filmov
tv
[ACC 2022] Robust Model Predictive Control with Data-Driven Koopman Operators
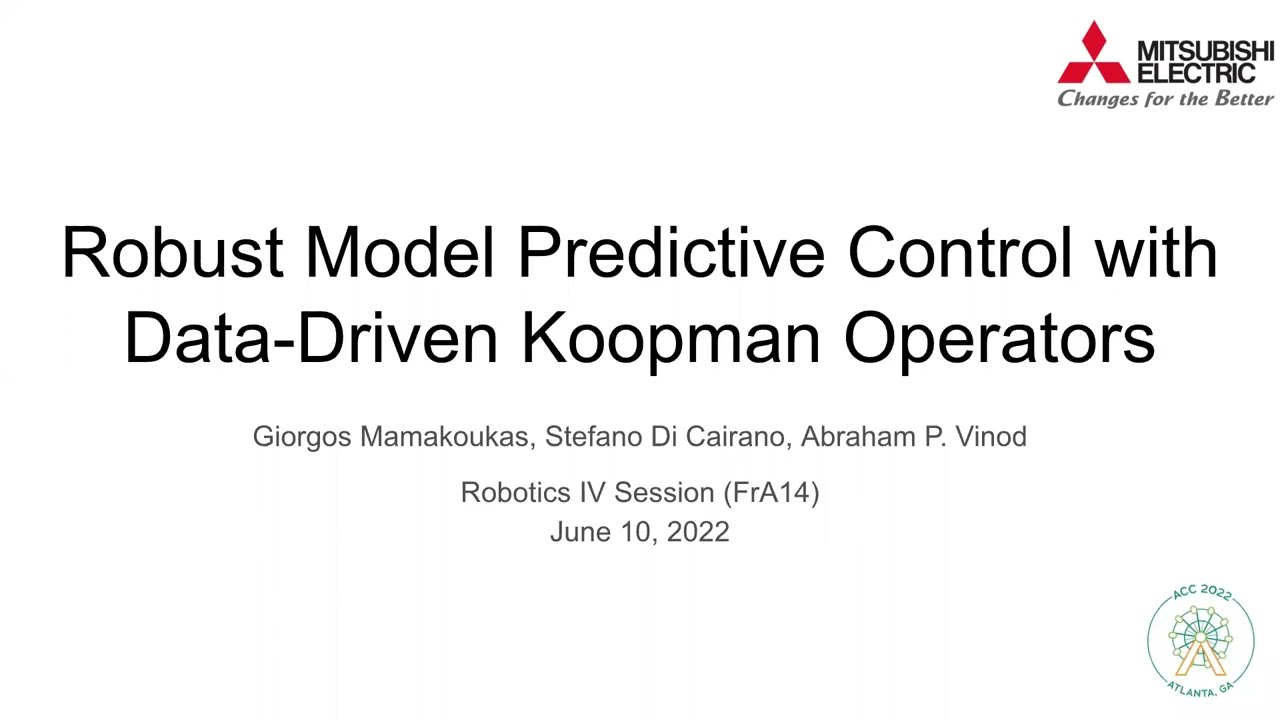
Показать описание
MERL Intern Giorgos Mamakoukas presents his paper titled "Robust Model Predictive Control with Data-Driven Koopman Operators" for the American Control Conference (ACC) 2022, held at Atlanta GA. The paper was co-authored with MERL researchers Stefano Di Cairano and Abraham P. Vinod.
Abstract: We present robust Koopman model predictive control (robust KMPC), a methodology that leverages the training performance of data-driven Koopman models to improve constraint satisfaction over nominal KMPC. Existing Koopman MPC approaches ignore the modeling error during control, which can lead to high constraint violations. The proposed approach significantly reduces such violations by combining Lipschitz analysis with convex optimization. Specifically, for Lipschitz continuous, unknown dynamics, we derive data-driven, state-dependent bounds for the modeling error. Next, we robustify the finite-horizon, optimal control problem using the obtained state-dependent modeling error bounds. Finally, we convexify the optimization problem to obtain a real-time implementable MPC framework. We demonstrate the efficacy of our approach with simulation results using the dynamics of a forced Duffing oscillator and a quadrotor. Our approach significantly outperforms the existing approaches in low-data regime.
Abstract: We present robust Koopman model predictive control (robust KMPC), a methodology that leverages the training performance of data-driven Koopman models to improve constraint satisfaction over nominal KMPC. Existing Koopman MPC approaches ignore the modeling error during control, which can lead to high constraint violations. The proposed approach significantly reduces such violations by combining Lipschitz analysis with convex optimization. Specifically, for Lipschitz continuous, unknown dynamics, we derive data-driven, state-dependent bounds for the modeling error. Next, we robustify the finite-horizon, optimal control problem using the obtained state-dependent modeling error bounds. Finally, we convexify the optimization problem to obtain a real-time implementable MPC framework. We demonstrate the efficacy of our approach with simulation results using the dynamics of a forced Duffing oscillator and a quadrotor. Our approach significantly outperforms the existing approaches in low-data regime.