filmov
tv
Exploring the Latency/Throughput & Cost Space for LLM Inference // Timothée Lacroix // CTO Mistral
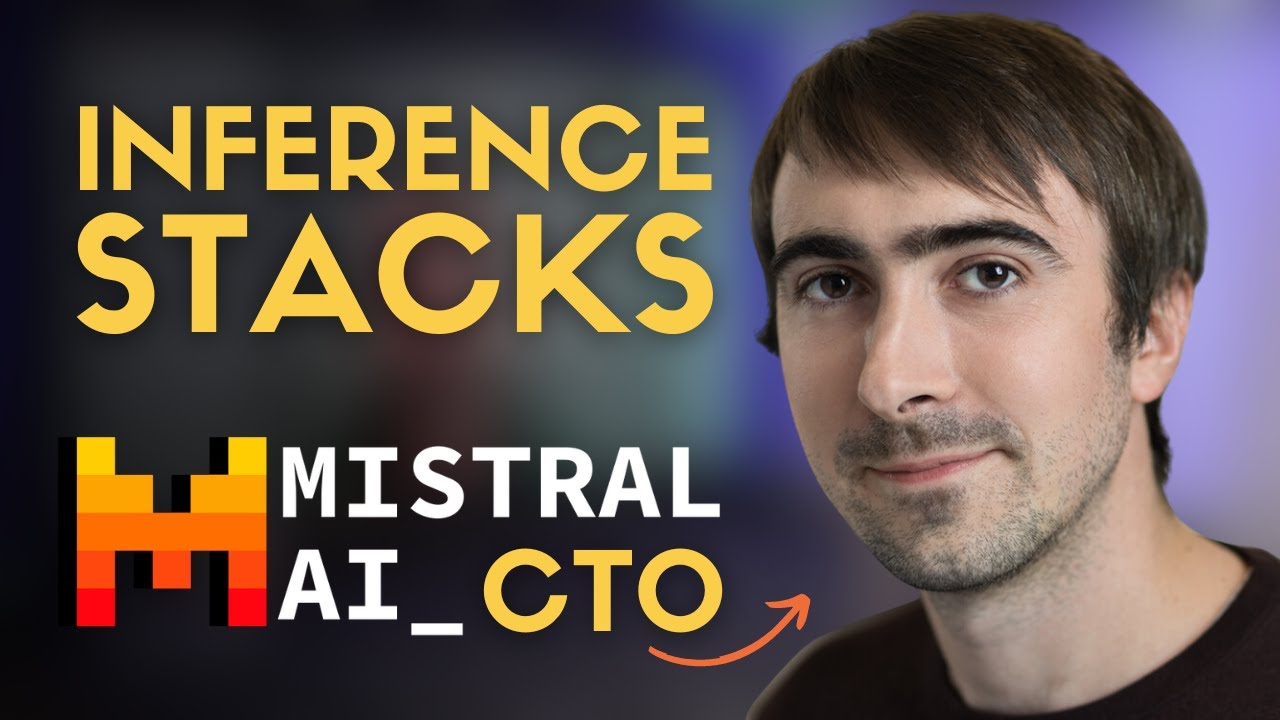
Показать описание
// Abstract
Getting the right LLM inference stack means choosing the right model for your task, and running it on the right hardware, with proper inference code. This talk will go through popular inference stacks and set-ups, detailing what makes inference costly. We'll talk about the current generation of open-source models and how to make the best use of them, but we will also touch on features currently missing from the open-source serving stack as well as what the future generations of models will unlock.
// Bio
Timothée Lacroix, aged 31, is Chief Technical Officer in charge of technical issues relating to product efficacy and research. Started as an engineer at Facebook AI Research in 2015 in New York, where he completed his thesis between 2016 and 2019, in collaboration with École des Ponts, on tensor factorization for recommender systems. He continued his career at Meta until 2023 when he co-founded @Mistral-AI.
// Sign up for our Newsletter to never miss an event:
// Watch all the conference videos here:
// Read our blog:
// Join an in-person local meetup near you:
// MLOps Swag/Merch:
// Follow us on Twitter:
//Follow us on Linkedin:
Комментарии