filmov
tv
Markov Chains : Data Science Basics
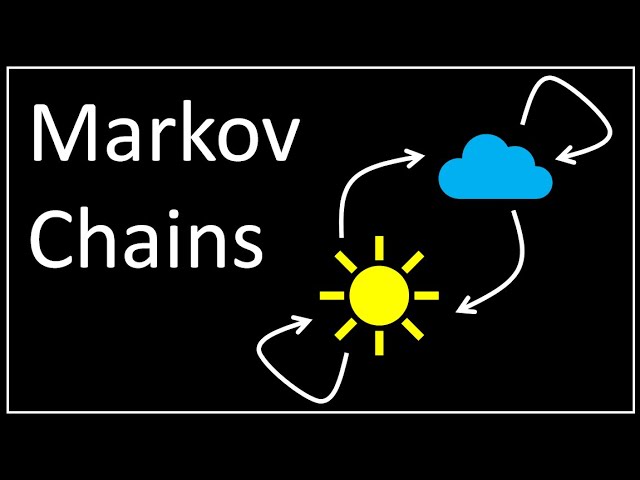
Показать описание
The basics of Markov Chains, one of my ALL TIME FAVORITE objects in data science.
Introducing Markov Chains
Markov Chains : Data Science Basics
Markov Chains Clearly Explained! Part - 1
Markov Chain Monte Carlo (MCMC) : Data Science Concepts
What is Markov Chain - Machine Learning & Data Science Terminologies
Intro to Markov Chains & Transition Diagrams
The Best Markov Chain Explanation
Hidden Markov Model : Data Science Concepts
Avik Das: Dynamics Programming for Machine Learning- Hidden Markov Models | PyData LA 2019
Niloy Biswas - Estimating Convergence of Markov Chains with L-Lag Couplings
GerryChain.jl: detecting gerrymandering with Markov chains | P J Rule, B Suwal, M Sun | JuliaCon2021
Markov Chains: n-step Transition Matrix | Part - 3
Origin of Markov chains | Journey into information theory | Computer Science | Khan Academy
Markov Chains & Transition Matrices
Statistical Methods Applied Maths Data Science: Simulate Discrete-time Markov Chain | packtpub.com
10.1 Markov chains
Stateful Structure Streaming and Markov Chains Join Forces to Monitor the Biggest Storage of Physics
Intro to Markov Chains and Bayesian Inference | Mackenzie Simper
Markov Chain Stationary Distribution : Data Science Concepts
Markov Chain Example - How to use Markov Chains in Natural Language Generation
Markov Chains: Generating Sherlock Holmes Stories | Part - 4
Hidden Markov Chains
Estimating the Mixing Time of Ergodic Markov Chains
Introduction To Markov Chains | Markov Chains in Python | Edureka
Комментарии