filmov
tv
Lecture 2: Image Classification
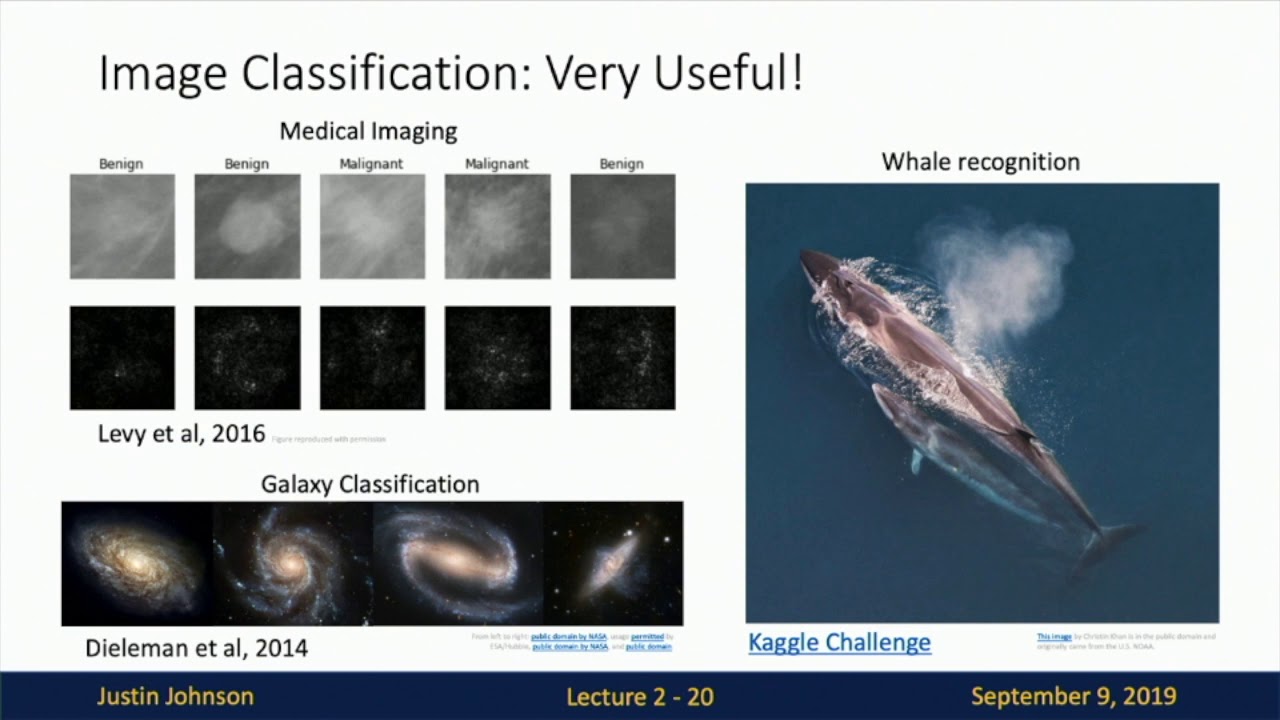
Показать описание
Lecture 2 introduces image classification as a core computer vision problem. We see that the image classification task is made challenging by the semantic gap, but that solutions to this task can be used as a building block in other more complicated computer vision systems. We introduce machine learning as a data-driven approach to solving hard problems like image classification. We discuss several common classification datasets in computer vision. Finally we introduce K-Nearest Neighbors (KNN) as our first machine learning algorithm. This leads to a discussion of hyperparameters and cross-validation strategies that will be crucial for all the machine learning algorithms we will later use.
_________________________________________________________________________________________________
Computer Vision has become ubiquitous in our society, with applications in search, image understanding, apps, mapping, medicine, drones, and self-driving cars. Core to many of these applications are visual recognition tasks such as image classification and object detection. Recent developments in neural network approaches have greatly advanced the performance of these state-of-the-art visual recognition systems. This course is a deep dive into details of neural-network based deep learning methods for computer vision. During this course, students will learn to implement, train and debug their own neural networks and gain a detailed understanding of cutting-edge research in computer vision. We will cover learning algorithms, neural network architectures, and practical engineering tricks for training and fine-tuning networks for visual recognition tasks.
_________________________________________________________________________________________________
Computer Vision has become ubiquitous in our society, with applications in search, image understanding, apps, mapping, medicine, drones, and self-driving cars. Core to many of these applications are visual recognition tasks such as image classification and object detection. Recent developments in neural network approaches have greatly advanced the performance of these state-of-the-art visual recognition systems. This course is a deep dive into details of neural-network based deep learning methods for computer vision. During this course, students will learn to implement, train and debug their own neural networks and gain a detailed understanding of cutting-edge research in computer vision. We will cover learning algorithms, neural network architectures, and practical engineering tricks for training and fine-tuning networks for visual recognition tasks.
Комментарии