filmov
tv
Easy way to compute Jacobian and gradient with forward and back propagation in graph
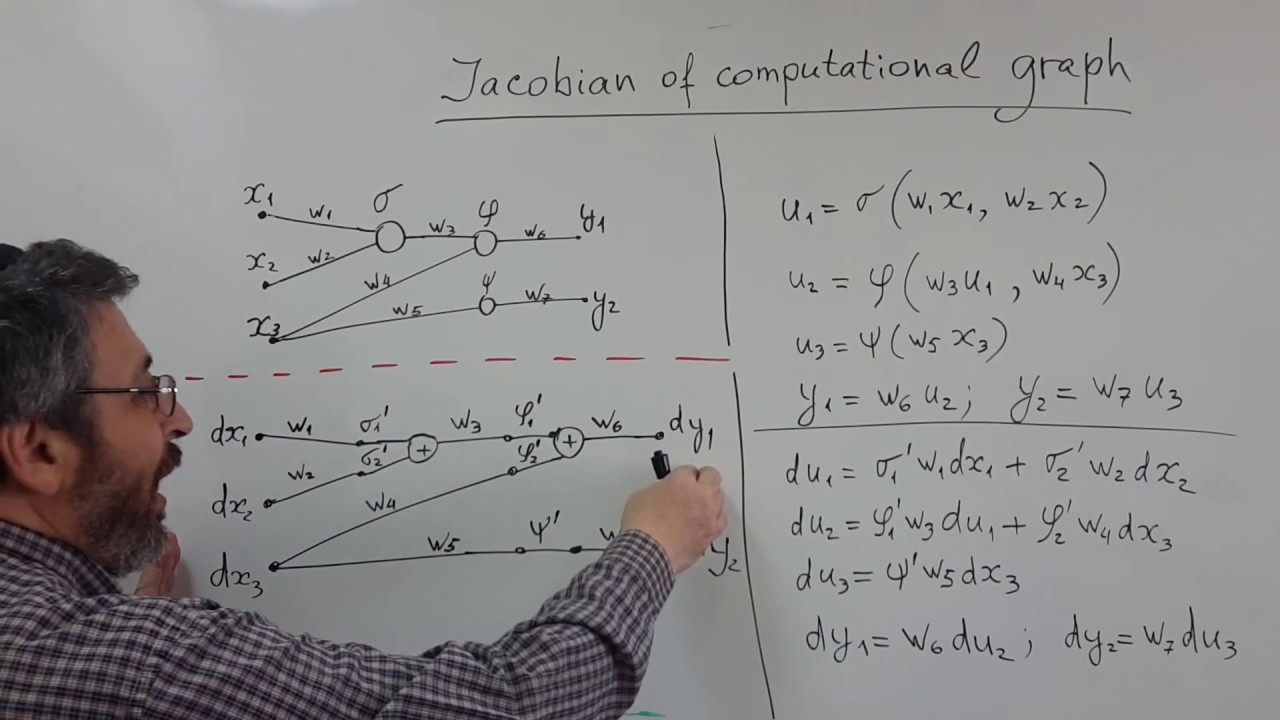
Показать описание
Before watching, you can refresh the notion of the differential with my other video:
Lecture 2-3: Gradient and Hessian of Multivariate Function
Help us caption & translate this video!
Lecture 2-3: Gradient and Hessian of Multivariate Function
Help us caption & translate this video!
Easy way to compute Jacobian and gradient with forward and back propagation in graph
What is Jacobian? | The right way of thinking derivatives and integrals
The Jacobian
Jacobian| jacobian transformation|differential calculus
Computing a Jacobian matrix
Change of Variables and the Jacobian
How to find Jacobian Matrix? | Solved Examples | Robotics 101
Change of Variables & The Jacobian | Multi-variable Integration
parameterization of surface in calculus - 3D Jacobian d(x, y, z) /d(u, v, w) part 8
The Jacobian matrix
How to Compute the Jacobian of a Robot Manipulator: A Numerical Example | Robotic Systems
The Jacobian Matrix
Linear Algebra: Jacobian Determinant
Jacobin theorem(engineering mathematics)
Oxford Calculus: Jacobians Explained
How REAL Men Integrate Functions
Double Integral through a Change of Coordinates (the Jacobian)
Example -- Forward Kinematics and Jacobian Matrix
Jacobian - how it works
5 simple unsolvable equations
Calculus: How to Find the Jacobian of the Transformation. [HD]
Robotics 2 U1 (Kinematics) S3 (Jacobian Matrix) P2 (Finding the Jacobian)
#jacobian
Jacobian of the transformation (2x2) (KristaKingMath)
Комментарии