filmov
tv
Full Python Tutorial: Customer Lifetime Value & RFM Analysis using Machine Learning
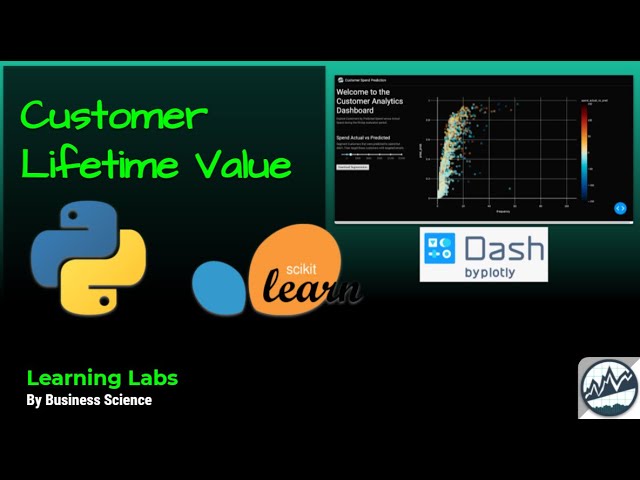
Показать описание
This is a full python tutorial where we analyze customer purchase behavior to predict their purchases over the next 90-days. This allows us to target customers to prevent churn and increase profitability. We use:
- Pandas to create Recency-Frequency-Monetary (RFM) Features
- Scikit-Learn XGBoost to create 2 predictive models (90-day spend amount and spend probability)
- Plotly Dash to productionalize a solution for Marketing Teams
WANT THE CODE?
Table of Contents
00:00 Customer Lifetime Value with Machine Learning
01:06 Project Workflow: Pandas, Scikit Learn, Plotly Dash
04:47 Plotly Dash Demo: Customer Spend Prediction App
08:08 Business Problem: Non-Contractual Purchase Relationship | CDNOW Customer Transactions
10:47 The 3 Questions We'll Answer Today
14:07 Customer Lifetime Value Modeling: Econometric Approach (Cashflow)
16:44 Customer Lifetime Value: Machine Learning Approach
20:47 Full Code Tutorial | Customer Lifetime Value with ML in Python
20:57 Project Setup: VSCode
25:44 Customer Lifetime Value Analysis
27:46 Data Preparation
30:08 Cohort Analysis
36:53 Machine Learning Feature Engineering
37:55 Time Splitting
40:03 Feature Engineering (RFM)
41:29 Recency (R) Features
44:29 Frequency (F) Features
45:31 Monetary (M) Features
46:21 Combine RFM Features
48:51 Machine Learning CLV Analysis: Spend Amount Model
54:09 Machine Learning CLV Analysis: Spend Probability Model
57:28 Feature Importance: Spend Amount Model
59:21 Feature Importance: Spend Probability Model
1:00:52 Save Work
1:01:42 Dash App
1:04:26 How Can We Use this Information? (3 Questions)
1:06:51 Next Steps: Learning More
1:08:50 Dash App Files
1:10:00 Python Track Roadmap
1:22:12 Q&A
- Pandas to create Recency-Frequency-Monetary (RFM) Features
- Scikit-Learn XGBoost to create 2 predictive models (90-day spend amount and spend probability)
- Plotly Dash to productionalize a solution for Marketing Teams
WANT THE CODE?
Table of Contents
00:00 Customer Lifetime Value with Machine Learning
01:06 Project Workflow: Pandas, Scikit Learn, Plotly Dash
04:47 Plotly Dash Demo: Customer Spend Prediction App
08:08 Business Problem: Non-Contractual Purchase Relationship | CDNOW Customer Transactions
10:47 The 3 Questions We'll Answer Today
14:07 Customer Lifetime Value Modeling: Econometric Approach (Cashflow)
16:44 Customer Lifetime Value: Machine Learning Approach
20:47 Full Code Tutorial | Customer Lifetime Value with ML in Python
20:57 Project Setup: VSCode
25:44 Customer Lifetime Value Analysis
27:46 Data Preparation
30:08 Cohort Analysis
36:53 Machine Learning Feature Engineering
37:55 Time Splitting
40:03 Feature Engineering (RFM)
41:29 Recency (R) Features
44:29 Frequency (F) Features
45:31 Monetary (M) Features
46:21 Combine RFM Features
48:51 Machine Learning CLV Analysis: Spend Amount Model
54:09 Machine Learning CLV Analysis: Spend Probability Model
57:28 Feature Importance: Spend Amount Model
59:21 Feature Importance: Spend Probability Model
1:00:52 Save Work
1:01:42 Dash App
1:04:26 How Can We Use this Information? (3 Questions)
1:06:51 Next Steps: Learning More
1:08:50 Dash App Files
1:10:00 Python Track Roadmap
1:22:12 Q&A
Комментарии