filmov
tv
Synthetic Gradients – Decoupling Layers of a Neural Nets: Anuj Gupta
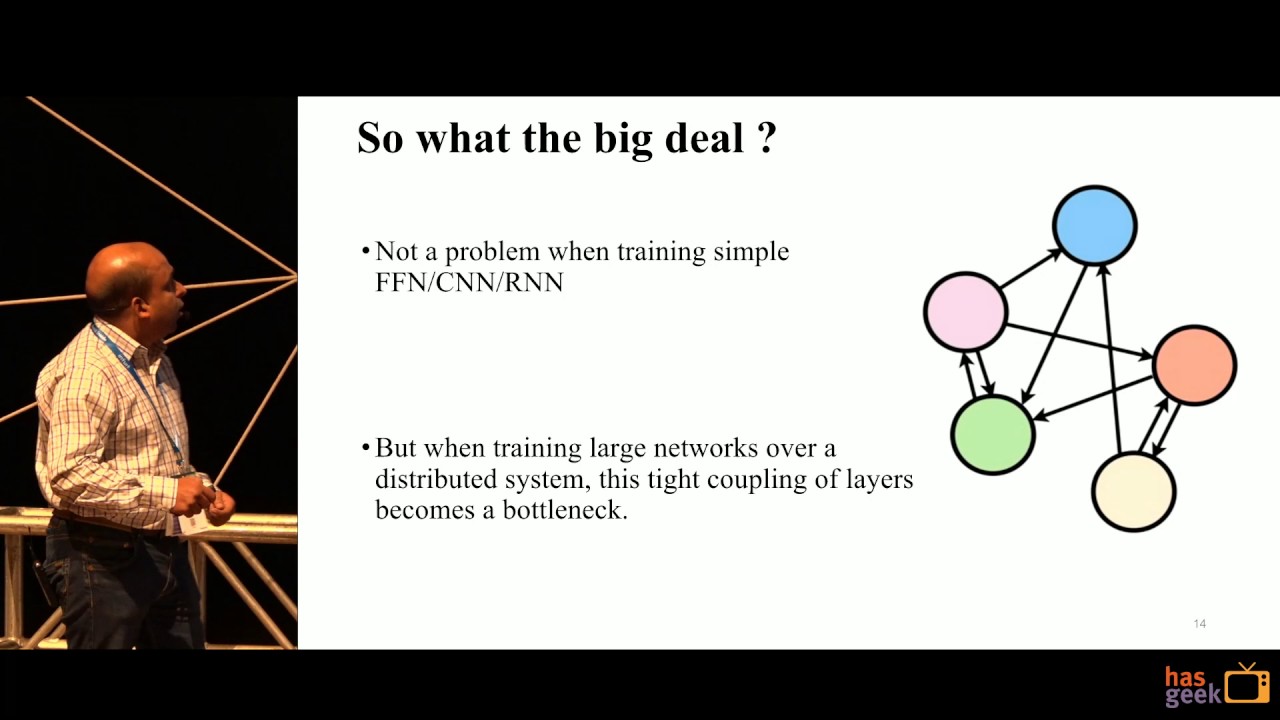
Показать описание
Once in a while comes a (crazy!) idea that can change the very fundamentals of an area. In this talk, we will see one such idea that can change how neural networks are trained.
As of now Back propagation algorithm is at the heart of training any neural net. However, the algorithm suffers from certain drawbacks which force layers of the neural net to be trained strictly in a sequential manner. In this talk we see a very powerful technique to break free from this severe limitation.
As of now Back propagation algorithm is at the heart of training any neural net. However, the algorithm suffers from certain drawbacks which force layers of the neural net to be trained strictly in a sequential manner. In this talk we see a very powerful technique to break free from this severe limitation.
Synthetic Gradients – Decoupling Layers of a Neural Nets: Anuj Gupta
Synthetic Gradients Tutorial - How to Speed Up Deep Learning Training
Synthetic Gradients Explained
Decoupled Transformers with Synthetic Gradients
Gradient descent, how neural networks learn | DL2
Training and generalization dynamics in simple deep networks
Regularization of Big Neural Networks
Transformer Network-based Optimal Decoupling Capacitor Design Method using Reinforcement Learning
Talk: Cortico-cerebellar networks as decoupled neural interfaces
Demystifying Deep Neural Nets – Rosie Campbell | Render 2017
Feature Selection with Gradient Descent on Two-layer Networks in Low-rotation Regimes
Apical dendrites as a site for gradient calculations
Implicit Reparameterization Gradients -Andriy Mnih, DeepMind
Andrew Saxe: A theory of deep learning dynamics: Insights from the linear case
Splitting Gradient Descent for Incremental Learning of Neural Architectures
Decoder-Only Transformers, ChatGPTs specific Transformer, Clearly Explained!!!
Drew Jaegle | Perceivers: Towards General-Purpose Neural Network Architectures
Decompiling Dreams: A New Approach to ARC?
CVPR 2021 Keynote -- Pieter Abbeel -- Towards a General Solution for Robotics.
The Tensors Must Flow - William Piel
Stanford Seminar - fastai: A Layered API for Deep Learning
tinyML Talks: Neural Architecture Search for Tiny Devices
TokenFormer: Rethinking Transformer Scaling with Tokenized Model Parameters (Paper Explained)
Deep Learning Review
Комментарии