filmov
tv
Advanced Methods for Hyperparameter Tuning
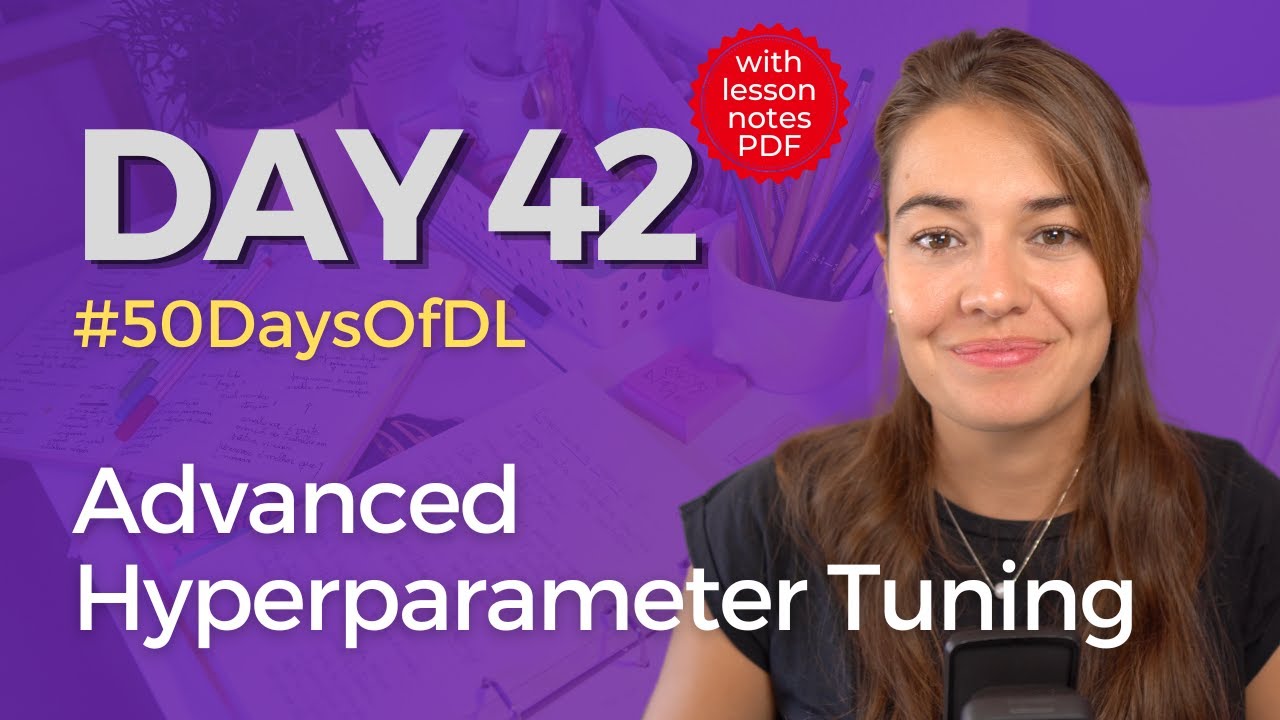
Показать описание
In this video, we learn how to tune hyperparameters of the network with advanced methods like Bayesian search, gradient-based search, and evolutionary computing.
❓To get the most out of the course, don't forget to answer the end of module questions:
👉 You can find the answers here:
RESOURCES:
COURSES:
❓To get the most out of the course, don't forget to answer the end of module questions:
👉 You can find the answers here:
RESOURCES:
COURSES:
Advanced Methods for Hyperparameter Tuning
Simple Methods for Hyperparameter Tuning
Advanced Machine Learning Techniques: Feature Engineering, Hyperparameter Tuning
Maximize accuracy of your ML model with advanced hyperparameter tuning strategies - AWS
Hyperparameter Tuning for Improving Model Accuracy #ai #datascience #learnwithav #hyperparameter
Time Series Forecasting with XGBoost - Advanced Methods
Master Optuna: Advanced Hyperparameter Tuning for Machine Learning (Step-by-Step)
XGBoost's Most Important Hyperparameters
Advanced Techniques to Accelerate Model Training & Tuning
AutoML20: A Modern Guide to Hyperparameter Optimization
Advanced Supervised Classification Techniques Hyperparameter Tuning using Google Earth Engine
Advanced Hyperparameter Optimization for Deep Learning with MLflow - Maneesh Bhide Databricks
Advanced Machine Learning with Python | Hyperparameter Tuning, Cross-Validation, and Random Forests
Deep Learning Hyperparameter Tuning in PyTorch | Making the Best Possible ML Model | Tutorial 2
Advanced Hyperparameters Tuning for AI Models
Tuning 2.0: Advanced Optimization Techniques Webinar
Best Practices for Hyperparameter Tuning with MLflowJoseph Bradley Databricks
Bayesian Hyperparameter Optimization for PyTorch (8.4)
Hyperparameter Tuning in Machine Learning
A Review of Hyperparameter Tuning Techniques for Neural Networks
Mastering Hyperparameter Tuning with Optuna: Boost Your Machine Learning Models!
Hyper-Parameter Optimization
Hyperparameter Tuning using Gridsearch - Coursera Advanced Machine Learning
1.22 Decision Tree Hyperparameter Tuning
Комментарии