filmov
tv
Mastering Hyperparameter Tuning with Optuna: Boost Your Machine Learning Models!
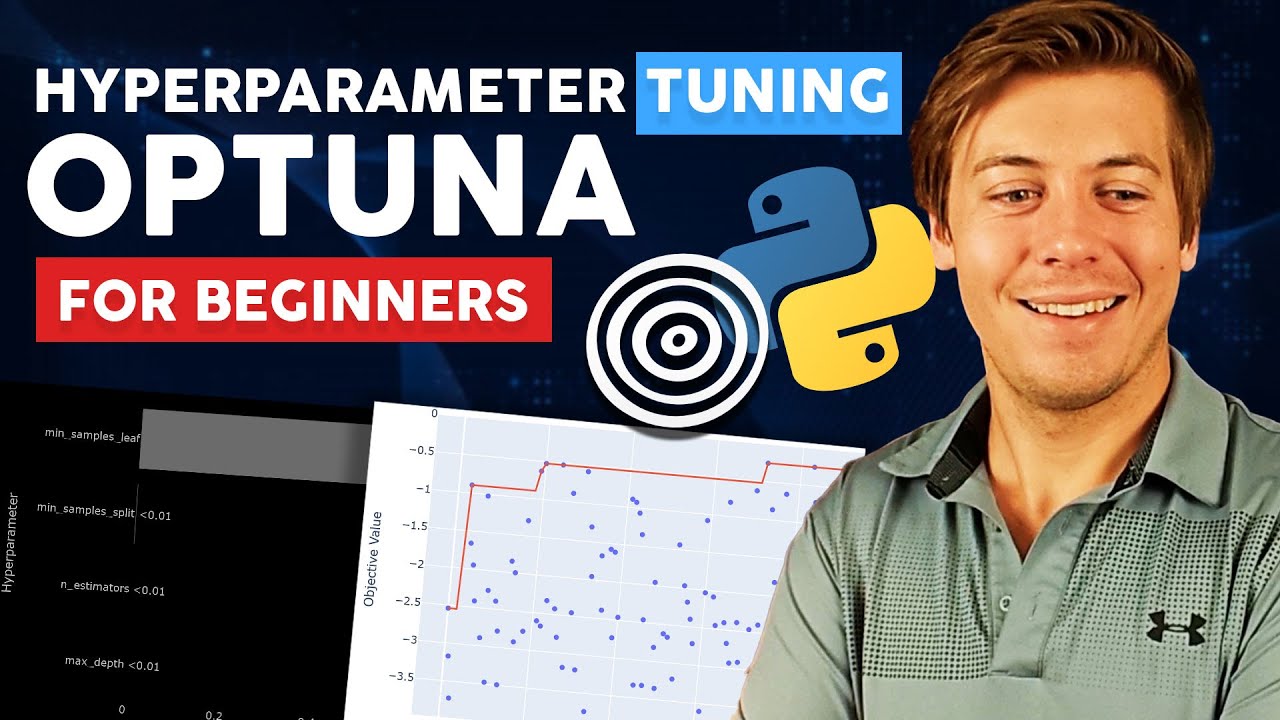
Показать описание
In this comprehensive tutorial, we delve deep into the world of hyperparameter tuning using Optuna, a powerful Python library for optimizing machine learning models. Whether you're a data scientist, machine learning enthusiast, or just looking to improve your model's performance, this video is packed with valuable insights and practical tips to help you harness the full potential of Optuna.
Interested in discussing a Data or AI project? Feel free to reach out via email or simply complete the contact form on my website.
🍿 WATCH NEXT
Vid 1:
Vid 2:
Vid 3:
MY OTHER SOCIALS:
WHO AM I?
As a full-time data analyst/scientist at a fintech company specializing in combating fraud within underwriting and risk, I've transitioned from my background in Electrical Engineering to pursue my true passion: data. In this dynamic field, I've discovered a profound interest in leveraging data analytics to address complex challenges in the financial sector.
This YouTube channel serves as both a platform for sharing knowledge and a personal journey of continuous learning. With a commitment to growth, I aim to expand my skill set by publishing 2 to 3 new videos each week, delving into various aspects of data analytics/science and Artificial Intelligence. Join me on this exciting journey as we explore the endless possibilities of data together.
*This is an affiliate program. I may receive a small portion of the final sale at no extra cost to you.
Interested in discussing a Data or AI project? Feel free to reach out via email or simply complete the contact form on my website.
🍿 WATCH NEXT
Vid 1:
Vid 2:
Vid 3:
MY OTHER SOCIALS:
WHO AM I?
As a full-time data analyst/scientist at a fintech company specializing in combating fraud within underwriting and risk, I've transitioned from my background in Electrical Engineering to pursue my true passion: data. In this dynamic field, I've discovered a profound interest in leveraging data analytics to address complex challenges in the financial sector.
This YouTube channel serves as both a platform for sharing knowledge and a personal journey of continuous learning. With a commitment to growth, I aim to expand my skill set by publishing 2 to 3 new videos each week, delving into various aspects of data analytics/science and Artificial Intelligence. Join me on this exciting journey as we explore the endless possibilities of data together.
*This is an affiliate program. I may receive a small portion of the final sale at no extra cost to you.
Комментарии