filmov
tv
Orca 2 🐳 GIANT Breakthrough For AI Logic/Reasoning
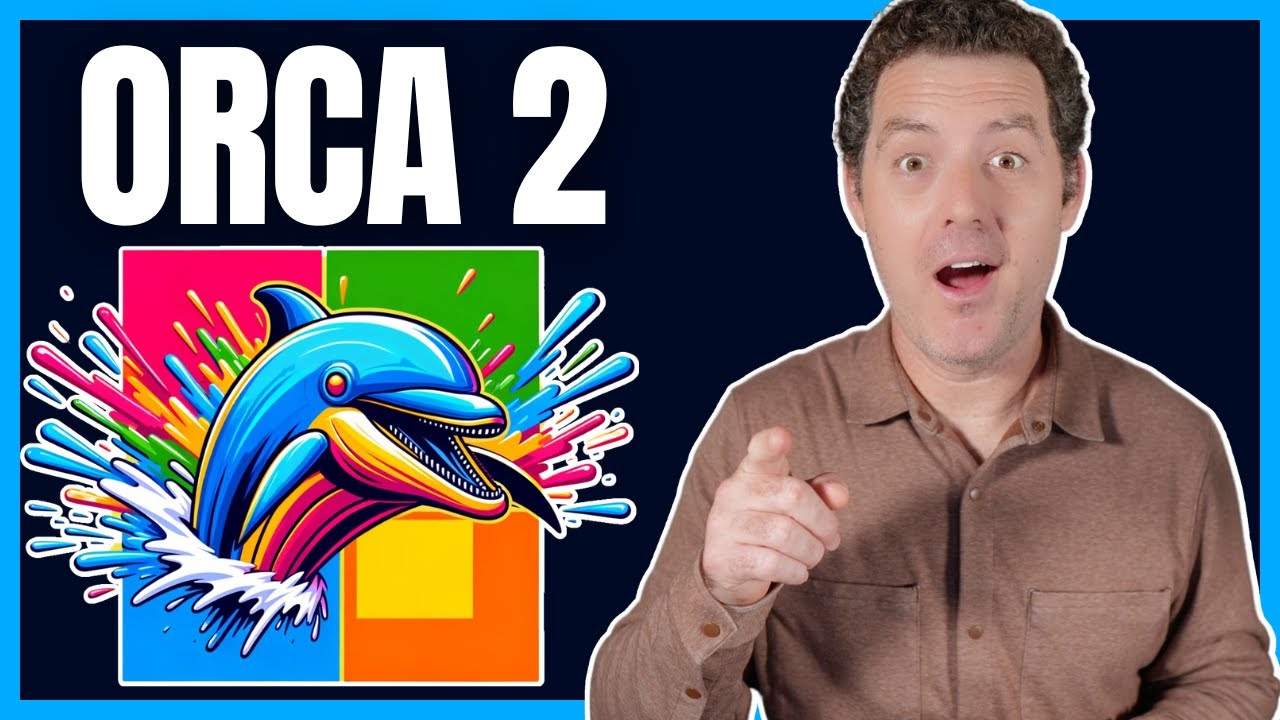
Показать описание
Orca 2 shows how smaller models can be trained to think through problems and achieve "slow thinking." This is a giant leap forward from Orca 1 and allows 13b models to perform as well as models that are 5-6x larger at logic and reasoning tasks.
Update: I made a mistake with the shirts drying problem, Orca 2 actually got it wrong :(
Enjoy :)
Join My Newsletter for Regular AI Updates 👇🏼
My Links 🔗
Media/Sponsorship Inquiries 📈
Links:
Update: I made a mistake with the shirts drying problem, Orca 2 actually got it wrong :(
Enjoy :)
Join My Newsletter for Regular AI Updates 👇🏼
My Links 🔗
Media/Sponsorship Inquiries 📈
Links:
Orca 2 🐳 GIANT Breakthrough For AI Logic/Reasoning
Smaller, Smarter, Stronger: Orca 2 Challenges GPT-4's Dominance
Discover the Secrets of Microsoft Ai Orca 2
Microsoft's ORCA 2: New AI Genius Beyond GPT-4 and GPT-4 Turbo |Microsoft ORCA AI
How ORCA 2, Microsoft's NEW AI Genius Is Better Than GPT4
AI Evolution Microsoft Orca 2 Surpasses OpenAi ChatGPT4
Microsoft's ORCA 2: New AI Outperforms GPT-4 in Intricate Tasks!
DARPA’s “Manta Ray” Program, unmanned underwater vehicles(UUV) Build and Testing Phase-II
Orca: The Model Few Saw Coming
Scientists discover 100 new maritime species off New Zealand | DW News
Microsoft's New AI 'ORCA' INSANE GPT Rival
NEW 'Orca' 🐳 Model 'Free Willy' Has Surprising Results
Orca Got Stuck and Cried for Hours. When Rescuers Arrived, Something Incredible Happens
Breakthrough: Run Massive Models On Any Device (ex: LLaMA 65b)
Microsoft’s Orca SHOCKS the entire industry - STUNNING GPT 4 competitor
Scientists Discovered A Lost Ancient City Hidden Underwater That Shouldn't Exist
The Top 40 Epic Military Vehicles Across The Globe
Marine Biologist Breaks Down Shark Attack Scenes from Movies | GQ
Most Wild Moments from Shark Week: Belly of the Beast: Feeding Frenzy 2023 | Discovery
$125B for Superintelligence? 3 Models Coming, Sutskever's Secret SSI, & Data Centers (in s...
LEGO Sharks!
Hear what pilots said about mysterious object shot down near Alaska
A little about BATTLETECH - Omega, the 1st Viable Superheavy
Elephants Being Elephants | BBC Earth
Комментарии