filmov
tv
Decompiling Dreams: A New Approach to ARC?
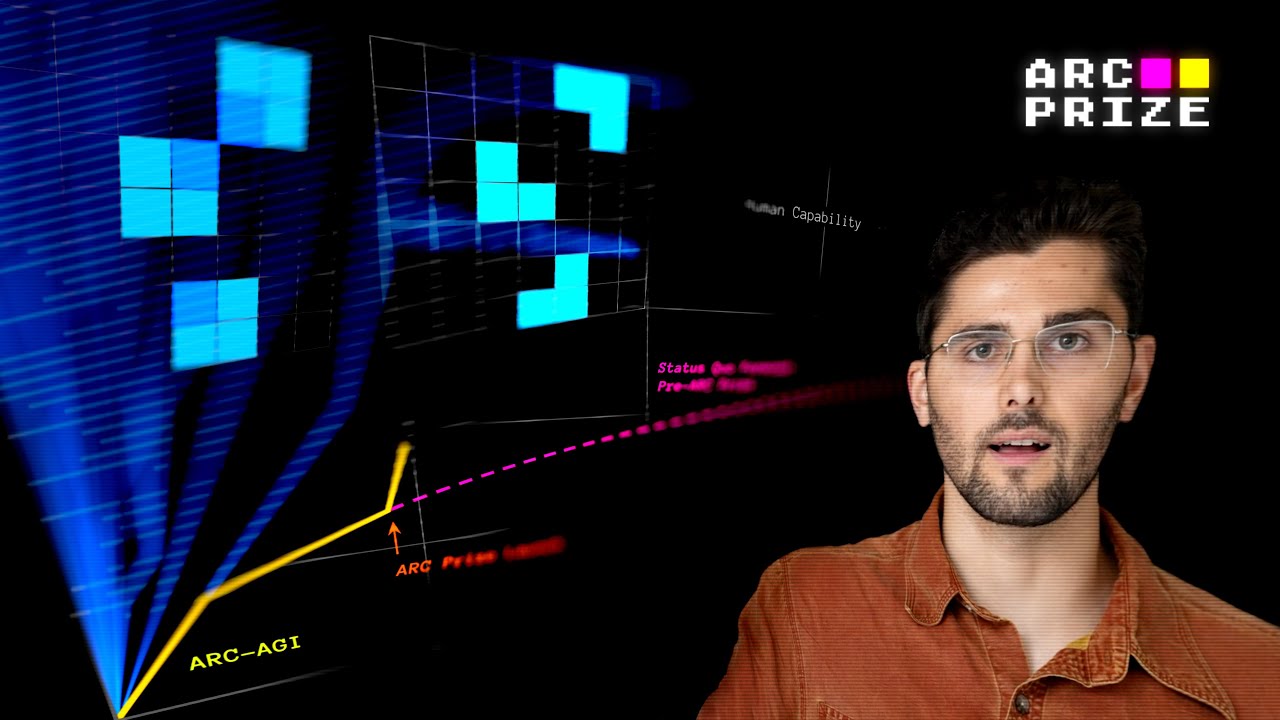
Показать описание
Alessandro Palmarini is a post-baccalaureate researcher at the Santa Fe Institute working under the supervision of Melanie Mitchell. He completed his undergraduate degree in Artificial Intelligence and Computer Science at the University of Edinburgh. Palmarini's current research focuses on developing AI systems that can efficiently acquire new skills from limited data, inspired by François Chollet's work on measuring intelligence. His work builds upon the DreamCoder program synthesis system, introducing a novel approach called "dream decompiling" to improve library learning in inductive program synthesis. Palmarini is particularly interested in addressing the Abstraction and Reasoning Corpus (ARC) challenge, aiming to create AI systems that can perform abstract reasoning tasks more efficiently than current approaches. His research explores the balance between computational efficiency and data efficiency in AI learning processes.
TOC:
1. Intelligence Measurement in AI Systems
[00:00:00] 1.1 Defining Intelligence in AI Systems
[00:02:00] 1.2 Research at Santa Fe Institute
[00:04:35] 1.3 Impact of Gaming on AI Development
[00:05:10] 1.4 Comparing AI and Human Learning Efficiency
2. Efficient Skill Acquisition in AI
[00:06:40] 2.1 Intelligence as Skill Acquisition Efficiency
[00:08:25] 2.2 Limitations of Current AI Systems in Generalization
[00:09:45] 2.3 Human vs. AI Cognitive Processes
[00:10:40] 2.4 Measuring AI Intelligence: Chollet's ARC Challenge
3. Program Synthesis and ARC Challenge
[00:12:55] 3.1 Philosophical Foundations of Program Synthesis
[00:17:14] 3.2 Introduction to Program Induction and ARC Tasks
[00:18:49] 3.3 DreamCoder: Principles and Techniques
[00:27:55] 3.4 Trade-offs in Program Synthesis Search Strategies
[00:31:52] 3.5 Neural Networks and Bayesian Program Learning
4. Advanced Program Synthesis Techniques
[00:32:30] 4.1 DreamCoder and Dream Decompiling Approach
[00:39:00] 4.2 Beta Distribution and Caching in Program Synthesis
[00:45:10] 4.3 Performance and Limitations of Dream Decompiling
[00:47:45] 4.4 Alessandro's Approach to ARC Challenge
[00:51:12] 4.5 Conclusion and Future Discussions
Refs:
1. Chollet, F. (2019). On the Measure of Intelligence. arXiv preprint arXiv:1911.01547.
Introduces a new formal definition of intelligence based on skill-acquisition efficiency. [0:01:45]
2. Mitchell, M. (2019). Artificial Intelligence: A Guide for Thinking Humans.
overview of AI for a general audience. [0:02:55]
3. OpenAI. (2019). OpenAI Five.
Developed AI agents capable of defeating professional human players in Dota 2. [0:04:40]
5. DeepMind. (2018). AlphaZero: Shedding new light on chess, shogi, and Go.
high performance in chess beyond human capabilities. [0:08:25]
6. Andoni, A., & Indyk, P. (2008). Near-optimal hashing algorithms for approximate nearest neighbor in high dimensions. Communications of the ACM, 51(1), 117-122.
