filmov
tv
Faster LLM Inference: Speeding up Falcon 7b (with QLoRA adapter) Prediction Time
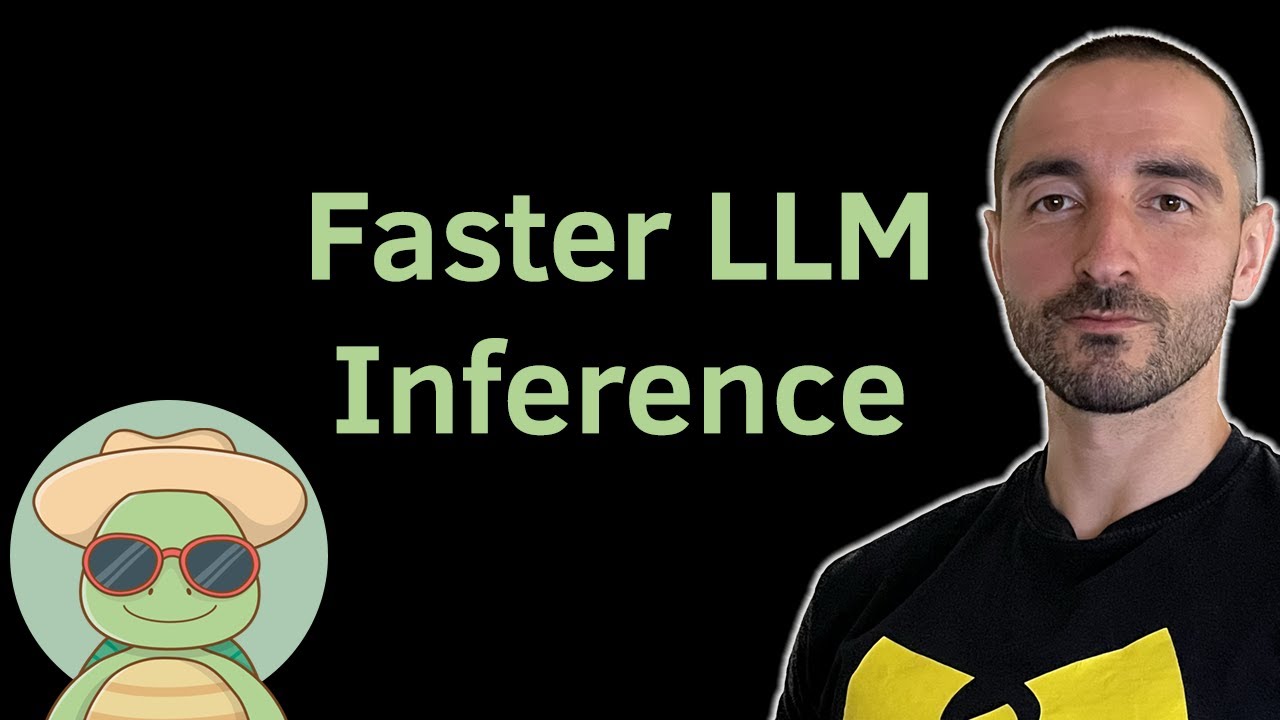
Показать описание
How can you speed up your LLM inference time?
In this video, we'll optimize the token generation time for our fine-tuned Falcon 7b model with QLoRA. We'll explore various model loading techniques and look into batch inference for faster predictions.
00:00 - Introduction
01:26 - Google Colab Setup
03:58 - Training Config Baseline
07:06 - Loading in 4 Bit
08:26 - Loading in 8 Bit
10:25 - Batch Inference
12:00 - Lit-Parrot
16:57 - Conclusion
Turtle image by stockgiu
#chatgpt #gpt4 #llms #artificialintelligence #promptengineering #chatbot #transformers #python #pytorch
In this video, we'll optimize the token generation time for our fine-tuned Falcon 7b model with QLoRA. We'll explore various model loading techniques and look into batch inference for faster predictions.
00:00 - Introduction
01:26 - Google Colab Setup
03:58 - Training Config Baseline
07:06 - Loading in 4 Bit
08:26 - Loading in 8 Bit
10:25 - Batch Inference
12:00 - Lit-Parrot
16:57 - Conclusion
Turtle image by stockgiu
#chatgpt #gpt4 #llms #artificialintelligence #promptengineering #chatbot #transformers #python #pytorch
Faster LLM Inference: Speeding up Falcon 7b (with QLoRA adapter) Prediction Time
Faster LLM Inference: Speeding up Falcon 7b For CODE: FalCODER 🦅👩💻
StreamingLLM - Extend Llama2 to 4 million token & 22x faster inference?
Speeding Up AI: Speculative Streaming for Fast LLM Inference
PowerInfer: 11x Faster than Llama.cpp for LLM Inference 🔥
LLMLingua: Speed up LLM's Inference and Enhance Performance up to 20x!
EAGLE: the fastest speculative sampling method speed up LLM inference 3 times! #llm #ai#inference
Accelerate Big Model Inference: How Does it Work?
Optimizing vLLM Performance through Quantization | Ray Summit 2024
Five Technique : How To Speed Your Local LLM Chatbot Performance - Here The Result
3090 vs 4090 Local AI Server LLM Inference Speed Comparison on Ollama
Llama2.mojo🔥: The Fastest Llama2 Inference ever on CPU
Exploring the Latency/Throughput & Cost Space for LLM Inference // Timothée Lacroix // CTO Mist...
vLLM - Turbo Charge your LLM Inference
Accelerating LLM Inference with vLLM
Accelerate Transformer inference on GPU with Optimum and Better Transformer
Herbie Bradley – EleutherAI – Speeding up inference of LLMs with Triton and FasterTransformer
Effort Engine: Speeding up LLM Inference 2x with Dynamic Weight Selection | AI News
Understanding LLM Inference | NVIDIA Experts Deconstruct How AI Works
LLaMA 3 “Hyper Speed” is INSANE! (Best Version Yet)
Go Production: ⚡️ Super FAST LLM (API) Serving with vLLM !!!
Mixtral 8X7B Crazy Fast Inference Speed
Fast LLM Serving with vLLM and PagedAttention
Build an API for LLM Inference using Rust: Super Fast on CPU
Комментарии