filmov
tv
Latent Space Visualisation: PCA, t-SNE, UMAP | Deep Learning Animated
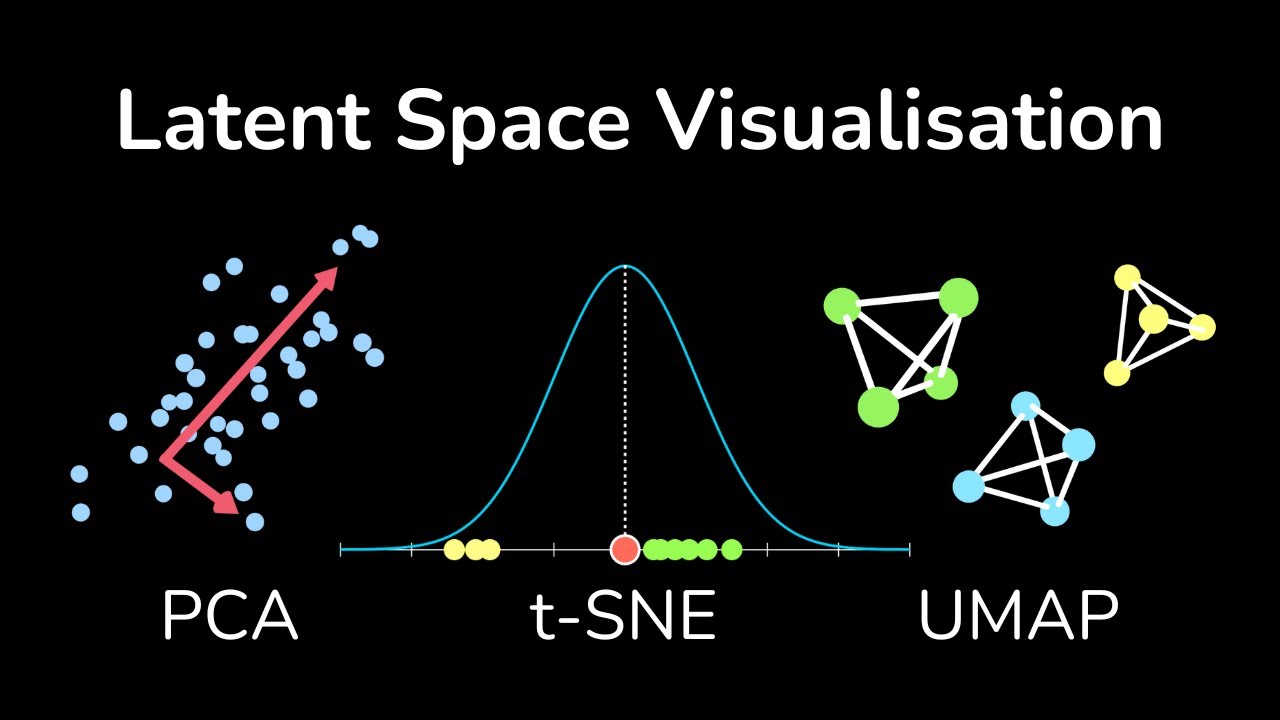
Показать описание
In this video you will learn about three very common methods for data dimensionality reduction: PCA, t-SNE and UMAP. These are especially useful when you want to visualise the latent space of an autoencoder.
If you want to learn more about these techniques, here are some key papers:
And if you want to learn about even more recent techniques such as TriMAP and PACMAP, here are the papers:
Chapters:
00:36 PCA
05:15 t-SNE
13:30 UMAP
18:02 Conclusion
If you enjoyed the content, please like, comment, and subscribe to support the channel!
#DeepLearning #PCA #ArtificialIntelligence #tsne #DataScience #LatentSpace #Manim #Tutorial #machinelearning #education #somepi
If you want to learn more about these techniques, here are some key papers:
And if you want to learn about even more recent techniques such as TriMAP and PACMAP, here are the papers:
Chapters:
00:36 PCA
05:15 t-SNE
13:30 UMAP
18:02 Conclusion
If you enjoyed the content, please like, comment, and subscribe to support the channel!
#DeepLearning #PCA #ArtificialIntelligence #tsne #DataScience #LatentSpace #Manim #Tutorial #machinelearning #education #somepi
Latent Space Visualisation: PCA, t-SNE, UMAP | Deep Learning Animated
StatQuest: t-SNE, Clearly Explained
A.I. Experiments: Visualizing High-Dimensional Space
tSNE Animation
T-SNE visualisation of novel diabetes subgroups in CNDMDS cohort: video 1 of 5
tsne
t-SNE Explanation With Visual Demo
Linear t-SNE on MNIST Dataset
Visualizing the Latent Space: This video will change how you imagine neural nets!
t-SNE per point cost during the optimization
t sne mnist10000 p8
StatQuest: Principal Component Analysis (PCA), Step-by-Step
Dimensionality reduction for feature extraction and denoising?
Latent space visualization of temporal encoder of human motion - Walking
Visualizing High Dimension Data Using UMAP Is A Piece Of Cake Now
Tensorboard t-sne to visualize local and global fraud on accounting statements
Introduction to Machine Learning - 11 - Manifold learning and t-SNE
t-SNE
UMAP Dimension Reduction, Main Ideas!!!
small NORB t-SNE visualization
Visualizing Data using t-SNE (algorithm) | AISC Foundational
Lecture 11a: PCA, SVD, and tSNE
Machine Intelligence - Lecture 4 (LDA, t-SNE)
visualizing data using t-SNE
Комментарии