locality-sensitive hash functions for approximate similarity search. [0:08:55]
7. Spelke, E. S., & Kinzler, K. D. (2007). Core knowledge. Developmental Science, 10(1), 89-96.
Introduces the concept of core knowledge systems as innate computational structures. [0:12:15]
8. Deutsch, D. (2011). The Beginning of Infinity: Explanations That Transform the World. Penguin UK.
Explores the nature of science and the role of creativity in human knowledge. [0:13:47]
9. Popper, K. (1959). The Logic of Scientific Discovery. Routledge.
falsification in scientific epistemology. [0:14:05]
10. Ellis, K., et al. (2021). DreamCoder: Bootstrapping inductive program synthesis with wake-sleep library learning. Proceedings of the 42nd ACM SIGPLAN Conference on Programming Language Design and Implementation, 835-850.
DreamCoder system for inductive program synthesis. [0:19:09]
11. Hinton, G. E., et al. (1995). The "wake-sleep" algorithm for unsupervised neural networks. Science, 268(5214), 1158-1161.
wake-sleep algorithm used in unsupervised neural networks. [0:28:25]
12. Palmarini, A. B., Lucas, C. G., & Siddharth, N. (2023). Bayesian Program Learning by Decompiling Amortized Knowledge. arXiv preprint arXiv:2306.07856.
"dream decompiling" for improving library learning in program synthesis. [0:32:25]
TOC:
1. Intelligence Measurement in AI Systems
[00:00:00] 1.1 Defining Intelligence in AI Systems
[00:02:00] 1.2 Research at Santa Fe Institute
[00:04:35] 1.3 Impact of Gaming on AI Development
[00:05:10] 1.4 Comparing AI and Human Learning Efficiency
2. Efficient Skill Acquisition in AI
[00:06:40] 2.1 Intelligence as Skill Acquisition Efficiency
[00:08:25] 2.2 Limitations of Current AI Systems in Generalization
[00:09:45] 2.3 Human vs. AI Cognitive Processes
[00:10:40] 2.4 Measuring AI Intelligence: Chollet's ARC Challenge
3. Program Synthesis and ARC Challenge
[00:12:55] 3.1 Philosophical Foundations of Program Synthesis
[00:17:14] 3.2 Introduction to Program Induction and ARC Tasks
[00:18:49] 3.3 DreamCoder: Principles and Techniques
[00:27:55] 3.4 Trade-offs in Program Synthesis Search Strategies
[00:31:52] 3.5 Neural Networks and Bayesian Program Learning
4. Advanced Program Synthesis Techniques
[00:32:30] 4.1 DreamCoder and Dream Decompiling Approach
[00:39:00] 4.2 Beta Distribution and Caching in Program Synthesis
[00:45:10] 4.3 Performance and Limitations of Dream Decompiling
[00:47:45] 4.4 Alessandro's Approach to ARC Challenge
[00:51:12] 4.5 Conclusion and Future Discussions
Refs:
1. Chollet, F. (2019). On the Measure of Intelligence. arXiv preprint arXiv:1911.01547.
Introduces a new formal definition of intelligence based on skill-acquisition efficiency. [0:01:45]
2. Mitchell, M. (2019). Artificial Intelligence: A Guide for Thinking Humans.
overview of AI for a general audience. [0:02:55]
3. OpenAI. (2019). OpenAI Five.
Developed AI agents capable of defeating professional human players in Dota 2. [0:04:40]
5. DeepMind. (2018). AlphaZero: Shedding new light on chess, shogi, and Go.
high performance in chess beyond human capabilities. [0:08:25]
6. Andoni, A., & Indyk, P. (2008). Near-optimal hashing algorithms for approximate nearest neighbor in high dimensions. Communications of the ACM, 51(1), 117-122.
locality-sensitive hash functions for approximate similarity search. [0:08:55]
7. Spelke, E. S., & Kinzler, K. D. (2007). Core knowledge. Developmental Science, 10(1), 89-96.
Introduces the concept of core knowledge systems as innate computational structures. [0:12:15]
8. Deutsch, D. (2011). The Beginning of Infinity: Explanations That Transform the World. Penguin UK.
Explores the nature of science and the role of creativity in human knowledge. [0:13:47]
9. Popper, K. (1959). The Logic of Scientific Discovery. Routledge.
falsification in scientific epistemology. [0:14:05]
10. Ellis, K., et al. (2021). DreamCoder: Bootstrapping inductive program synthesis with wake-sleep library learning. Proceedings of the 42nd ACM SIGPLAN Conference on Programming Language Design and Implementation, 835-850.
DreamCoder system for inductive program synthesis. [0:19:09]
11. Hinton, G. E., et al. (1995). The "wake-sleep" algorithm for unsupervised neural networks. Science, 268(5214), 1158-1161.
wake-sleep algorithm used in unsupervised neural networks. [0:28:25]
12. Palmarini, A. B., Lucas, C. G., & Siddharth, N. (2023). Bayesian Program Learning by Decompiling Amortized Knowledge. arXiv preprint arXiv:2306.07856.
"dream decompiling" for improving library learning in program synthesis. [0:32:25]
Комментарии