filmov
tv
Deep Recurrent Models for Nonlinear Model Predictive Control in Deformable Manipulation Tasks
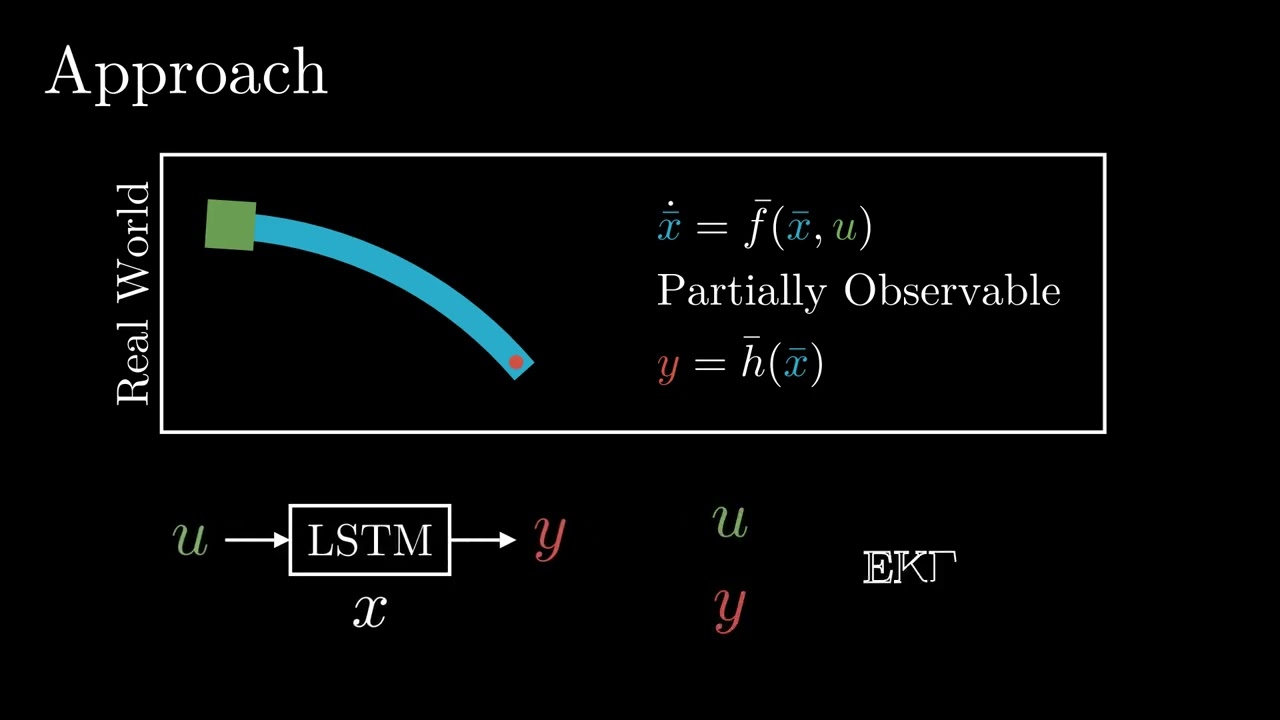
Показать описание
Spotlight talk at 2nd Workshop on Representing and Manipulating Deformable Objects @ ICRA 2022
Title: Deep Recurrent Models for Nonlinear Model Predictive Control in Deformable Manipulation Tasks
Abstract: We propose a method for robotic control of deformable objects using a learned nonlinear dynamics model. After collecting a dataset of trajectories from the real system, we train a recurrent neural network (RNN) to approximate its input-output behavior with a latent state-space model. The RNN internal state is low-dimensional enough to enable realtime nonlinear control methods. We demonstrate a closed- loop control scheme with the RNN model using a standard nonlinear state observer and model-predictive controller. We apply our method to track a highly dynamic trajectory with a point on the deformable object, in real time and on real hardware. Our experiments show that the RNN model captures the true system’s dynamics and can be used to track trajectories outside the training distribution. In an ablation study, we find that the full method improves tracking accuracy compared to an open-loop version without the state observer.
Title: Deep Recurrent Models for Nonlinear Model Predictive Control in Deformable Manipulation Tasks
Abstract: We propose a method for robotic control of deformable objects using a learned nonlinear dynamics model. After collecting a dataset of trajectories from the real system, we train a recurrent neural network (RNN) to approximate its input-output behavior with a latent state-space model. The RNN internal state is low-dimensional enough to enable realtime nonlinear control methods. We demonstrate a closed- loop control scheme with the RNN model using a standard nonlinear state observer and model-predictive controller. We apply our method to track a highly dynamic trajectory with a point on the deformable object, in real time and on real hardware. Our experiments show that the RNN model captures the true system’s dynamics and can be used to track trajectories outside the training distribution. In an ablation study, we find that the full method improves tracking accuracy compared to an open-loop version without the state observer.