning for physics (and how to fix it) by Miles Cranmer
DDPS | Machine Learning and Multi-scale Modeling
DDPS | Model-constrained deep learning approaches for inference, control and UQ
DDPS | The passive symmetries of machine learning by Soledad Villar
DDPS | Machine Learning and Physics-based Simulations – Yin and Yang of Industrial Digit
DDPS | A mathematical understanding of modern Machine Learning: theory, algorithms and applications
DDPS | Reduced Order Modeling and Inverse Design of Flexible Structures by Machine Learning
Scientific Machine Learning: Physics-Informed Neural Networks with Craig Gin
DDPS | Generative Machine Learning Approaches for Data-Driven Modeling and Reductions
DDPS | Interpretable and Generalizable Machine Learning for Fluid Mechanics
DDPS | Physics-Guided Deep Learning for Dynamics Forecasting
DDPS | Non-intrusive reduced order models using physics informed neural networks
DDPS | Incorporating power system physics into deep learning via implicit layers
DDPS | Big Data Inverse Problems — Promoting Sparsity and Learning to Regularize by Mattias Chung
DDPS | Bayesian Optimization: Exploiting Machine Learning Models, Physics, & Throughput Experime...
DIRECT 2021 12 Scientific Machine Learning
DDPS |Scientific Uses of Automatic Differentiation by Michael Brenner
DDPS | Deep neural operators with reliable extrapolation for multiphysics & multiscale problems
DDPS | Neural Differentiable Physics
DDPS | Modeling and controlling turbulent flows through deep learning
Комментарии