filmov
tv
A Systematic Understanding of Exempt and Non-Exempt Algorithmic Biases (Sanghamitra Dutta,CMU)
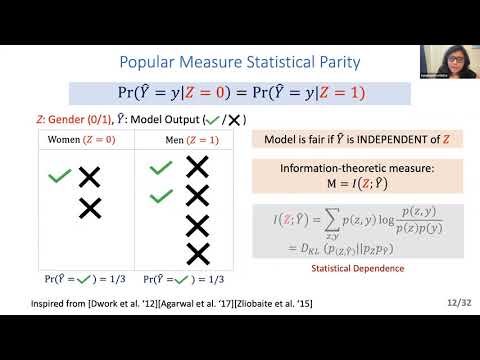
Показать описание
Abstract: With the growing use of machine learning algorithms in highly consequential domains, the quantification and removal of bias with respect to gender, race, etc., is becoming increasingly important. While quantifying bias is essential, sometimes the needs of a business (e.g., hiring) may require the use of certain features that are critical in a way that any bias that can be explained by them might need to be exempted (inspired from the business necessity defense of Title VII of Civil Rights Act). For instance, in hiring a software engineer, a standardized coding-test score may be a critical feature that is weighed strongly in the decision even if it introduces bias, whereas other features, such as name, zip code, or reference letters may be used to improve decision-making, but only to the extent that they do not introduce bias. In this work, we propose a novel information-theoretic measure of non-exempt bias, which quantifies the part of the bias that cannot be accounted for by the critical features. This measure can be applied for (i) Auditing trained models to check if the bias arose purely due to the critical features; and also for (ii) Training with selective removal of the non-exempt bias if desired. We arrive at this decomposition through canonical examples that lead to a set of desirable properties (axioms) that any measure of non-exempt bias should satisfy. We then propose a causal measure of non-exempt bias that satisfies all of them. We also propose observational measures that only satisfy some of these properties (including an impossibility result on observational measures being able to satisfy all properties). Then, we perform case studies using them to show how one can train models while reducing non-exempt bias. Our quantification bridges ideas of causality, Simpson's paradox, and a body of work from information theory called Partial Information Decomposition (PID). The talk will be fairly accessible, no knowledge of information theory is required.
Bio: Sanghamitra Dutta (B. Tech. IIT Kharagpur) is a doctoral candidate in the Department of Electrical and Computer Engineering at Carnegie Mellon University, PA, USA. Her research interests revolve around machine learning and information theory. She is currently focussed on addressing the emerging trust issues in machine learning concerning fairness, privacy and reliability. Her work bridges the fields of information theory, causality, reliability and machine learning. In her prior work, she has also examined problems in reliable computing, proposing novel algorithmic solutions for large-scale machine-learning in the presence of faults and failures, using tools from coding theory (an emerging area called “coded computing”). Her results on coded computing address problems that have been open for several decades and have received substantial attention from across communities. She is a recipient of the 2019 K&L Gates Presidential Fellowship, 2019 Axel Berny Presidential Graduate Fellowship, 2017 Tan Endowed Graduate Fellowship, 2016 Prabhu and Poonam Goel Graduate Fellowship, and the 2014 HONDA Young Engineer and Scientist Award.
Bio: Sanghamitra Dutta (B. Tech. IIT Kharagpur) is a doctoral candidate in the Department of Electrical and Computer Engineering at Carnegie Mellon University, PA, USA. Her research interests revolve around machine learning and information theory. She is currently focussed on addressing the emerging trust issues in machine learning concerning fairness, privacy and reliability. Her work bridges the fields of information theory, causality, reliability and machine learning. In her prior work, she has also examined problems in reliable computing, proposing novel algorithmic solutions for large-scale machine-learning in the presence of faults and failures, using tools from coding theory (an emerging area called “coded computing”). Her results on coded computing address problems that have been open for several decades and have received substantial attention from across communities. She is a recipient of the 2019 K&L Gates Presidential Fellowship, 2019 Axel Berny Presidential Graduate Fellowship, 2017 Tan Endowed Graduate Fellowship, 2016 Prabhu and Poonam Goel Graduate Fellowship, and the 2014 HONDA Young Engineer and Scientist Award.