filmov
tv
MIT EI seminar, Hyung Won Chung from OpenAI. 'Don't teach. Incentivize.'
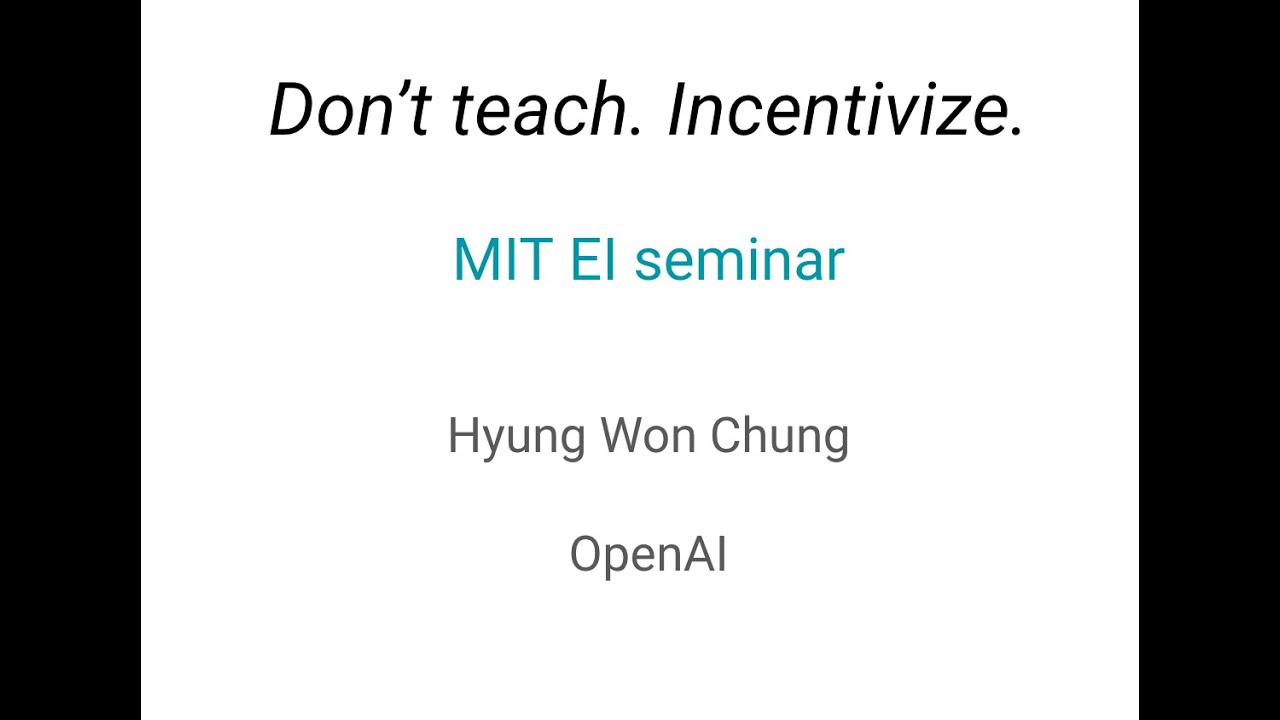
Показать описание
I made this talk last year, when I was thinking about a paradigm shift. This delayed posting is timely as we just released o1, which I believe is a new paradigm. It's a good time to zoom out for high level thinking
I titled the talk “Don’t teach. Incentivize”. We can’t enumerate every single skill we want from an AGI system because there are just too many of them. In my view, the only feasible way is to incentivize the model such that general skills emerge.
I use next token prediction as a running example, interpreting it as a weak incentive structure. It is a massive multitask learning that incentivizes the model to learn a smaller number of general skills to solve trillions of tasks, as opposed to dealing with them individually
If you try to solve tens of tasks with minimal effort possible, then pattern-recognizing each task separately might be easiest.
If you try to solve trillions of tasks, it might be easier to solve them by learning generalizable skills, e.g. language understanding, reasoning.
An analogy I used is extending the old saying: "Give a man a fish, you feed him for a day. Teach him how to fish, you feed him for a lifetime." I go one step further and solve this task with an incentive-based method: "Teach him the taste of fish and make him hungry."
Then he will go out to learn to fish. In doing so, he will learn other skills, such as being patient, learning to read weather, learn about the fish, etc. Some of these skills are general and can be applied to other tasks.
You might think that it takes too long to teach via the incentive instead of direct teaching. That is true for humans, but for machines, we can give more compute to shorten the time. In fact, I'd say this "slower" method allows us to put in more compute.
This has interesting implications for generalist vs specialist tradeoff. Such tradeoff exist for humans because time spent on specializing a topic is time not spent on generalizing. For machines, that doesn’t apply. Some models get to enjoy 10000x more compute.
Another analogy is “Room of spirit and time” from Dragon ball. You train one year inside the room and it is only a day outside. The multiplier is 365. For machines it is a lot higher. So a strong generalist with more compute is often better at special domains than specialists.
I hope this lecture sparks interest in high level thinking, which will be useful in building better perspectives. This in turn will lead to finding more impactful problems to solve.
I titled the talk “Don’t teach. Incentivize”. We can’t enumerate every single skill we want from an AGI system because there are just too many of them. In my view, the only feasible way is to incentivize the model such that general skills emerge.
I use next token prediction as a running example, interpreting it as a weak incentive structure. It is a massive multitask learning that incentivizes the model to learn a smaller number of general skills to solve trillions of tasks, as opposed to dealing with them individually
If you try to solve tens of tasks with minimal effort possible, then pattern-recognizing each task separately might be easiest.
If you try to solve trillions of tasks, it might be easier to solve them by learning generalizable skills, e.g. language understanding, reasoning.
An analogy I used is extending the old saying: "Give a man a fish, you feed him for a day. Teach him how to fish, you feed him for a lifetime." I go one step further and solve this task with an incentive-based method: "Teach him the taste of fish and make him hungry."
Then he will go out to learn to fish. In doing so, he will learn other skills, such as being patient, learning to read weather, learn about the fish, etc. Some of these skills are general and can be applied to other tasks.
You might think that it takes too long to teach via the incentive instead of direct teaching. That is true for humans, but for machines, we can give more compute to shorten the time. In fact, I'd say this "slower" method allows us to put in more compute.
This has interesting implications for generalist vs specialist tradeoff. Such tradeoff exist for humans because time spent on specializing a topic is time not spent on generalizing. For machines, that doesn’t apply. Some models get to enjoy 10000x more compute.
Another analogy is “Room of spirit and time” from Dragon ball. You train one year inside the room and it is only a day outside. The multiplier is 365. For machines it is a lot higher. So a strong generalist with more compute is often better at special domains than specialists.
I hope this lecture sparks interest in high level thinking, which will be useful in building better perspectives. This in turn will lead to finding more impactful problems to solve.
Комментарии